A High Resolution and Configurable 1T1R1C ReRAM Macro for Medical Semantic Segmentation.
IEICE electronics express(2024)
摘要
Medical industry increasingly using convolutional neural networks (CNNs) for image processing. Nowadays, computing facilities based on Von Neumann architecture aredevoted to accelarate CNNs, yet rapidly hitting a bottlenneck in performance and energy efficiency. The computing-in-memory (CIM) architecture based on random-access memory (ReRAM) emerged as a method to overcome the issue. This work proposes a charge-domain one-transistor-one-resistor-onecapacitor (1T1R1C) CIM macro using energy-efficient charge calculation and capacitive coupling for CNNs acceleration in medical semantic segmentation. The multiplication-and-accumulation (MAC) is realized by charge distribution with a cell and capacitive coupling across different cells on a plate line. The configurable output resolution is achieved by on-chip ReRAM-based charge integral, which is energy efficient and flexible to change the output resolution. By evaluation in the 180nm technology, the proposed macro with a 64x64 array achieves a peak energy efficiency of 142.2 GOPS/W, similar to 1.3X higher than previous work. The inference dice coefficient of UNet reaches 89.7%.
更多查看译文
关键词
ReRAM,computing-in-memory,medical semantic segmentation,configurable
AI 理解论文
溯源树
样例
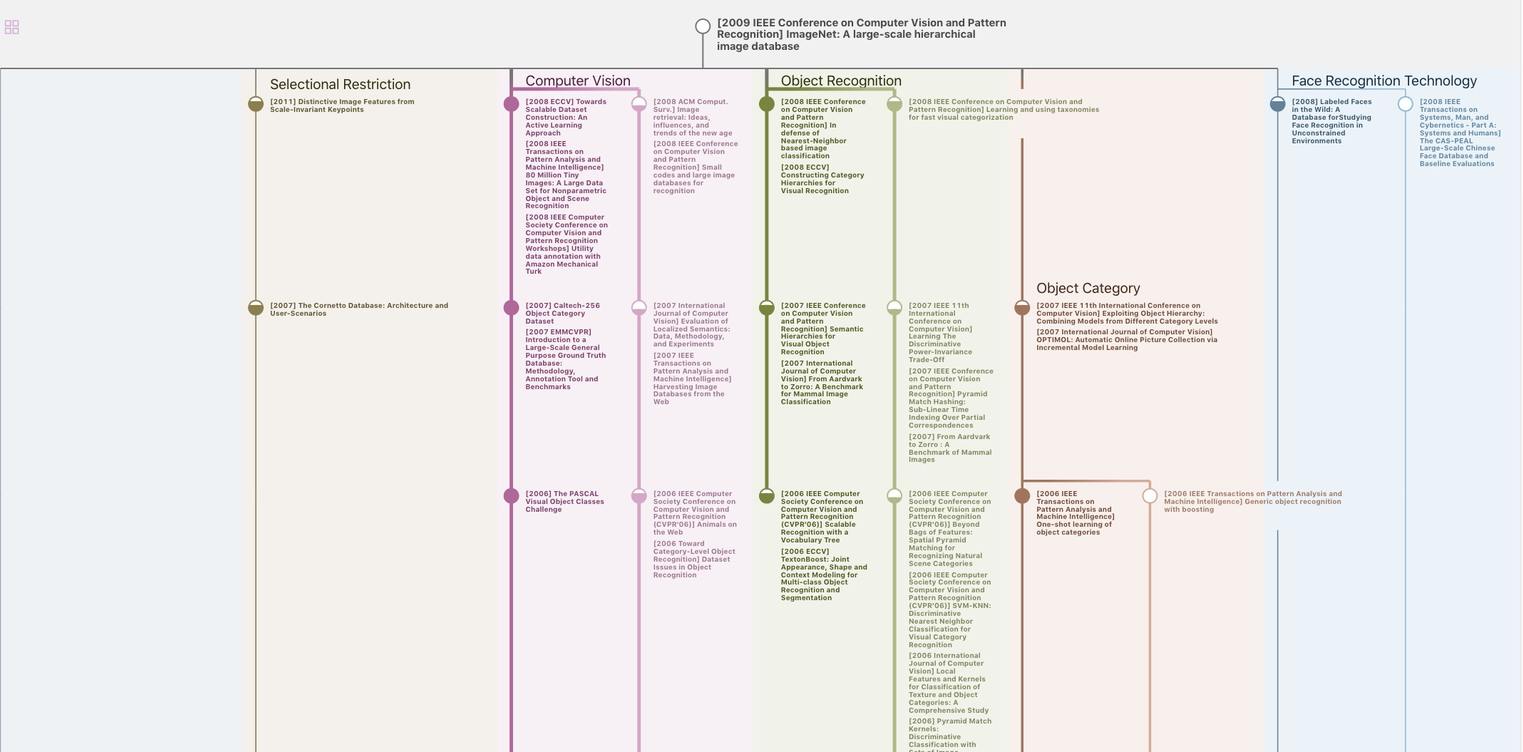
生成溯源树,研究论文发展脉络
Chat Paper
正在生成论文摘要