Evaluation of concrete's fracture toughness under an acidic environment condition using advanced machine learning algorithms
ENGINEERING FRACTURE MECHANICS(2024)
摘要
The assessment of concrete fracture toughness in acidic environments holds paramount importance, given its substantial influence on the mechanical characteristics of concrete. Concrete's widespread use in civil engineering, driven by its cost-effectiveness and abundance, underscores the need to comprehend and address potential damage stemming from fractures and cracks-issues that may pose challenges in terms of repair feasibility. Furthermore, exposure to acidic conditions can lead to diminished mechanical strength in concrete structures. Consequently, scrutinizing the mechanical properties of concrete within acidic environments becomes pivotal for informing construction design and ensuring effective maintenance strategies. This study investigates the impact of an acidic environment on the effective fracture toughness (Keff) of three concrete types: conventional concrete, concrete containing glass fiber and microsilica, and concrete containing glass fiber. The central straight-notched Brazilian disc (CSNBD) test was utilized. However, conducting laboratory tests to measure the concrete's Keff can be expensive and time-consuming. To this end, fifteen supervised and unsupervised learning algorithms were proposed in this study based on 420 datasets generated from the CSNBD test. The performance and accuracy of the applied models were evaluated and compared to the laboratory results. The results confirmed the acceptable performance of the models to estimate the Keff of concrete. The CSNBD and soft computing results indicated that the acidic environment is more damaging to concrete's Keff than the neutral one. Moreover, reinforced concrete consistently resisted acidic environments more than conventional concrete. Additionally, sensitivity analysis and importance ranking identified the minimal impact of crack length and sample thickness on the Keff, while crack inclination angle and diameter had the most significant influence. Various loss functions were employed to evaluate the performance and accuracy of the applied methods. Notably, the proposed models demonstrated satisfactory and reliable accuracy, with all models exhibiting an R2 value above 0.7 and loss function values approaching zero. The results highlighted the superior precision of the supervised learning algorithms for evaluating the Keff of concrete, particularly the gene expression programming (GEP) model, with an R2 value of 0.91 and 0.82 for training and testing, respectively.
更多查看译文
关键词
Fracture toughness,Reinforced concrete,Acidic environment,Soft computing algorithms
AI 理解论文
溯源树
样例
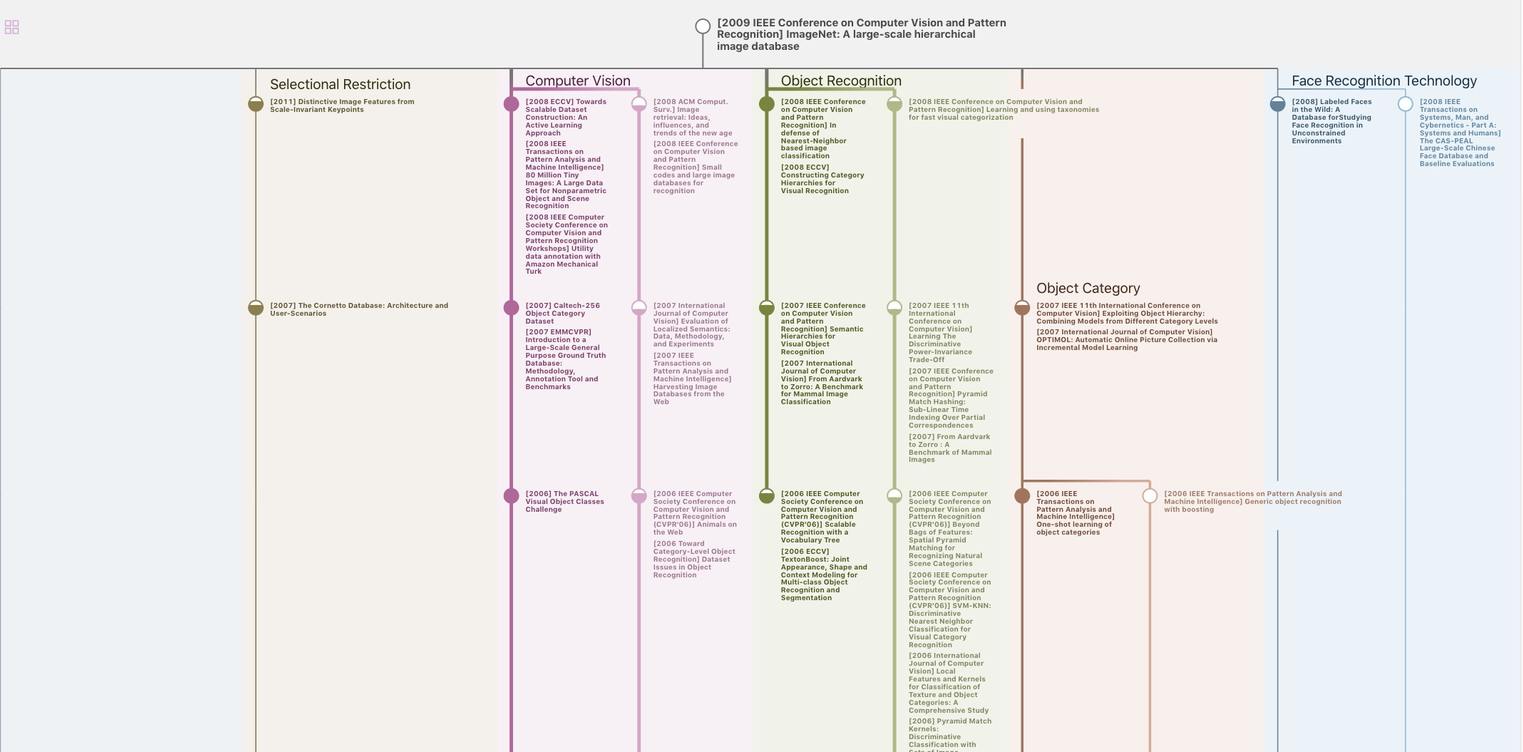
生成溯源树,研究论文发展脉络
Chat Paper
正在生成论文摘要