Improved Convolutional Neural Network-Assisted Laser-Induced Breakdown Spectroscopy for Identification of Soil Contamination Types
Spectrochimica acta Part B, Atomic spectroscopy(2024)
摘要
Identification of soil contamination types is of great scientific significance for soil remediation and environmental pollution control. However, traditional identification methods for soil determination are time-consuming, laborious, and complicated. Here, we proposed an accurate method for identifying soil contamination types based on laser-induced breakdown spectroscopy (LIBS) and an improved convolutional neural network (CNN) model. The spectral feature extraction-based multiple attention residual network (SFEMARNet) model was constructed to extract detailed features by spectral feature extraction (SFE) modules, and highlight useful features by multiple attention residual (MAR) modules in LIBS spectral data. In addition, deep learning models and machine learning models were used to identify the data. The results showed that the SFEMARNet model achieved an accuracy of 98.75% on the test set. The recall, precision, and F1-score of the models reached 98.78%, 98.75%, and 98.76%, respectively, which were significantly better than the three deep learning models and of four machine learning models. It seems that the SFEMARNet model combined with LIBS technology may be a potential method for the accurate identification of soil contamination types.
更多查看译文
关键词
Soil contamination types,Laser -induced breakdown spectroscopy,Convolutional neural network,Identification
AI 理解论文
溯源树
样例
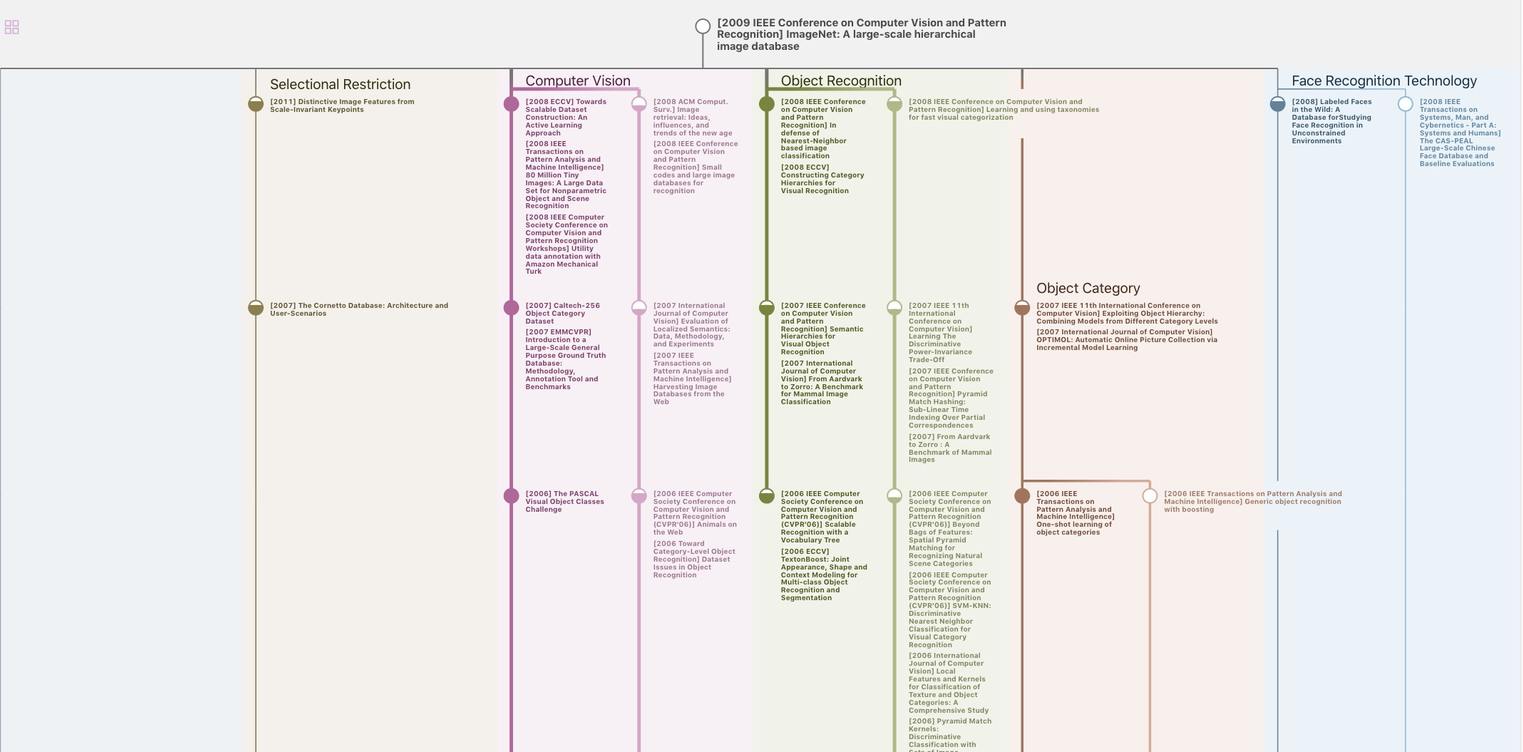
生成溯源树,研究论文发展脉络
Chat Paper
正在生成论文摘要