Emotion recognition based on multimodal physiological signals using spiking feed-forward neural networks
BIOMEDICAL SIGNAL PROCESSING AND CONTROL(2024)
摘要
Real -time emotion recognition via wearable devices is a pivotal component of health monitoring and human- computer interaction. To realize this objective, a spiking feed-forward neural networks (SFNNs) model was developed, which leverages six physiological signals from the psychophysiology of positive and negative emotions (POPANE) dataset to construct feature vectors. By converting well-trained artificial neural networks (ANNs) to spiking neural networks (SNNs) and employing weight normalization techniques, the SFNNs with data-based normalization achieved a maximum classification accuracy of 88.17% at a maximum input firing rate of 1000 Hz. In comparison to existing models, the SFNNs model integrates multimodal physiological signals to classify six discrete emotions, demonstrating high classification performance and rapid convergence speed, rendering it ideal for real -time emotion recognition. This work has potential applications in psychological diagnosis and medical rehabilitation through the use of wearable wristbands.
更多查看译文
关键词
Emotion recognition,Multimodal physiological signals,Time series,Feature extraction,Spiking feed-forward neural networks,Wearable wristbands
AI 理解论文
溯源树
样例
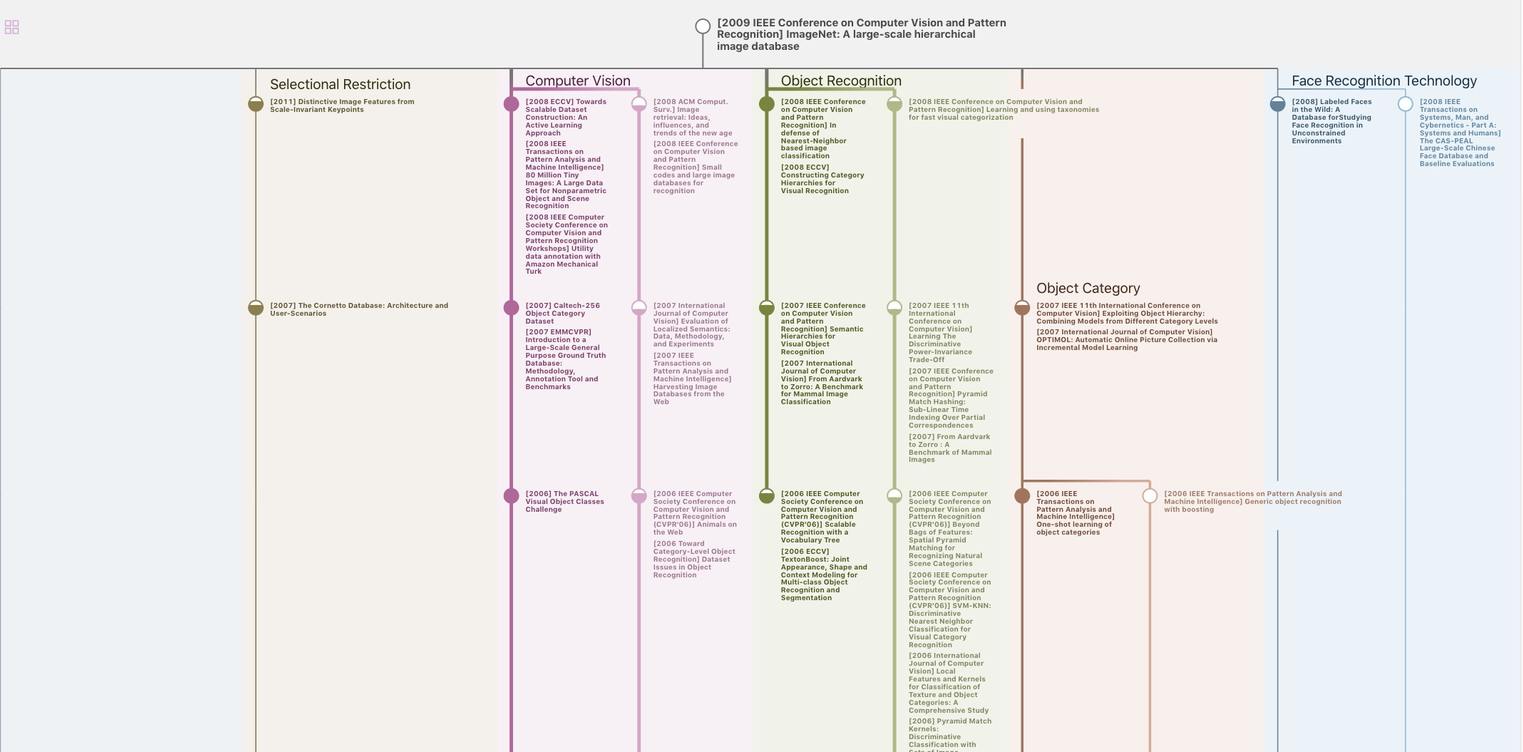
生成溯源树,研究论文发展脉络
Chat Paper
正在生成论文摘要