Energy-Efficient Brain Floating Point Convolutional Neural Network Using Memristors
IEEE TRANSACTIONS ON ELECTRON DEVICES(2024)
摘要
In this article, a memristor-based convolutional neural network (CNN) is implemented to achieve both brain floating point (BF16) processing accuracy and high energy efficiency for cloud artificial intelligence (AI) acceleration for the first time. A low-cost in-memory floating-point (FP) computing arithmetic is developed with an approximate computing technique, which sufficiently considers the requirement of both efficiency and accuracy in FP-CNN applications. For further optimization, we investigate the impact of nonideal effects at the device and array level (including the device variation, line resistance, array parasitic capacitance, etc.) on analog computing. Based on these, a bit-weight slicing technique is employed for highly efficient and accurate FP computing within the memristor crossbar array. Meanwhile, an in-memory convolutional operating method is proposed to further reduce the hardware overhead in deploying large-scale CNNs for complex datasets. By combining the above strategies, we evaluate the performance of memristor-implemented BF16-CNNs using the VGG-16 network on the CIFAR-10 dataset. An 85.47% classification accuracy with 1.987 TFLOPS/W energy efficiency and 20.9895 GFLOPS/mm(2) area efficiency is obtained.
更多查看译文
关键词
Memristors,Arrays,Artificial intelligence,Convolutional neural networks,Arithmetic,Energy efficiency,Computational modeling,Convolutional neural network (CNN),floating-point (FP) computing,in-memory computing (IMC),memristor
AI 理解论文
溯源树
样例
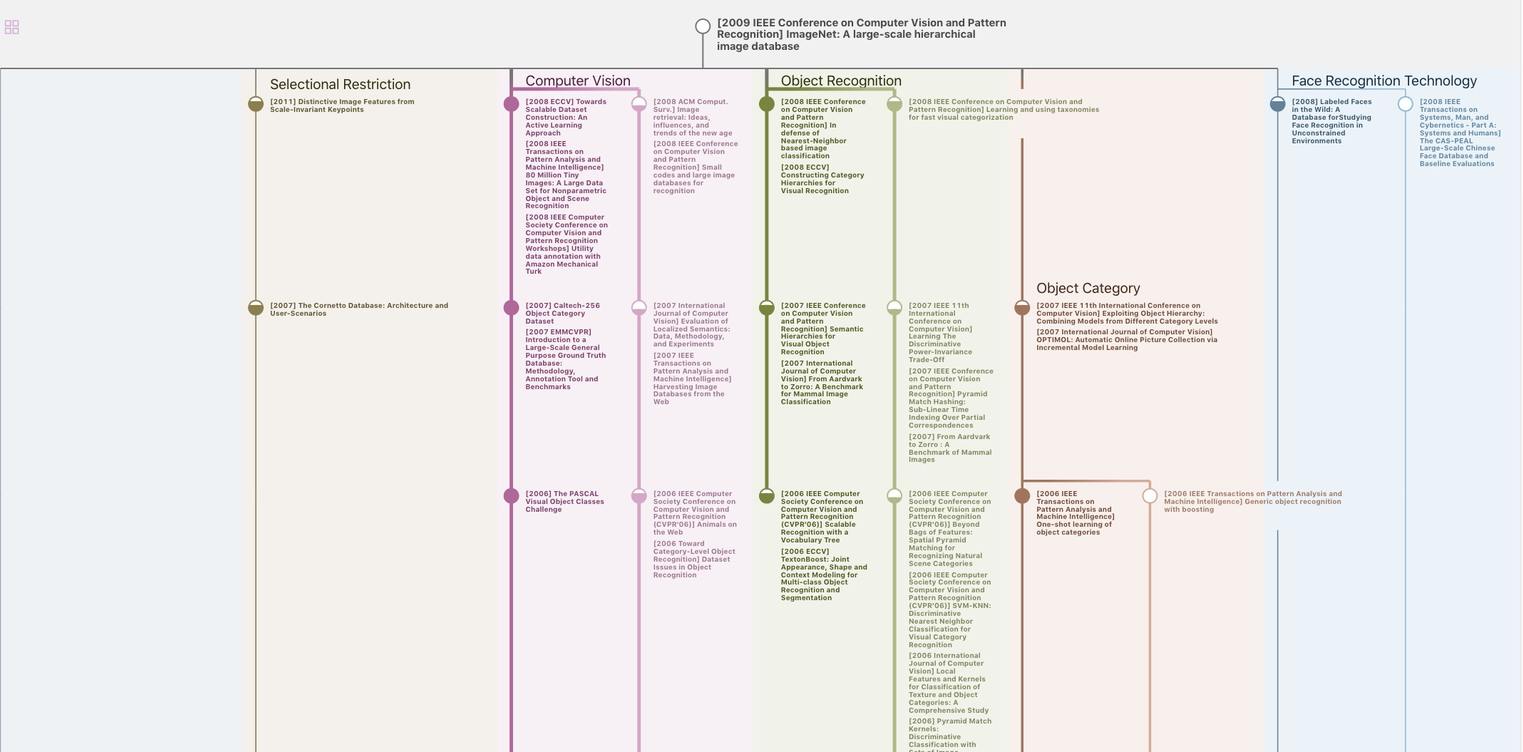
生成溯源树,研究论文发展脉络
Chat Paper
正在生成论文摘要