AlloyManufacturingNet for discovery and design of hardness-elongation synergy in multi-principal element alloys
ENGINEERING APPLICATIONS OF ARTIFICIAL INTELLIGENCE(2024)
摘要
Located around the center of multicomponent phase space, multi -principal element alloys (MPEAs) are often characterized with a unique blend of contrasting physico-chemical properties, and have a good prospective of presenting hardness -ductility synergy. A datasets of MPEAs fabricated by casting, wrought, sintering, annealing procedures, was collected and the mean values for hardness and elongation was determined as 495.3 HV and 22.16 % respectively. After screening and processing the datasets with the help of feature selection by tools such as Pearson's correlation coefficient, multicollinearity analysis and principal component analysis, artificial neural networks (ANNs) were subsequently trained in this study. An ensemble of these networks known as AlloyManufacturingNet was then purposed as the prediction toolkit for the inverse alloy design in applications including metamorphic manufacturing. During the in-silico design of external elements doped ZrHfNb and VNbTa alloys for maximum hardness, the model has estimated that Cr0.4W0.5(ZrHfNb)0.1 and Cr0.5W0.3(VNbTa)0.2, both fabricated by sintering method, show hardness values of 684.49 and 733.42 HV respectively. While Ti0.7(ZrHfNb)0.3 prepared via annealing procedure shows the largest elongation of 43.52 % in the category of ZrHfNb-derived alloys, Mo0.1Ti0.8(VNbTa)0.1 processed through the wrought process is estimated to have a maximum elongation of 34.36 % in the group of VNbTa-derived alloys. For establishment of the hardness -ductility synergy as required in structural applications, the composition spaces for given manufacturing routes have been searched with the machine learning model. In general, the MPEAs derived from base VNbTa alloy tend to perform better in context of possessing composition values having both the hardness and ductility above the aforementioned mean values. The machine learning based estimation was done in another MPEA, namely, CoCrNiNbx alloy to quantify the change in hardness with the change in Nb content, and the mechanistic insights were obtained via molecular dynamics simulations.
更多查看译文
关键词
Multi-principal element alloys,Feature selection,Ensemble neural network,Hardness-ductility synergy,Scale invariant optimization,Digital manufacturing
AI 理解论文
溯源树
样例
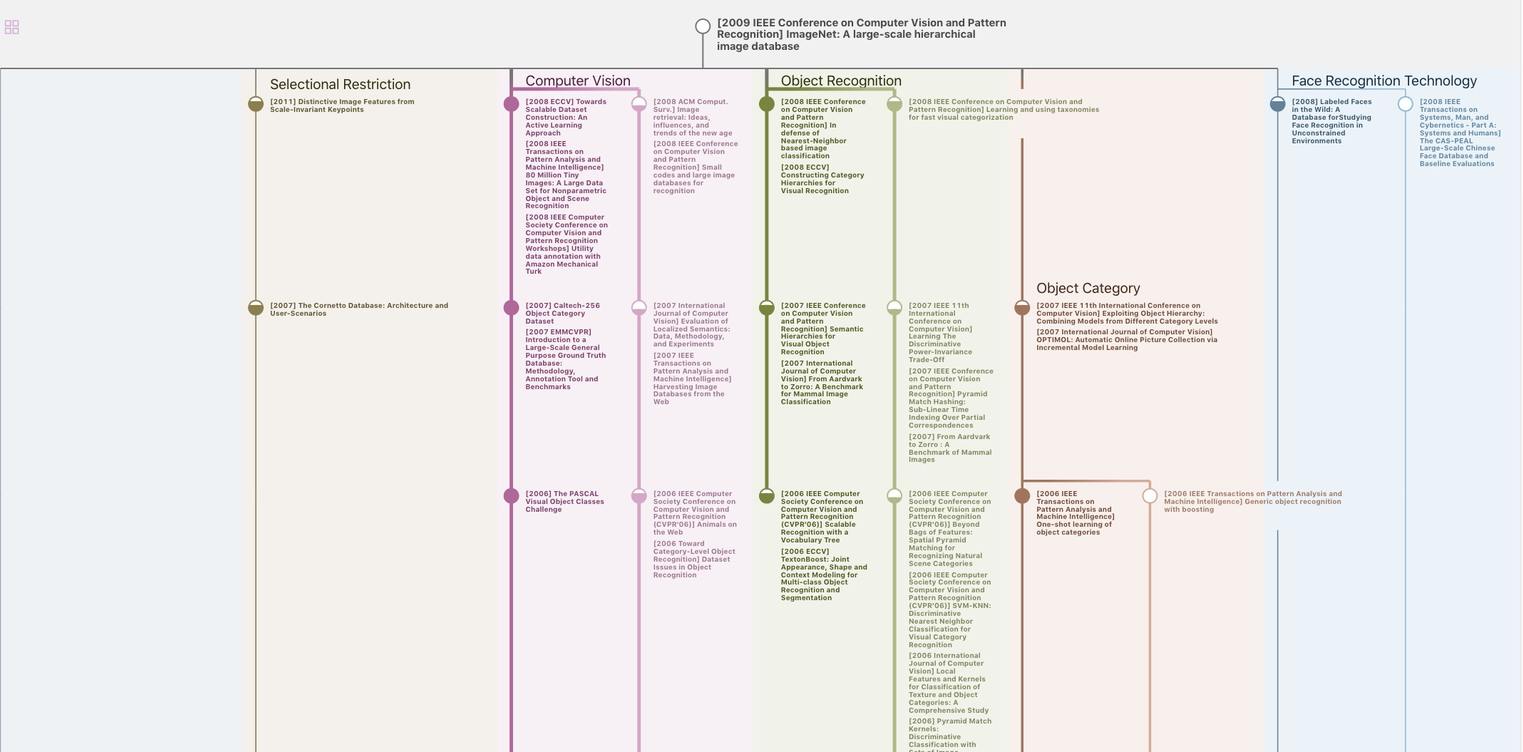
生成溯源树,研究论文发展脉络
Chat Paper
正在生成论文摘要