Automated Text Analysis of Organic Chemistry Students' Written Hypotheses
JOURNAL OF CHEMICAL EDUCATION(2024)
摘要
Generating a testable hypothesis is a necessary skill for engaging in science, requiring both general reasoning skills and specific content knowledge of the phenomenon being investigated. While many students have the reasoning skills necessary for developing testable hypotheses in a general science context, it can be challenging for students to apply these skills to content areas where the phenomena being studied are unobservable, such as problems in organic chemistry. Generating hypotheses for experiments is a skill that students are expected to apply in chemistry teaching laboratories. Students can benefit from receiving real-time feedback to support developing this ability. However, providing feedback on writing can be challenging for instructors, especially in large-enrollment courses. For this study, we explored and compared the performance of several machine learning algorithms to classify organic chemistry students' written hypotheses for a science-general scenario and an organic chemistry-specific scenario. These models were trained to analyze students' written hypotheses for the presence of five features: (1) a testable prediction in a given situation; (2) a predicted change in the independent variable that is related to the dependent variable; (3) scientific content addressing the research question provided in the prompt; (4) a correct and completely defined independent variable; and (5) a correct and completely defined dependent variable. This work has implications for guiding future research on the feasibility of providing instructors with information about students' hypothesis-generating abilities that can support the delivery of real-time feedback.
更多查看译文
关键词
Organic Chemistry,Undergraduate Education,Hypothesis,Machine Learning,Automated Text Analysis,Formative Feedback
AI 理解论文
溯源树
样例
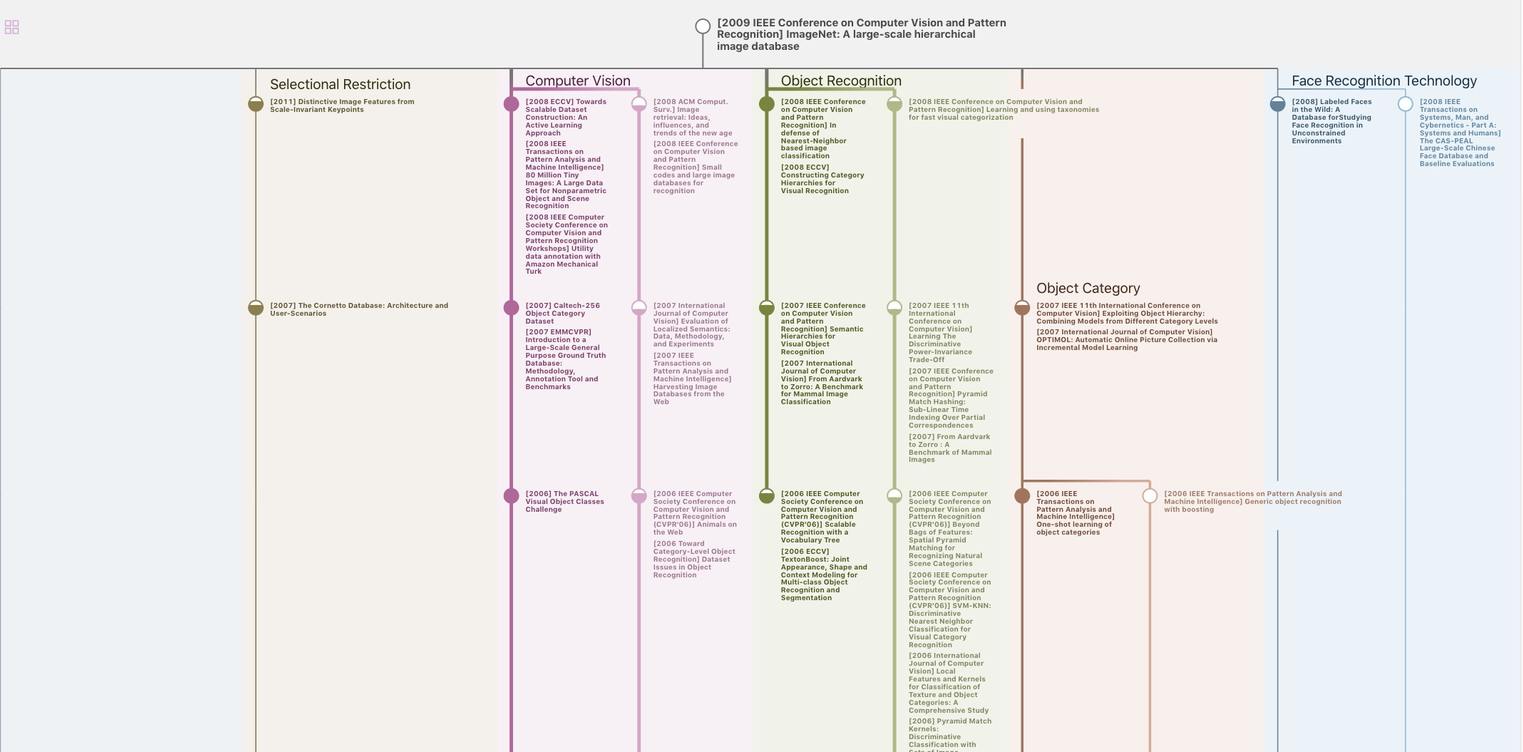
生成溯源树,研究论文发展脉络
Chat Paper
正在生成论文摘要