Relaxed multi-view discriminant analysis
ENGINEERING APPLICATIONS OF ARTIFICIAL INTELLIGENCE(2024)
摘要
Consistency and complementarity are two important principles in multiview feature extraction. However, most current multiview feature extraction methods only explore the former but neglect the latter. To alleviate this limitation, in this article we propose a relaxed multiview discriminant analysis (RMDA) model. Firstly, a relaxed loss function is formulated to make the projection matrices have more degrees of freedom. Then two scatter matrices are utilized to preserve cross-view between-class and within-class discriminative information. The proposed RMDA explores the complementarity of multiple views while maintaining the consistency across different views. To solve the RMDA problem efficiently, an iteration strategy is proposed. Theoretical analysis demonstrates the effectiveness and quadratic convergence rate of the RMDA algorithm. To further deal with nonlinearities present in the data, a relaxed kernel multiview discriminant analysis (RKMDA) is put forward too. Several corroborating numerical tests using artificial dataset and real datasets are provided to showcase the merits of the RMDA and RKMDA relative to several competing methods.
更多查看译文
关键词
Multi-view,Feature extraction,Relaxed loss function,Discriminant analysis
AI 理解论文
溯源树
样例
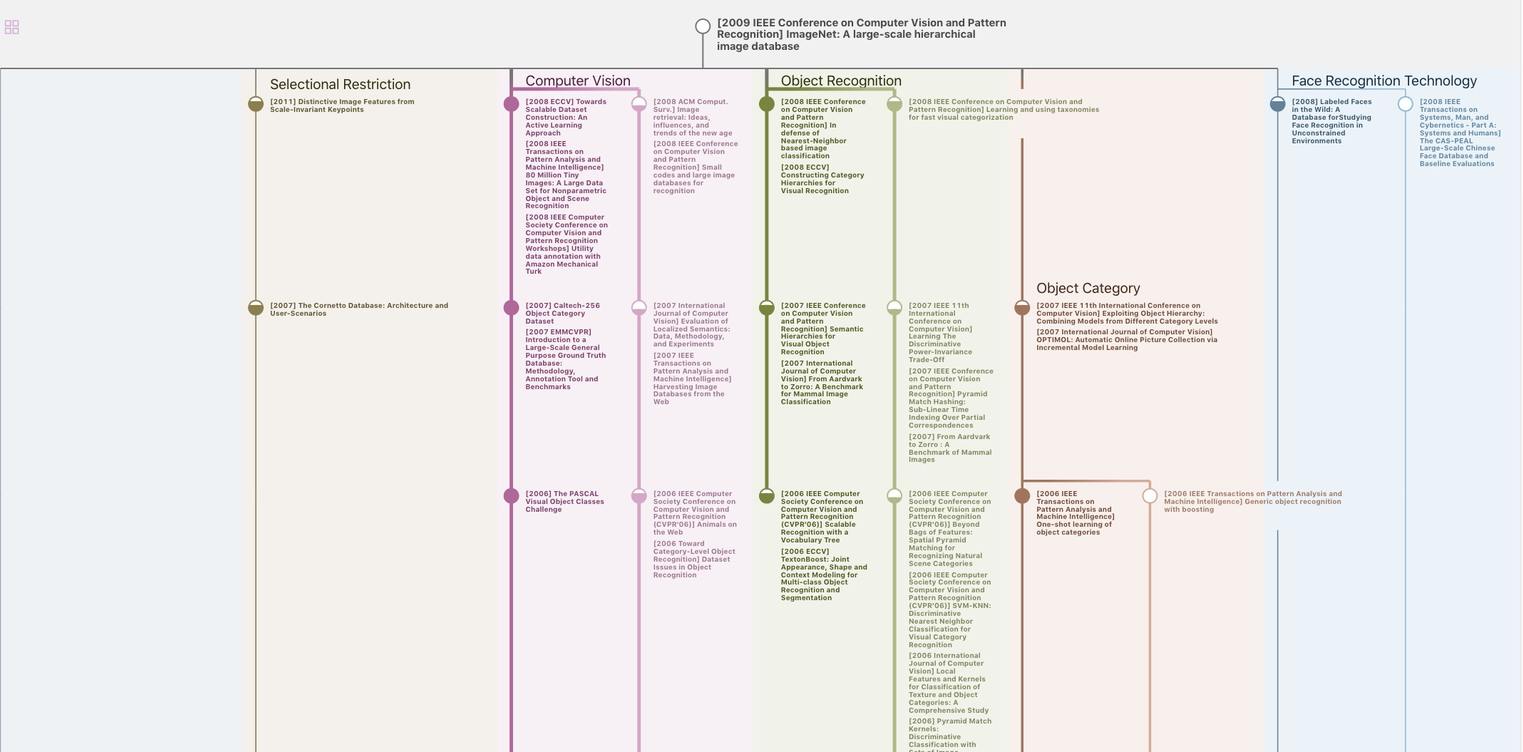
生成溯源树,研究论文发展脉络
Chat Paper
正在生成论文摘要