Prediction of PM2.5 using Super Learner Ensemble
JOURNAL OF KOREAN SOCIETY FOR ATMOSPHERIC ENVIRONMENT(2023)
摘要
PM2.5 is one of the air pollutants, the most of which are generated through chemical reactions involving emissions from fossil fuels, exhaust gases, and factories. Given PM2.5 ' s negative impact on society and health, the importance of prediction is increasing in response to growing public interest. In this study, we aimed to predict the concentration of PM2.5 in Jung-gu, Seoul, using machine learning methods. We collected data on various air pollutants (SO2, O-3, NO2, CO, PM10) that are known to be potential factors affecting PM2.5 levels. We employed seven different machine learning algorithms as base learners and utilized the Super Learner, which combines the predictions obtained from the weight averaging of the seven algorithms. The results indicated that ensemble models, such as Random Forest, Gradient Boosting, and eXtreme Gradient Boosting, exhibited superior predictive performance compared to other base learners. However, most base learners struggled to accurately predict the high concentrations of PM2.5 during the test period. In contrast, the Super Learner delivers more accurate predictions for high- concentration observations, ultimately improving prediction results compared to the base learners.
更多查看译文
关键词
PM2.5,Machine learning,Base learners,Super Learner
AI 理解论文
溯源树
样例
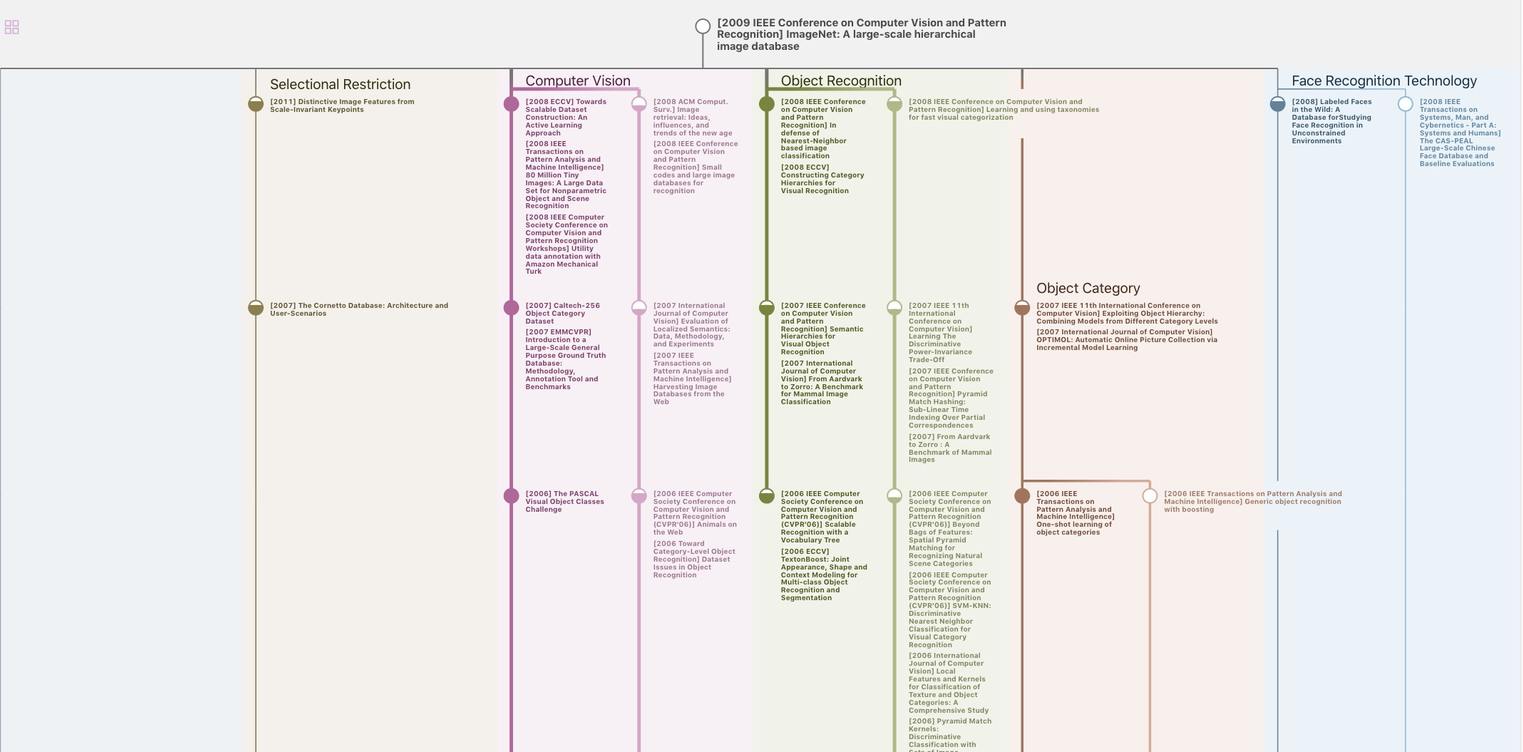
生成溯源树,研究论文发展脉络
Chat Paper
正在生成论文摘要