Temporal adaptive feature pyramid network for action detection
COMPUTER VISION AND IMAGE UNDERSTANDING(2024)
摘要
Detecting actions in videos has become a prominent research task due to its wide application. In addition to recognizing action category, this task also needs to localize the start time and end time of each action instance, which requires the model to have high temporal modeling capability. Moreover, the duration between each action instance is often different and highly variable. Although previous works have made attempts to address this difficulty, it is still a persistent problem. To further address the difficulty, we propose an action detection network using temporal feature pyramid, which can collect data using cameras and predict precise action categories and localizations. Specifically, we introduce a temporal adaptive module, which mixes self -attention and 1D convolution to flexibly adjust the temporal receptive field to improve the temporal modeling ability for different actions. We also propose a channel adaptive module to adjust channel weights and suppress useless information. We then propose the Temporal Adaptive Feature Pyramid Network (TAFPN) by integrating the two modules to adaptively extract multi -scale temporal information. We also improve the traditional parallel head into a unified head by stacking channel adaptive modules to simplify the network structure. Experimental results on the THUMOS14 dataset and ActivityNet1.3 dataset show that our method is competitive with state-of-the-art methods, which proves the effectiveness of our method.
更多查看译文
关键词
Action detection,Deep learning,Feature pyramid network,Self-attention,1D convolution
AI 理解论文
溯源树
样例
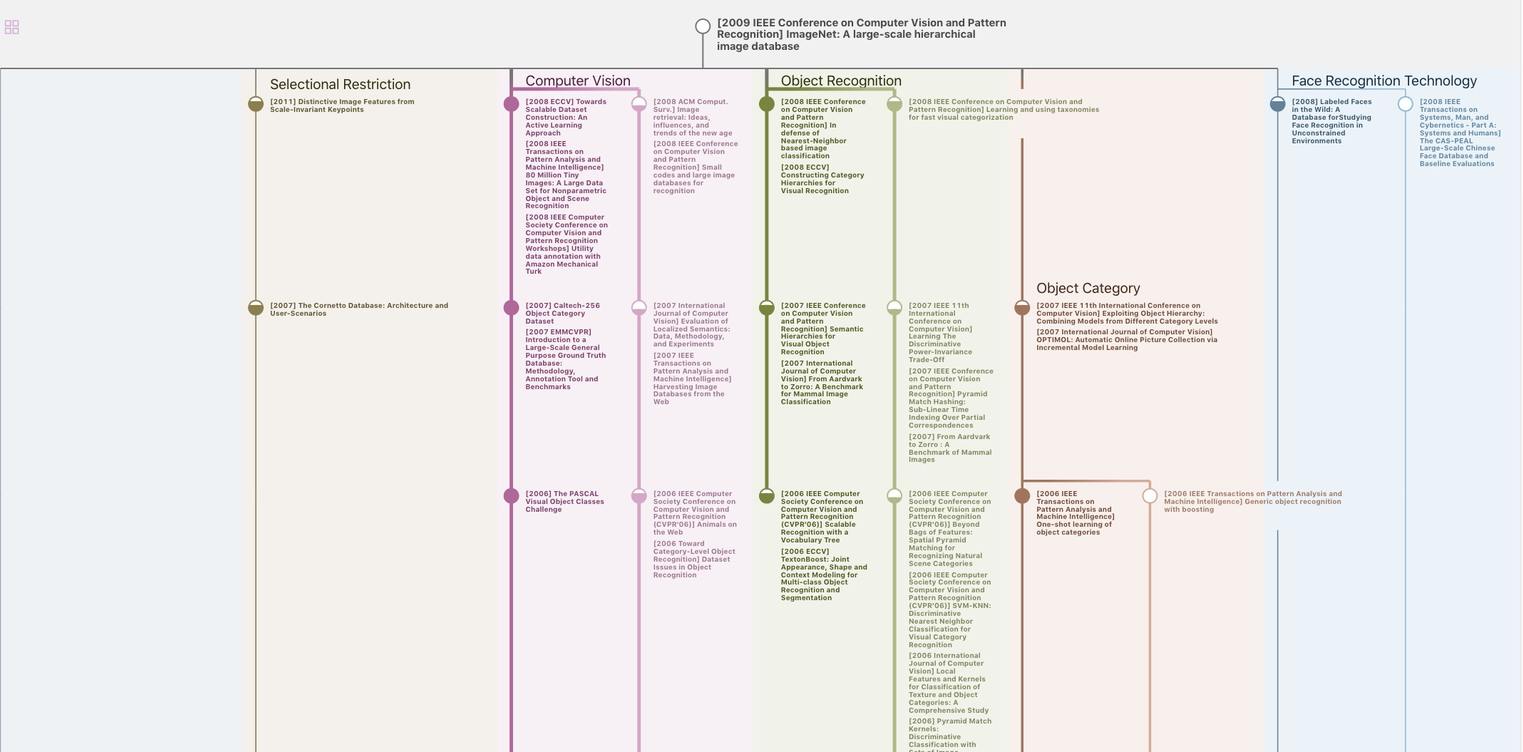
生成溯源树,研究论文发展脉络
Chat Paper
正在生成论文摘要