ATR-FTIR spectroscopy and Machine learning for sustainable wood sourcing and species Identification: Applications to wood forensics
Microchemical Journal(2024)
摘要
Wood forensics, a vital tool in addressing global concerns surrounding illegal logging and the authenticity of wood products, plays a pivotal role in promoting sustainable forestry practices. The integration of Attenuated Total Reflectance Fourier-Transform Infrared (ATR-FTIR) spectroscopy and machine learning presents a potent approach to streamline identification processes and enhance responsible wood sourcing. ATR-FTIR facilitates the detailed characterization of wood components by analyzing spectral data, revealing unique chemical signatures associated with different wood species. This study focuses on Eucalyptus, Dalbergia, and Populus wood species in India, revealing common peaks related to cellulose, flavones, hemicellulose, and lignin constituents. Isolation Forest (iForest) identifies outliers, subsequently removed for supervised modelling, while Principal Component Analysis (PCA) reduces dimensionality effectively. Tree-based supervised machine learning algorithms, including Decision Tree, Extra Tree, Random Forest, and CatBoost classifiers, enhances the accuracy of wood species discrimination by deciphering complex patterns within ATR-FTIR spectra. Further, evaluation through various performance metrics supports the comparative analysis. The outcomes of this study hold significant value for law enforcement agencies in combating illegal logging and timber trade, and potential applications in quality control for timber industries. This research substantiates its real-world applicability, marking a noteworthy advancement in the evolving field of wood forensics.
更多查看译文
关键词
Wood Forensics,ATR-FTIR,Supervised Modelling,Tree-based classifiers,PyCaret
AI 理解论文
溯源树
样例
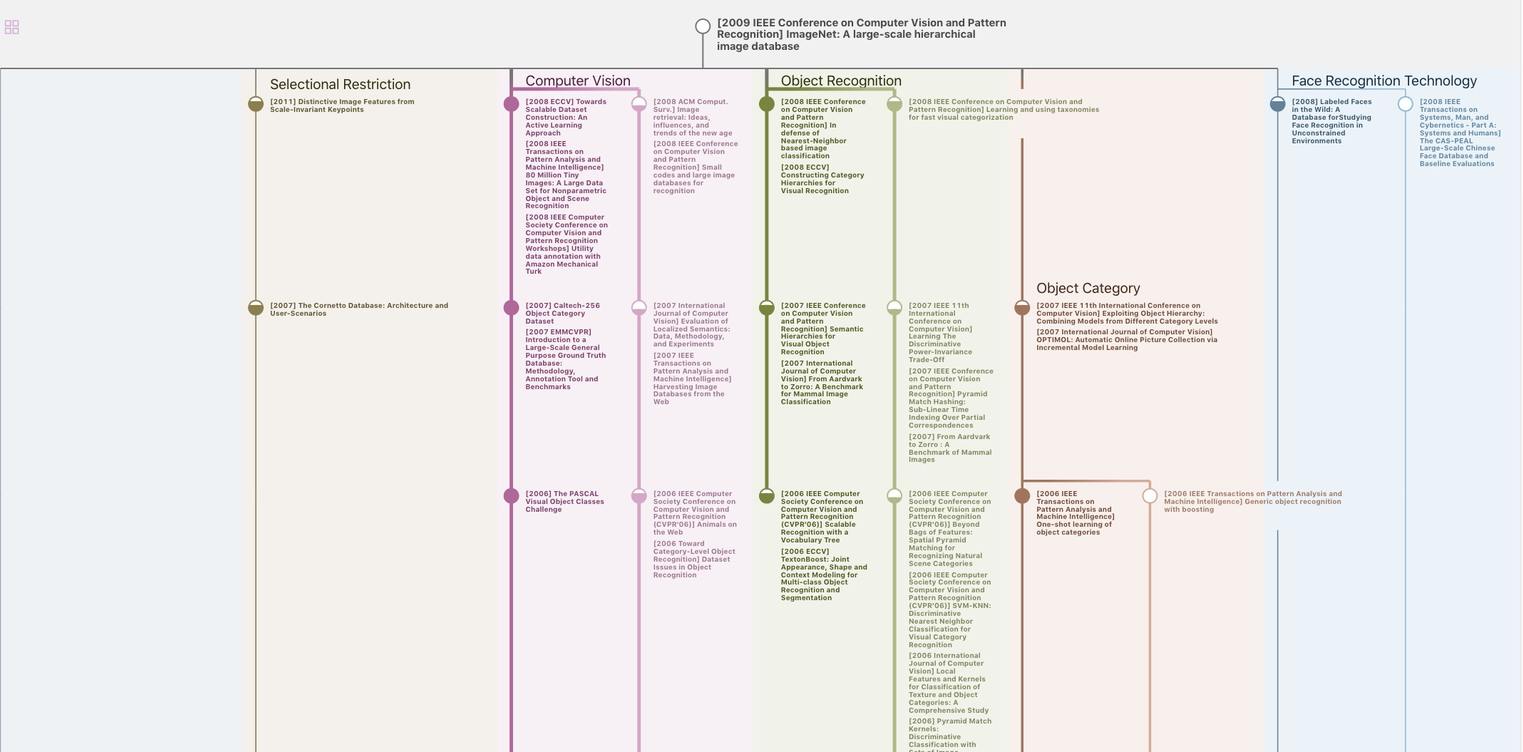
生成溯源树,研究论文发展脉络
Chat Paper
正在生成论文摘要