Avoiding Mistakes in Bivariate Linear Regression and Correlation Analysis, in Rigorous Research
ACTA POLYTECHNICA HUNGARICA(2024)
摘要
Data science and artificial intelligence are emergently, very fast -evolving fields, being applied to a large diversity of real -life problem -solving. In this context, some methods are applied without verifying assumptions that must be met, for the correct applicability and the necessary model fit. Such mistakes could lead to misinterpretations of the results. One of the application domains, that is very affected in this sense, is healthcare, where misinterpretations could have dangerous effects on human health. Based on an indepth study of the scientific literature, it was identified that bivariate linear regression (BLR) even is considered simple, is one of the methods that sometimes leads to confusion in application. With this in mind, this paper proposes in an algorithmic form of a methodology that consists of assumptions, that must be passed by the BLR, so that the applicability is correct and should pass the required threshold model fit. Also, presented in algorithmic form is the decision for the correct calculus of the bivariate linear correlation coefficient (BCC). There are other considerations, like the necessary sample sizes for the two variables in the case of BCC and BLR. The proposed methodology, herein, will be useful for researchers, since BLR is frequently applied in research in diverse domains, like industry and healthcare, individually or combined with methods of data science and artificial intelligence.
更多查看译文
关键词
data science,linear regression,model fit,prediction,artificial intelligence,mathematical modeling,goodness-of-fit,mistakes encountered in clinical research,correlation coefficient,data misinterpretation
AI 理解论文
溯源树
样例
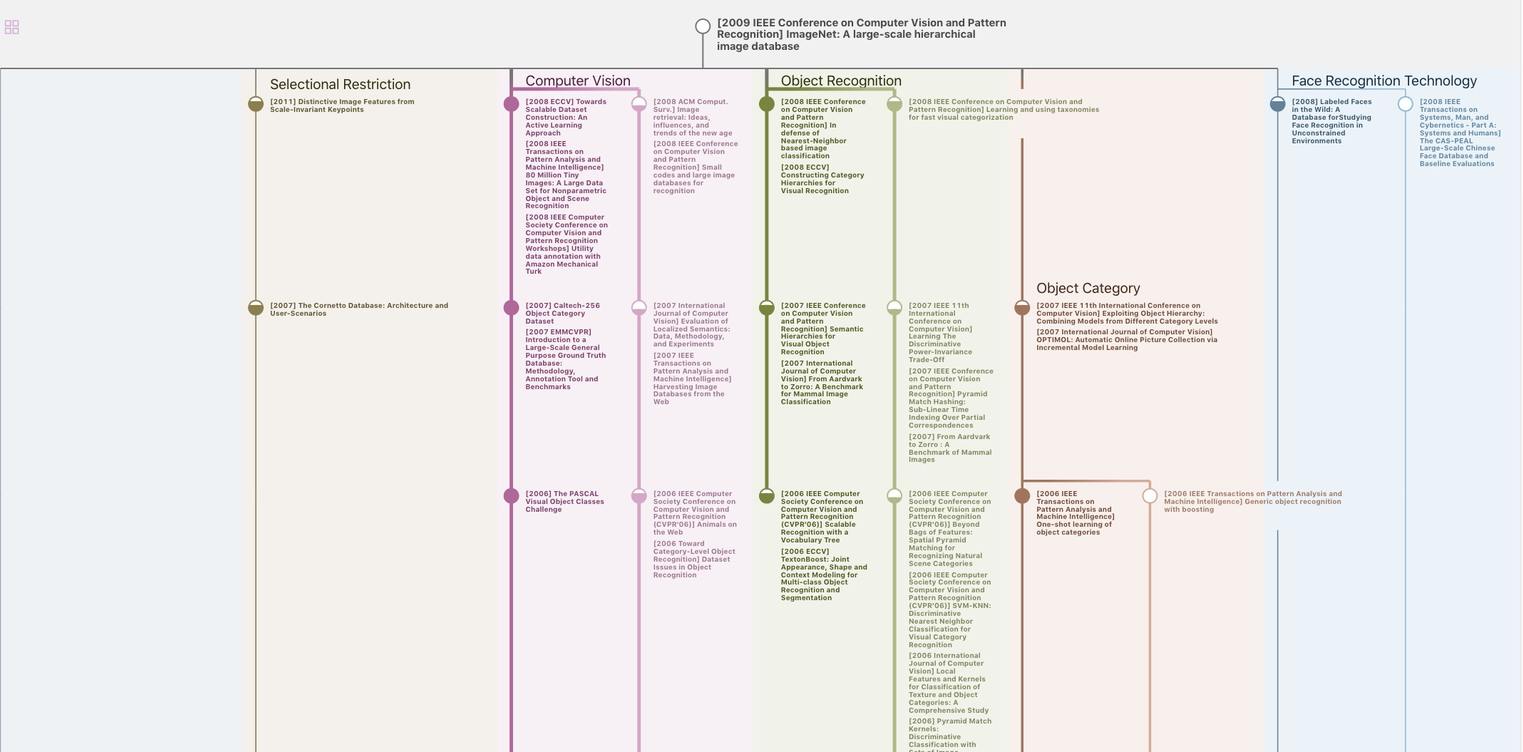
生成溯源树,研究论文发展脉络
Chat Paper
正在生成论文摘要