A Comparative Analysis of Machine Learning Algorithms for Breast Cancer Detection and Identification of Key Predictive Features
TRAITEMENT DU SIGNAL(2024)
摘要
Cancer, a disease with numerous subtypes, poses a deadly threat to human life, with the potential for successful clinical treatment heavily reliant on early detection and appropriate treatment planning. The classification of cancer patients into either low or high -risk subgroups is critical. Consequently, various research teams spanning the biomedical and bioinformatics fields have explored the use of Machine Learning (ML) technology in this crucial domain. The impressive capability of ML algorithms to discern significant features in complex datasets underscores their value. In the current study, we propose a framework to detect breast cancer (through benign and malignant categorization) utilizing advanced ML techniques with high accuracy. This framework deploys the Wisconsin Breast Cancer (Diagnostic) dataset. Five supervised ML techniques, namely Decision Tree, Random Forest (RF), Support Vector Machine (SVM), Extreme Gradient Boosting (XGBoost), and Artificial Neural Network (ANN), are trained for classification purposes. Out of 569 samples, 70% are allocated for training while the other 30% for testing. A comprehensive evaluation of ML techniques is performed using an array of metrics: precision, recall, specificity, F1 score, classification accuracy, ROC Curve, training time, and feature utilization. Additionally, feature importance is computed for each classifier. The results reveal that the SVM has the maximum accuracy as 97.66%, with an F1 -score of 0.98 for benign and 0.97 for malignant classifications. Conversely, the decision tree registers the minimum performance (94.55%) with an F1 -score of 0.95 for benign and 0.91 for malignant classes. Accuracy scores for RF, XGBoost, and ANN stand at 95.32%, 95.91%, and 97.07%, with corresponding F1 -scores of 0.96, 0.97, and 0.98 for benign and 0.94, 0.95, and 0.96 for malignant respectively. Interestingly, RF and XGBoost exhibited near -equivalent similarly with respect of accuracy measurements. In the context of the area over the ROC curve, SVM outperformed the other ML classifiers and also reported the shortest training time. Conversely, the ANN reported the longest training time.
更多查看译文
关键词
benign feature importance malignant,,supervised machine learning,feature selection,feature importance,malignant
AI 理解论文
溯源树
样例
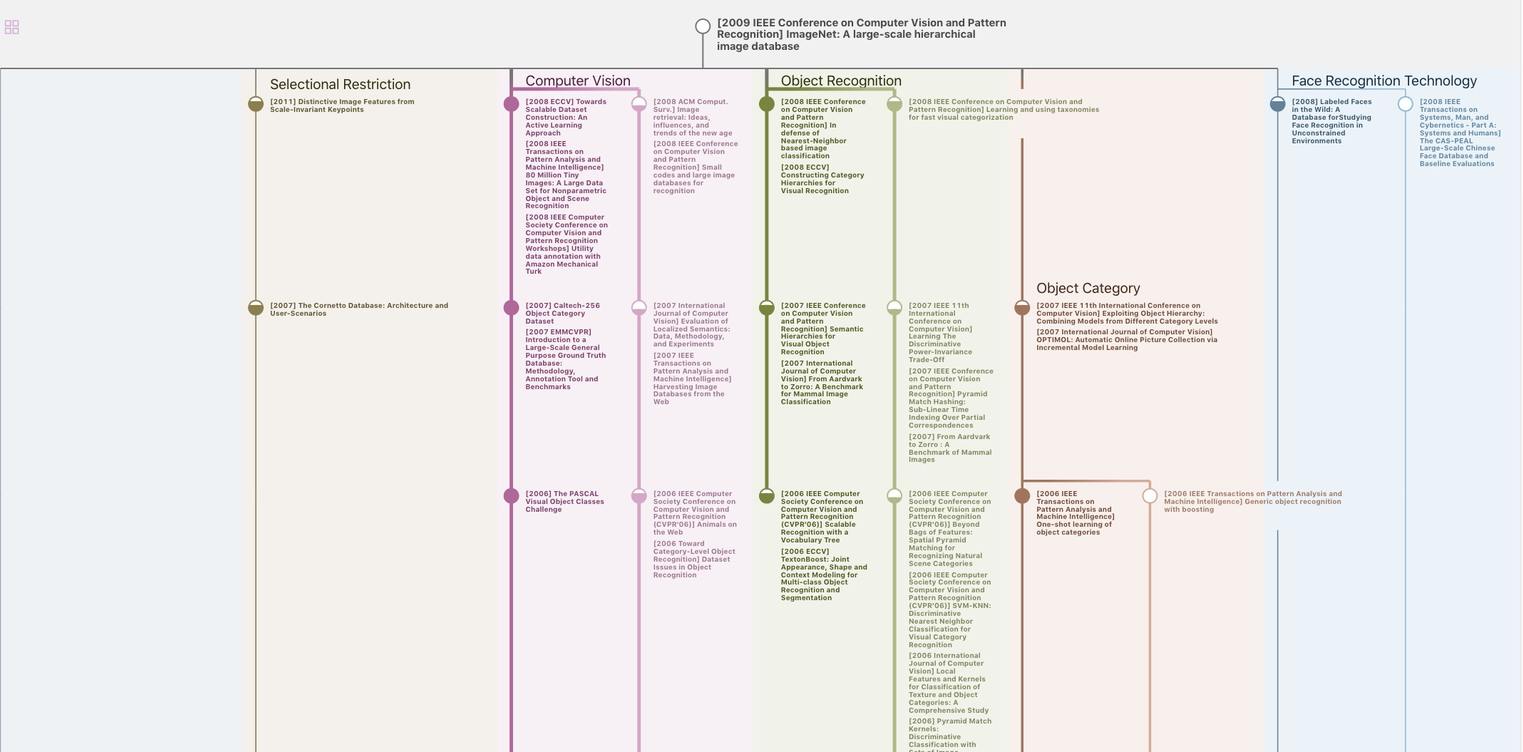
生成溯源树,研究论文发展脉络
Chat Paper
正在生成论文摘要