Large-Margin Extreme Learning Machines With Hybrid Features for Wafer Map Defect Recognition.
IEEE Trans. Instrum. Meas.(2024)
摘要
The critical information regarding the semiconductor manufacturing can be provided based on the wafer map defect patterns. Automatic wafer map defect identification with machine learning methods has recently received increasing attention. The wafer map defect recognition problem can be tackled in two steps. Firstly, hybrid features including Radon-based features, density-based features, and autoencoder-based features are extracted. Then two novel large-margin extreme learning machine (LM-ELM) methods are proposed for wafer map defect recognition. Compared with classical extreme learning machines, these approaches take the large absolute/relative margin constraint on the regression target vector into consideration, resulting in two novel ELM variants referred to as large-absolute-margin extreme learning machine (LAM-ELM) and large-relative-margin extreme learning machine (LRM-ELM). The convexity of the proposed methods is proved which can guarantee the unique optimal solution. Furthermore, the algorithms to solve both LAM-ELM and LRM-ELM are provided and convergence analysis of the presented algorithms are conducted. The experiments show that the proposed methods performs better than other competing approaches with an accuracy of 98.26% on the WM-811K dataset.
更多查看译文
关键词
Feature extraction,wafer map defect recognition,large absolute margin,large relative margin,extreme learning machine (ELM)
AI 理解论文
溯源树
样例
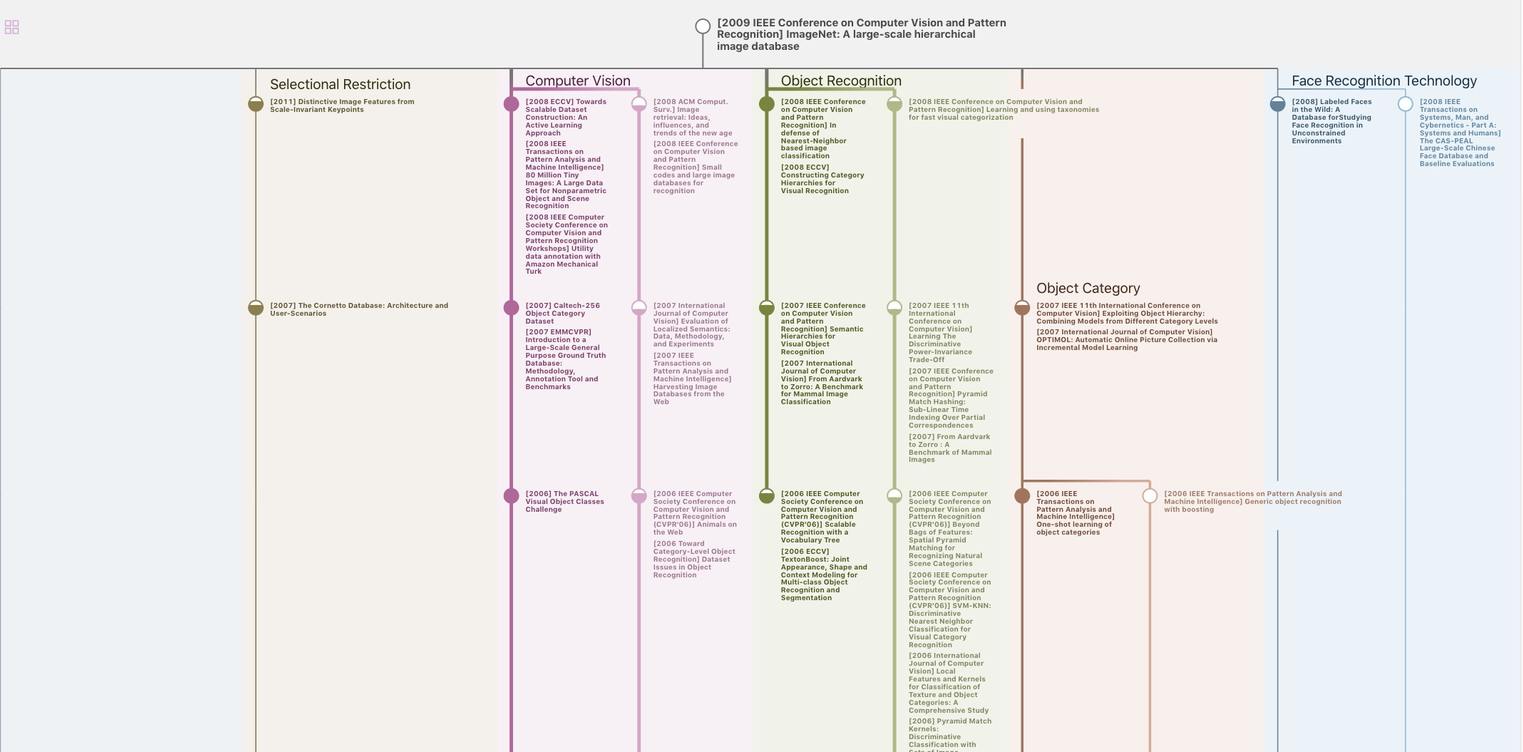
生成溯源树,研究论文发展脉络
Chat Paper
正在生成论文摘要