Bayesian non-negative matrix factorization with Student's t-distribution for outlier removal and data clustering
ENGINEERING APPLICATIONS OF ARTIFICIAL INTELLIGENCE(2024)
摘要
Non -negative Matrix Factorization (NMF) is an effective way to solve the redundancy of non -negative highdimensional data. Most of the traditional probability -based NMF methods use Gaussian distribution to model the differences between the matrices before and after decomposition. However, the Gaussian distribution is strongly affected by outliers, and it may not fit all datasets accurately when there are no outliers in the data. In this article, we propose a novel Bayesian NMF with the Student's t -distribution, i.e., TNMF. specifically, in order to reduce the impact of outliers on the algorithm, we use the Student's t -distribution to fit the data points instead of the Gaussian distribution. In addition, it is possible to adjust the Degree of Freedom (DF) to make the Student's t -distribution more flexible than the Gaussian distribution to fit data points when there are no outliers. Next, we combine the Automatic Relevance Determination (ARD) prior in our algorithm to simplify the model and allow for better performance of the algorithm. Finally, the article used 10 datasets to design two kinds of experiments, outlier removal and data clustering. The outlier removal results of this proposed algorithm are significantly better than the other methods, and it performs better in clustering compared to the other methods in the majority of cases.
更多查看译文
关键词
Non-negative Matrix Factorization (NMF),Student's t-distribution,Automatic Relevance Determination (ARD),Outlier removal,Clustering
AI 理解论文
溯源树
样例
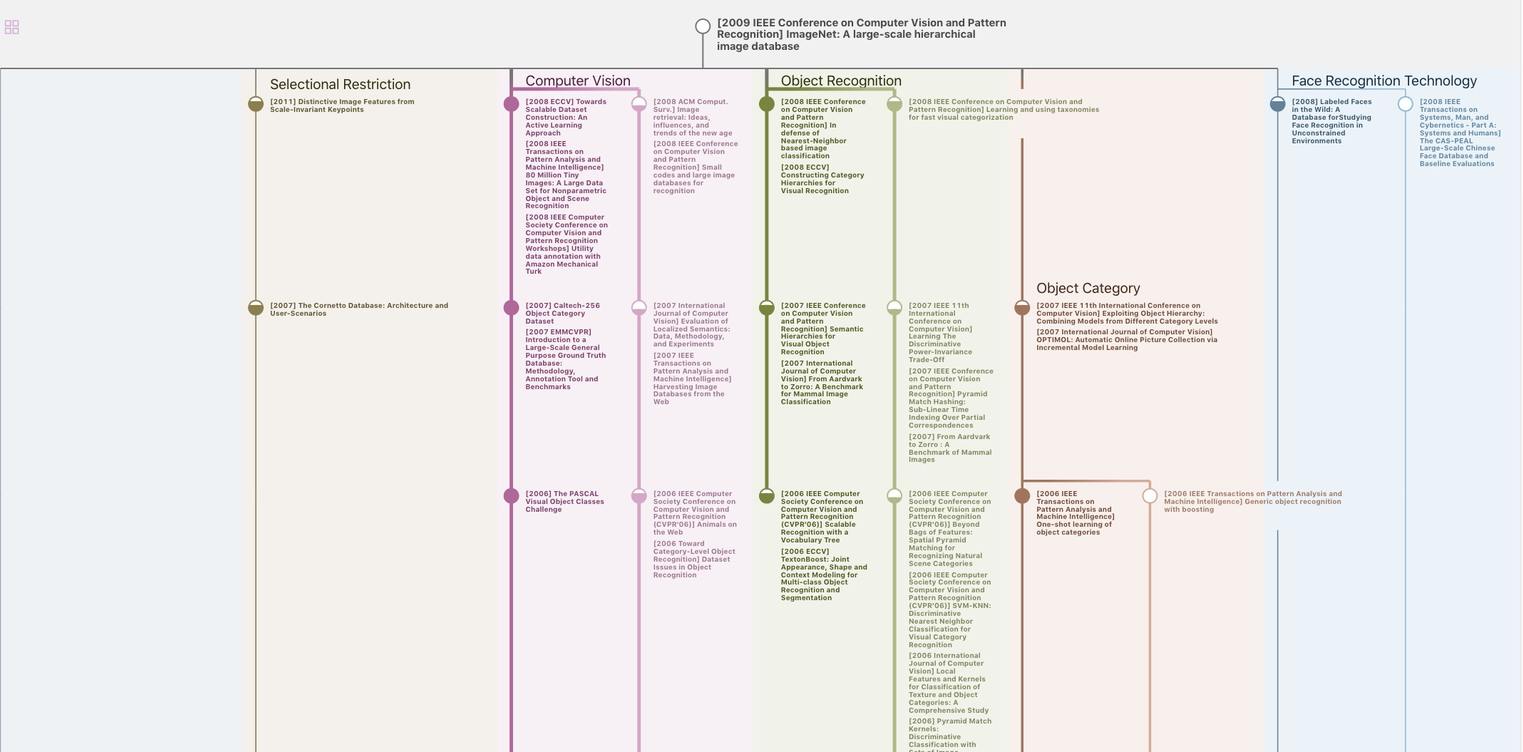
生成溯源树,研究论文发展脉络
Chat Paper
正在生成论文摘要