Multi-task Graph Adaptive Learning for Multivariate Electricity Price Short-Term Forecasting in Australia's National Electricity Market
IEEE Transactions on Power Systems(2024)
摘要
Accurate electricity price short-term forecasting plays an essential role in the digitization of the electricity market. However, due to the expansion of renewable energy resources and the development of electricity demands, electricity prices are increasingly volatile and difficult to predict, posing a significant threat to the security of daily electricity market operations. The uncertainty of the supply-demand balance, the spatiotemporal correlation of the electricity market are two major obstacles to making the forecasting precisely. In this paper, a multi-task learning model (MGAAL) utilizes a graph attention mechanism and incorporates an auxiliary task focused on predicting abnormal price spikes, enhancing generalization and reducing overfitting risk. Specifically, MGAAL employs attention-based Graph Neural Networks to enhance price forecasting by capturing temporal and spatial power flow dynamics. In addition, MGAAL can also adaptively assign task weights based on homoscedasticity uncertainty and gradient normalization of the tasks. Finally, our experiments, conducted using data from Australia's National Electricity Market (NEM), demonstrate the effectiveness of MGAAL, surpassing current state-of-the-art methods.
更多查看译文
关键词
Electricity price forecasting,Price spiking prediction,Anomaly event detection,Multi-task learning,Graph neural networks
AI 理解论文
溯源树
样例
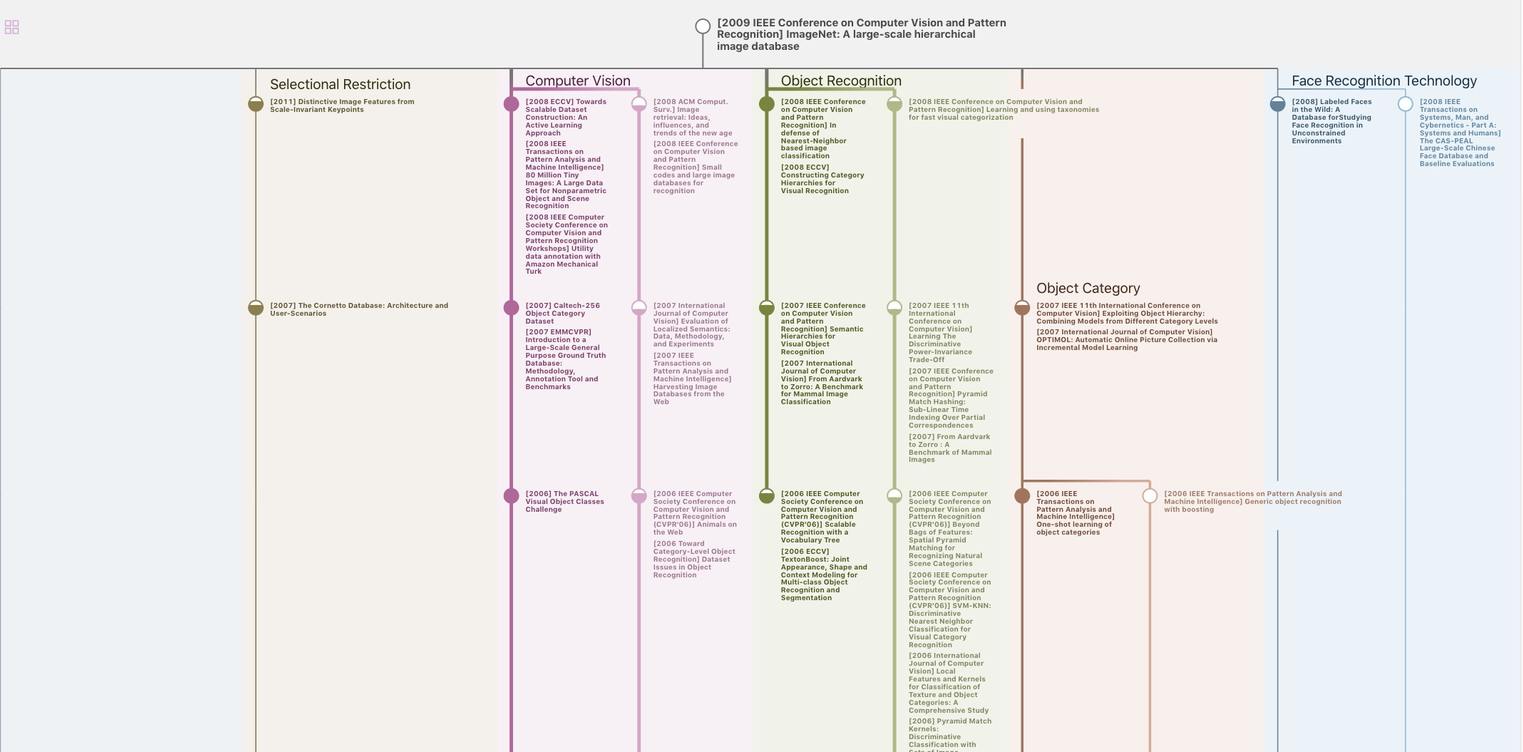
生成溯源树,研究论文发展脉络
Chat Paper
正在生成论文摘要