Synergizing multiple machine learning techniques and remote sensing for advanced landslide susceptibility assessment: a case study in the Three Gorges Reservoir Area
Environmental Earth Sciences(2024)
摘要
This study conducts an in-depth exploration of the efficacy of deep learning and ensemble learning techniques for slope-unit-based landslide susceptibility prediction within the context of the Three Gorges Reservoir area in China, with a specific focus on Wanzhou District. Leveraging a dataset comprising twelve distinct landslide factors and 1909 Slope Units, the research evaluates three deep learning models (Long Short-Term Memory, Recurrent Neural Network, and Gated Recurrent Unit) as well as three ensemble learning models (LightGBM (LGBM), Extra Trees, and Random Forest) using five performance metrics. Central to this endeavor is the adept utilization of remote sensing technology, including Landsat 8 OLI images, Digital Elevation Model (DEM) data, and Google Earth Pro images. The Landsat 8 OLI images offer a panoramic view of the study area, capturing essential landscape features and variations. The DEM data, providing detailed elevation information, empowers the analysis of terrain morphology crucial for landslide susceptibility assessment. The findings conclusively showcase that ensemble learning models harnessed in this study, augmented by the integration of diverse remote sensing data, exhibit exceptional predictive capabilities in accurately anticipating landslide susceptibility. These models outperform their deep learning model counterparts, attributing their success to the multi-faceted insights derived from the synergy between remote sensing imagery and advanced machine learning algorithms. The ensemble models’ enhanced performance metrics, such as F1-score, recall, precision, and area under the curve (AUC) values, underscore their potential utility in real-world landslide prediction scenarios. Especially noteworthy among the ensemble models is LGBM, which emerges as the most promising candidate with the highest F1-score (0.561) and Recall (0.524), indicating that the LGBM model possesses a more robust predictive capability for landslide samples. In-depth interpretability analysis using SHapley Additive exPlanations (SHAP) values and Partial Dependence Plots (PDP) assessments delves into the mechanics of LGBM’s predictive prowess. This analysis, reliant on remote sensing data, provides clarity into the contributions of various evaluation factors, emphasizing the roles of attributes such as proximity to the river, rainfall, and elevation. The correlation patterns revealed between these factors and landslide susceptibility add layers of understanding, while the intricate interplay of distance to the river unveils the complex interactions between geological and climatic variables.
更多查看译文
关键词
Slope-unit-based,Landslide susceptibility,Three Gorges Reservoir,Interpretability
AI 理解论文
溯源树
样例
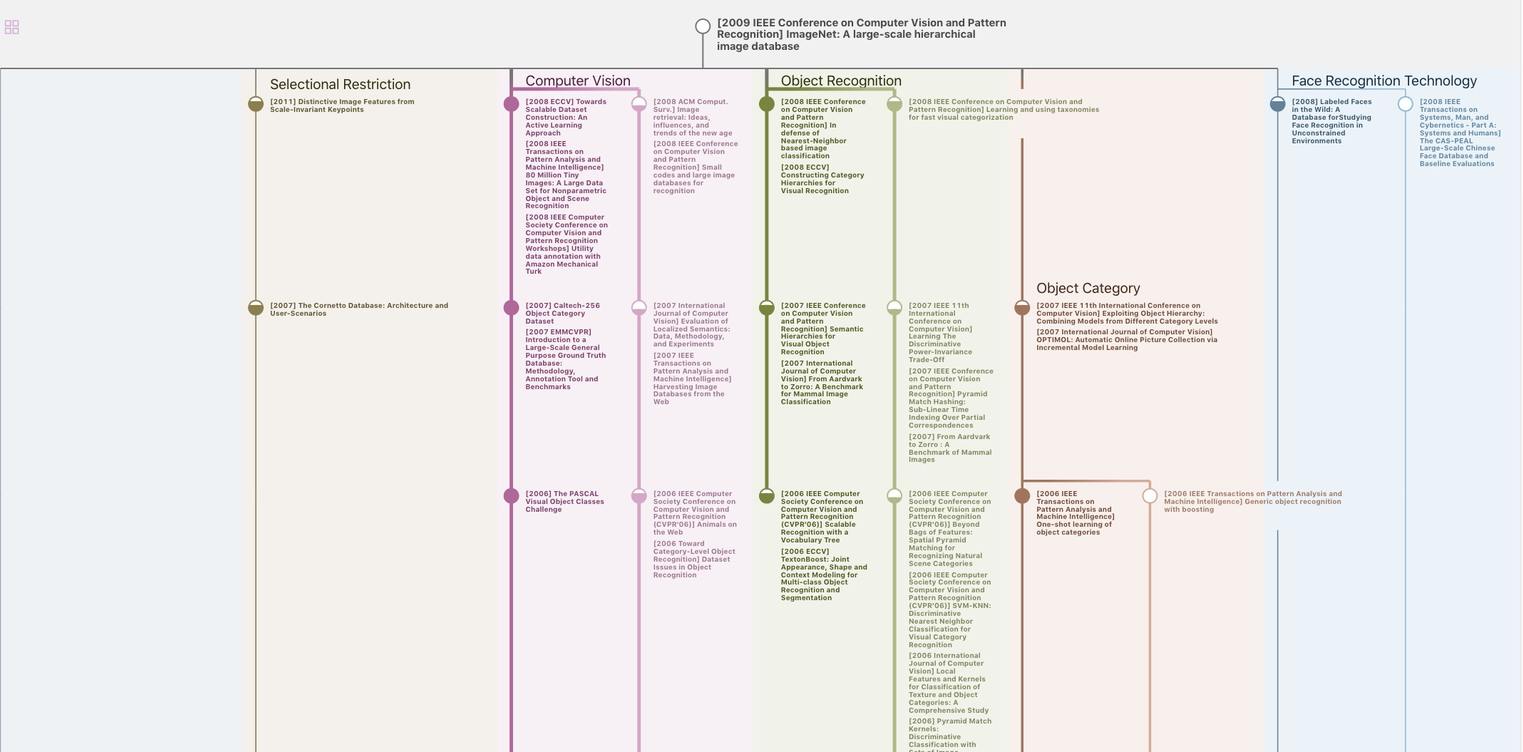
生成溯源树,研究论文发展脉络
Chat Paper
正在生成论文摘要