Modelling potential land suitability of large-scale wind energy development using explainable machine learning techniques: Applications for China, USA and EU
ENERGY CONVERSION AND MANAGEMENT(2024)
摘要
Spatial siting decisions for wind energy utilization are known to be greatly influenced by complex drivers, such as climate, geography, and socio-economics. However, traditional multi-criteria decision making methods (MCDM) inevitably subject to subjectivity and uncertainties in weight determination of assessment index, which may lead into the mismatch between real-world practices and evaluation outcomes. Here, we proposed a novel data-driven machine learning-based modeling framework to predict potential land suitability of large-scale wind energy development by combining the national level inventory of existing wind farms and various spatial decision criteria. Three major wind energy application economies (China, USA, and EU) were selected as the case study. Four advanced machine learning algorithms, Deep Neural Network (DNN), Random Forest (RF), XGBoost and LightGBM, were adopted to build the prediction model. Thirty conditioning factors were chosen as informative reflecting biophysical, geographical, socio-economic, landscape composition and configuration properties for modelling optimized wind farms site. Further, three model interpretation techniques including variable importance, partial dependence plots, as well as Shapley additive explanation (SHAP), were used to discern the contribution of individual variables and explain their nonlinear driving effects on the wind energy utilization. Results showed that RF models achieved the highest predictive accuracy (AUC > 0.91) among the other algorithms for the testing dataset. Local wind energy resource endowments including capacity factor (CF) and average wind speed (WS) are the most important decision-making criteria of wind farms siting for all three economics, which confirmed that a resource-oriented layout pattern was currently followed by wind energy development. Furthermore, China has a relatively relaxed standards of wind farms location selection among three economies (such as the threshold of CF close to 0.23 and threshold of WS close to 6.5 w/s). According to high-resolution gridded probability prediction mapping derived from optimized RF model, we identified high priority development zones occupied about 14-29 % of total land area in each economy, with estimated technical potential of 5028 TWh/year for China, 2297 TWh/year for USA, 1114 TWh/year for EU, respectively. An ensemble learning framework provides valuable insights and useful practical tools for optimizing renewable energy capacity expansion planning, informing policy development, and promoting the long-term sustainability of wind energy development.
更多查看译文
关键词
Wind energy,Potential land suitability,Explainable machine learning,Shapley additive explanation (SHAP)
AI 理解论文
溯源树
样例
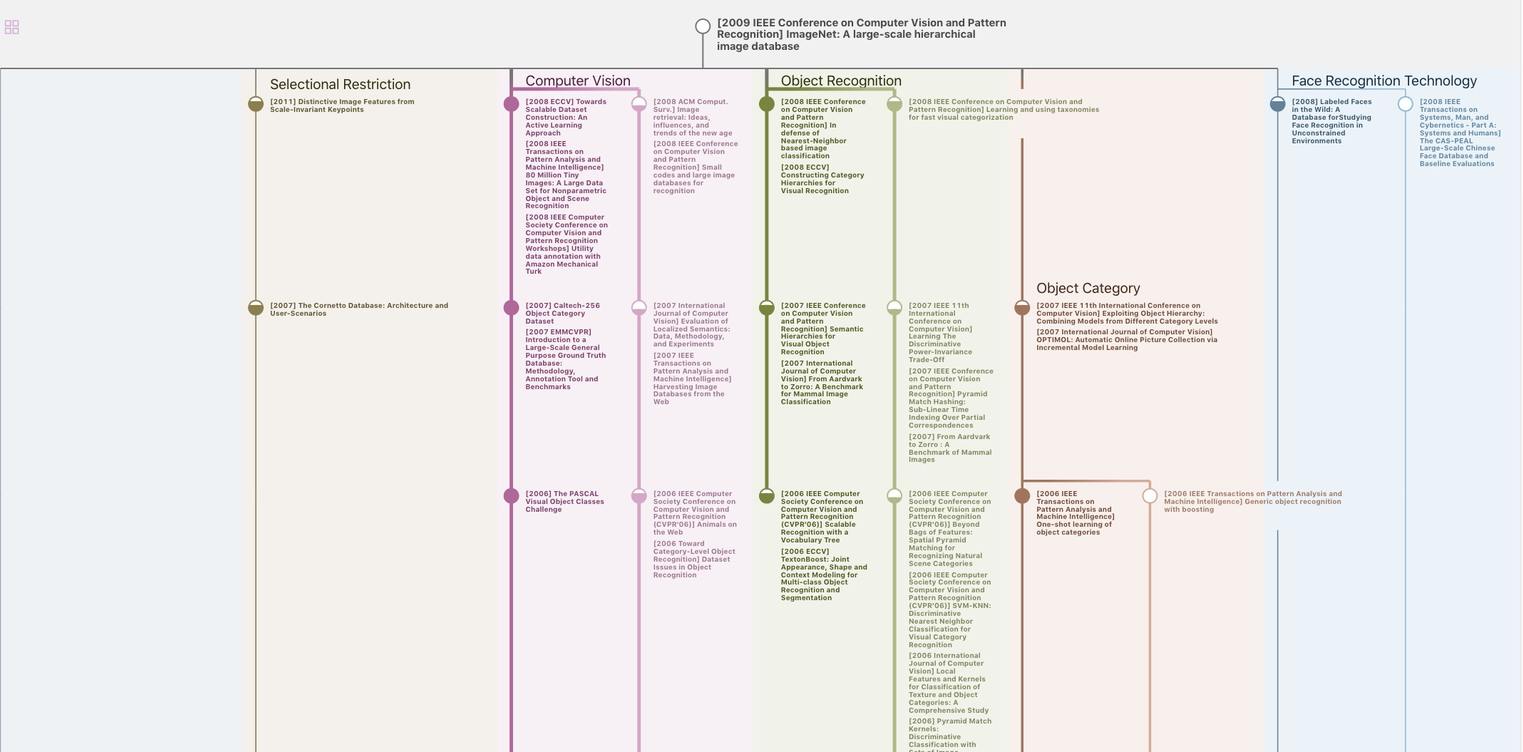
生成溯源树,研究论文发展脉络
Chat Paper
正在生成论文摘要