Generative machine learning methods for multivariate ensemble postprocessing
ANNALS OF APPLIED STATISTICS(2024)
摘要
Ensemble weather forecasts based on multiple runs of numerical weather prediction models typically show systematic errors and require postprocessing to obtain reliable forecasts. Accurately modeling multivariate dependencies is crucial in many practical applications, and various approaches to multivariate postprocessing have been proposed where ensemble predictions are first postprocessed separately in each margin and multivariate dependencies are then restored via copulas. These two-step methods share common key limitations, in particular, the difficulty to include additional predictors in modeling the dependencies. We propose a novel multivariate postprocessing method based on generative machine learning to address these challenges. In this new class of nonparametric data-driven distributional regression models, samples from the multivariate forecast distribution are directly obtained as output of a generative neural network. The generative model is trained by optimizing a proper scoring rule, which measures the discrepancy between the generated and observed data, conditional on exogenous input variables. Our method does not require parametric assumptions on univariate distributions or multivariate dependencies and allows for incorporating arbitrary predictors. In two case studies on multivariate temperature and wind speed forecasting at weather stations over Germany, our generative model shows significant improvements over state-of-the-art methods and particularly improves the representation of spatial dependencies.
更多查看译文
关键词
Generative machine learning,ensemble postprocessing,multivariate postprocessing,probabilistic forecasting,weather forecasting
AI 理解论文
溯源树
样例
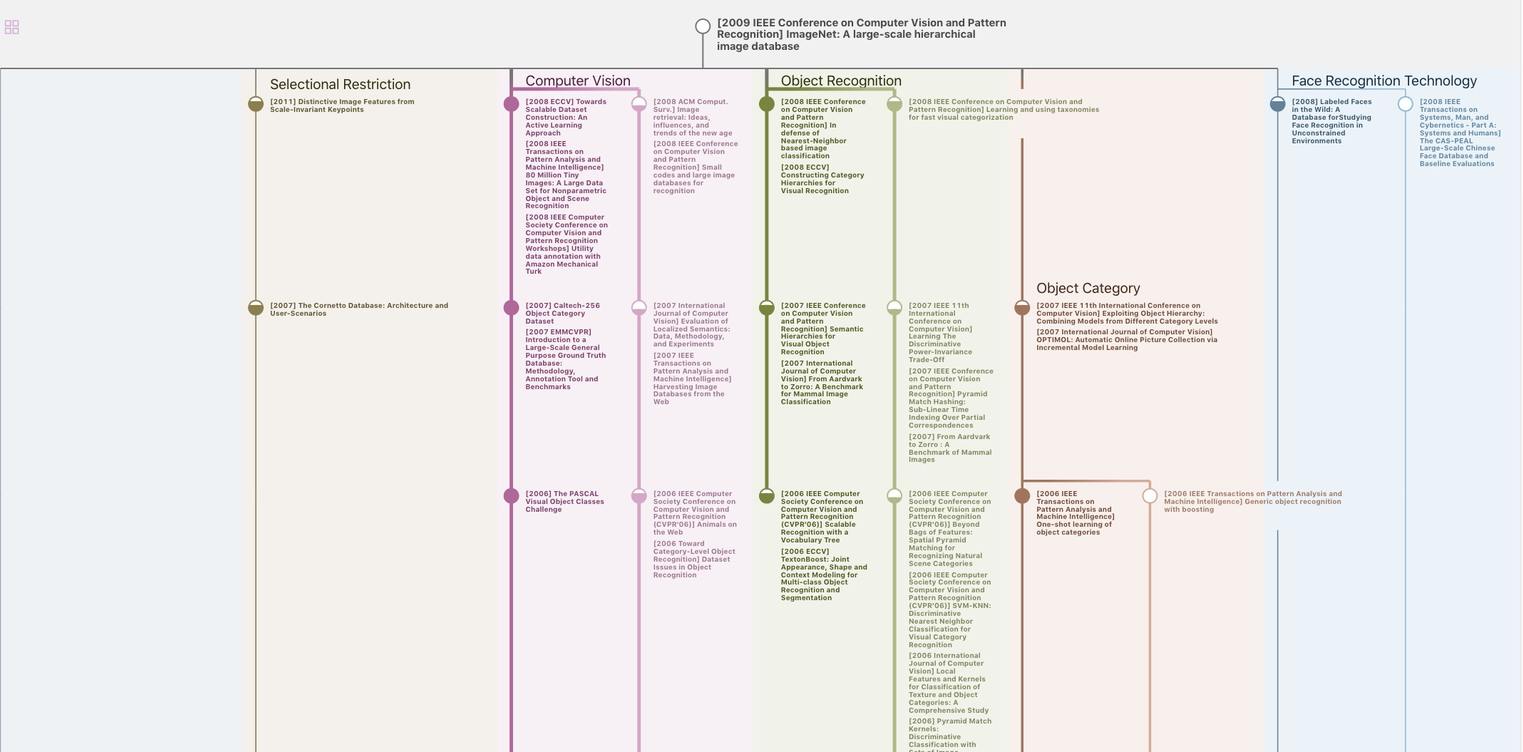
生成溯源树,研究论文发展脉络
Chat Paper
正在生成论文摘要