Optimization of Deep Neural Networks for Enhanced Efficiency in Small Scale Autonomous Vehicles
TRAITEMENT DU SIGNAL(2024)
摘要
Autonomous vehicles of the contemporary era constitute a sophisticated blend of artificial intelligence and electronic components. These vehicles operate autonomously by employing neural networks trained to interpret visual input from multiple onboard cameras and subsequently produce corresponding steering angles. However, the existing neural networks are characterized by their substantial scale, necessitating substantial GPU resources, and are prone to latency issues and complex architectural requirements. These factors render these networks unsuitable for small-scale applications where latency, complex architecture, and expensive hardware are prohibitive. This paper proposes a methodology for optimizing these neural networks for small-scale operations while preserving their accuracy and precision. This is achieved through a fine-tuning process that customizes the architecture and modifies various functional values and their parameters, resulting in a deep neural network tailored for small-scale applications. This optimized network boasts a simpler architecture, lower storage requirements, and reduced demand for GPU resources. The network is developed, trained, and evaluated using TensorFlow, a widely employed API for machine learning applications. The optimized network offers several advantages, including reduced latency, a customizable architecture, minimized memory requirements, and decreased GPU demand, making it a viable solution for various applications. The paper provides a detailed exploration of the development of this bespoke deep neural network and its potential implications for the future of small-scale autonomous vehicles.
更多查看译文
关键词
AI autopilot,autonomous vehicles,deep neural networks
AI 理解论文
溯源树
样例
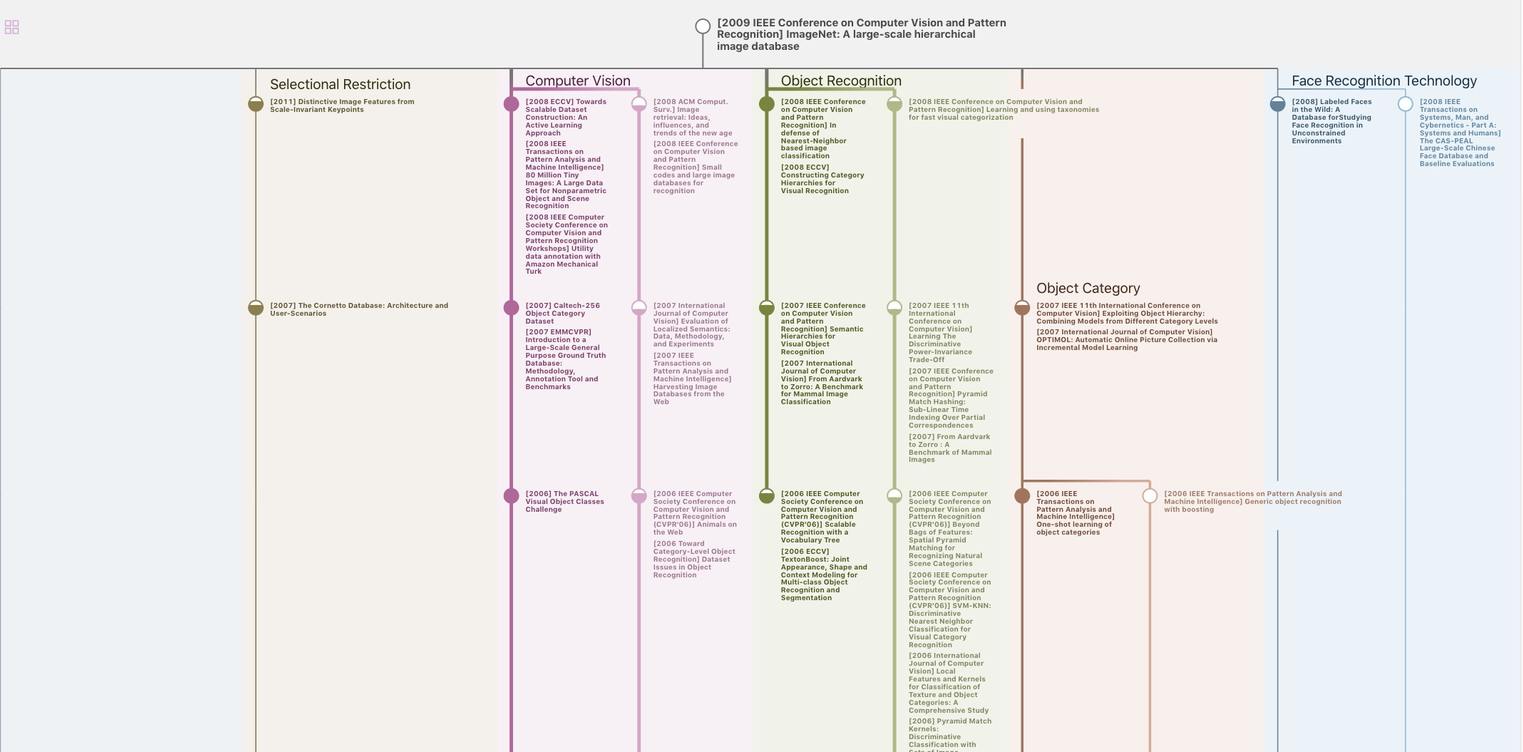
生成溯源树,研究论文发展脉络
Chat Paper
正在生成论文摘要