Multifidelity Gaussian Processes for Predicting Shear Viscosity over Wide Ranges of Liquid State Points Based on Molecular Dynamics Simulations
INDUSTRIAL & ENGINEERING CHEMISTRY RESEARCH(2024)
摘要
A linear multifidelity model based on Gaussian process (GP) regression is proposed that uses shear viscosities from a few molecular dynamics simulations as well as a few experimental shear viscosities to enable a high-quality prediction of this transport property over a large range of thermodynamic state points. Transport properties, such as viscosity, determined from molecular simulations are sensitive to the underlying force field, which is often parametrized with emphasis on vapor-liquid coexisting properties, so that mean absolute deviations of 20% or more are frequently observed. A linear multifidelity model based on GP regression allows for compensating for such quantitative deviations based on very limited experimental data. The requirement for the molecular simulation is to describe the univariate relationship between the dimensionless shear viscosity and the reduced residual entropy qualitatively over a wide range of reduced residual entropy. This allows for a high-fidelity prediction of the shear viscosity for regions not covered by the experimental training data. The reliability of this new approach is showcased for 14 substances with more than 6000 data points, whereby only about 5 data points per species were used to train the multifidelity model. The few data points for training (selected in a narrow range of temperatures and ambient pressure, if possible) were used to mimic situations where substances are characterized by few experimental measurements, only.
更多查看译文
AI 理解论文
溯源树
样例
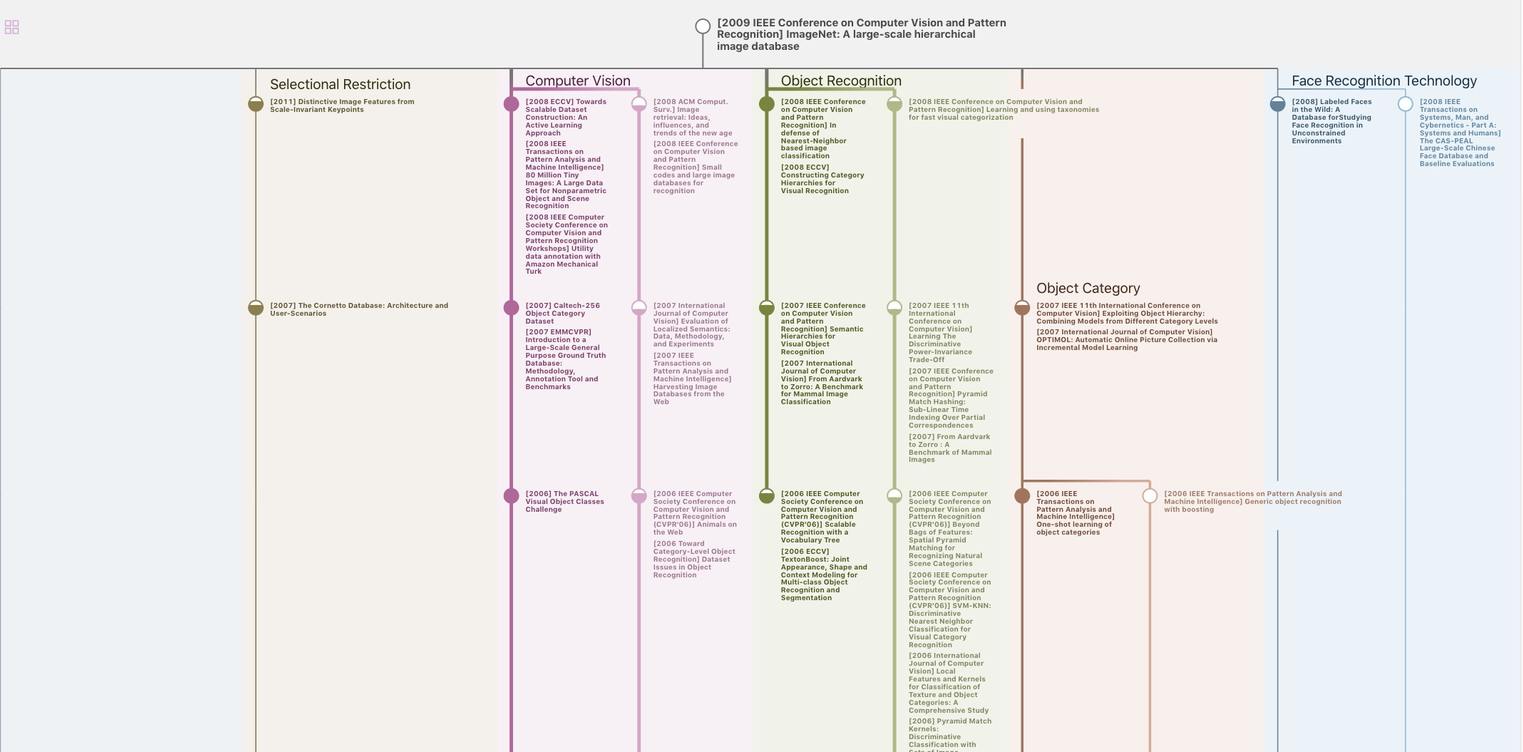
生成溯源树,研究论文发展脉络
Chat Paper
正在生成论文摘要