Statistically conditioned polycrystal generation using denoising diffusion models
ACTA MATERIALIA(2024)
摘要
Synthetic microstructure generation algorithms have emerged as a key tool for enabling large ICME and Materials Informatics efforts. In particular, statistically conditioned generative models allow researchers to systematically explore complex design spaces encountered in microstructure design. In spite of the engineering importance of polycrystalline materials, generative frameworks for these systems remain extremely limited. This stunted development - in comparison to the N -phase microstructure generation problem - occurs because of the complexities inherent to the representation of the polycrystalline orientation fields. For example, these fields exhibit multiple crystal- and sample -level symmetries. In prior work, these difficulties have resulted in instabilities in deep generative models for polycrystalline microstructures. In this work, we propose the use of a Reduced -Order Generalized Spherical Harmonic (ROGSH) basis to address the challenge described above. The proposed approach accounts for the complex sample- and crystal -level symmetries, and produces well behaved and low dimensional representations whose space has a meaningful Euclidean measure. We then demonstrate the ROGSH basis's remarkable ability to produce stable denoising diffusion models by using our recently established Local-Global generative framework to create visually realistic synthetic polycrystalline microstructures. Furthermore, we demonstrate that the generation process can be conditioned on both firstand second -order spatial statistics of the polycrystalline orientation fields.
更多查看译文
关键词
Polycrystalline microstructure generation,Conditional microstructure generation,2-Point statistics,Denoising diffusion models,Spherical harmonics
AI 理解论文
溯源树
样例
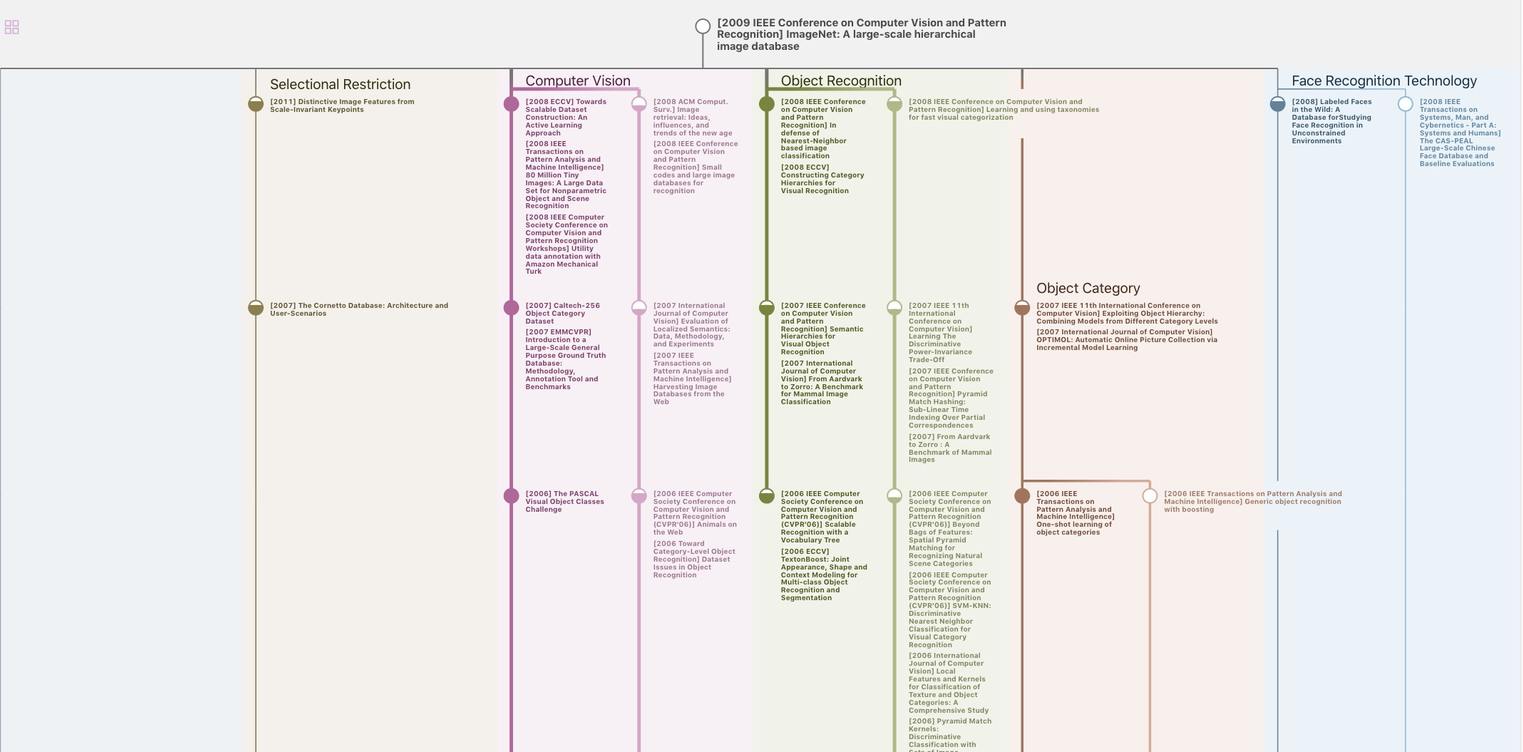
生成溯源树,研究论文发展脉络
Chat Paper
正在生成论文摘要