Physics-Informed Deep Operator Learning Based on Reduced-Order Modeling for Retrieving the Ocean Interior Density From the Surface
JOURNAL OF GEOPHYSICAL RESEARCH-OCEANS(2024)
摘要
Exploring methods to reconstruct the ocean interior from surface data is a crucial focus in the study of ocean processes and phenomena due to the shortage of subsurface and deep-sea data. Nonetheless, the existing methods predominantly concentrate on either data-driven or dynamical methodologies, with limited exploration of integrating the strengths of both approaches. To combine the advantages of these two methods for reconstructing the subsurface density field from surface data, a novel dynamics-constrained deep operator learning network based on reduced-order model is proposed. Encoding the mean-squared residuals of the reduced-order equation along with the mean-squared error between the network outputs and targets into the loss function effectively merges the dynamical and data constraints during the training process. This integration makes the network outputs and inputs approximately satisfy a specific form of the equation, allowing for interpretability, and once the network is well-trained, rapid reconstruction evaluation can be performed. The reduced-order equation is established by the Galerkin projection of quasi-geostrophic equation onto the low-dimensional subspace identified via reduced-basis, which explains the vertical variation of ocean density. The developed model can tackle the challenge of directly measuring subsurface potential vorticity and predicting subsurface density. Evaluation is conducted using simulation data from the Max-Planck-Institute ocean model, indicating that it can offer precise estimations, outperforms the purely data-driven algorithm presented in the paper and the interior plus surface quasi-geostrophic method, and enables model sharing across different regions. Reconstructing the interior density of the ocean from surface data is a valuable way to make up for the lack of ocean observations. Most of the previous works are purely data-driven or dynamics-based methods. In order to employ the advantages of dynamic and data-driven models at the same time, a physics-informed deep operator learning algorithm is proposed to reconstruct the density field. The proposed method shows superior predictive performance and greater robustness when compared with the pure data-driven and dynamics-based methods presented in the paper. These findings underscore the potential of deep learning algorithms incorporating physical constraints for marine scientific research. The deep operator learning algorithm based on physical constraints is proposed for density field inversion The combination of deep learning and dynamics-based methods provides interpretability while enabling fast density reconstruction The proposed reconstruction framework outperforms proposed purely data-driven algorithm and traditional dynamics algorithm
更多查看译文
AI 理解论文
溯源树
样例
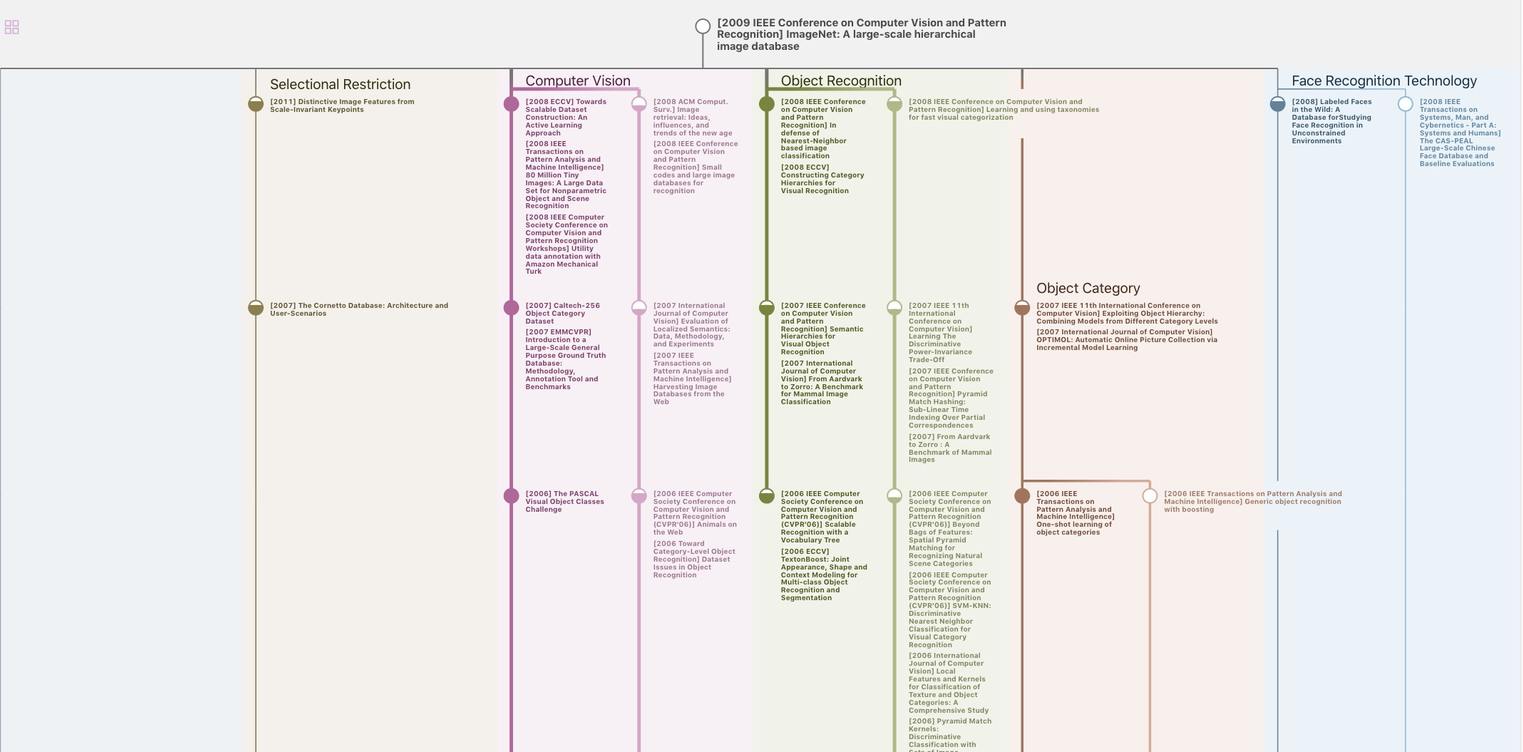
生成溯源树,研究论文发展脉络
Chat Paper
正在生成论文摘要