Advancements in memory technologies for artificial synapses
JOURNAL OF MATERIALS CHEMISTRY C(2024)
摘要
Neural networks (NNs) have made significant progress in recent years and have been applied in a broad range of applications, including speech recognition, image classification, automatic driving, and natural language processing. The hardware implementation of NNs presents challenges, and research communities have explored various analog and digital neuronal and synaptic devices for resource-efficient implementation. However, these hardware NNs face several challenges, such as overheads imposed by peripheral circuitry, speed-area tradeoffs, non-idealities associated with memory devices, low on-off resistance ratio, sneak path issues, low weight precision, and power-inefficient converters. This article reviews different synaptic devices and discusses the challenges associated with implementing these devices in hardware, along with corresponding solutions, and prospecting future research directions. Several categories of emerging synaptic devices such as resistive random-access memory (RRAM), phase change memory (PCM), analog-to-digital hybrid volatile memory-based, ferroelectric field effect transistor (FeFET)-based, spintronic-based spin transfer, spin-orbit, magnetic domain wall (DW) and skyrmion synaptic devices have been explored, and a comparison between them is presented. This study provides insights for researchers engaged in the field of hardware neural networks. This article reviews different synaptic devices and discusses the challenges associated with implementing these devices in hardware, along with corresponding solutions, applications, and prospecting future research directions.
更多查看译文
AI 理解论文
溯源树
样例
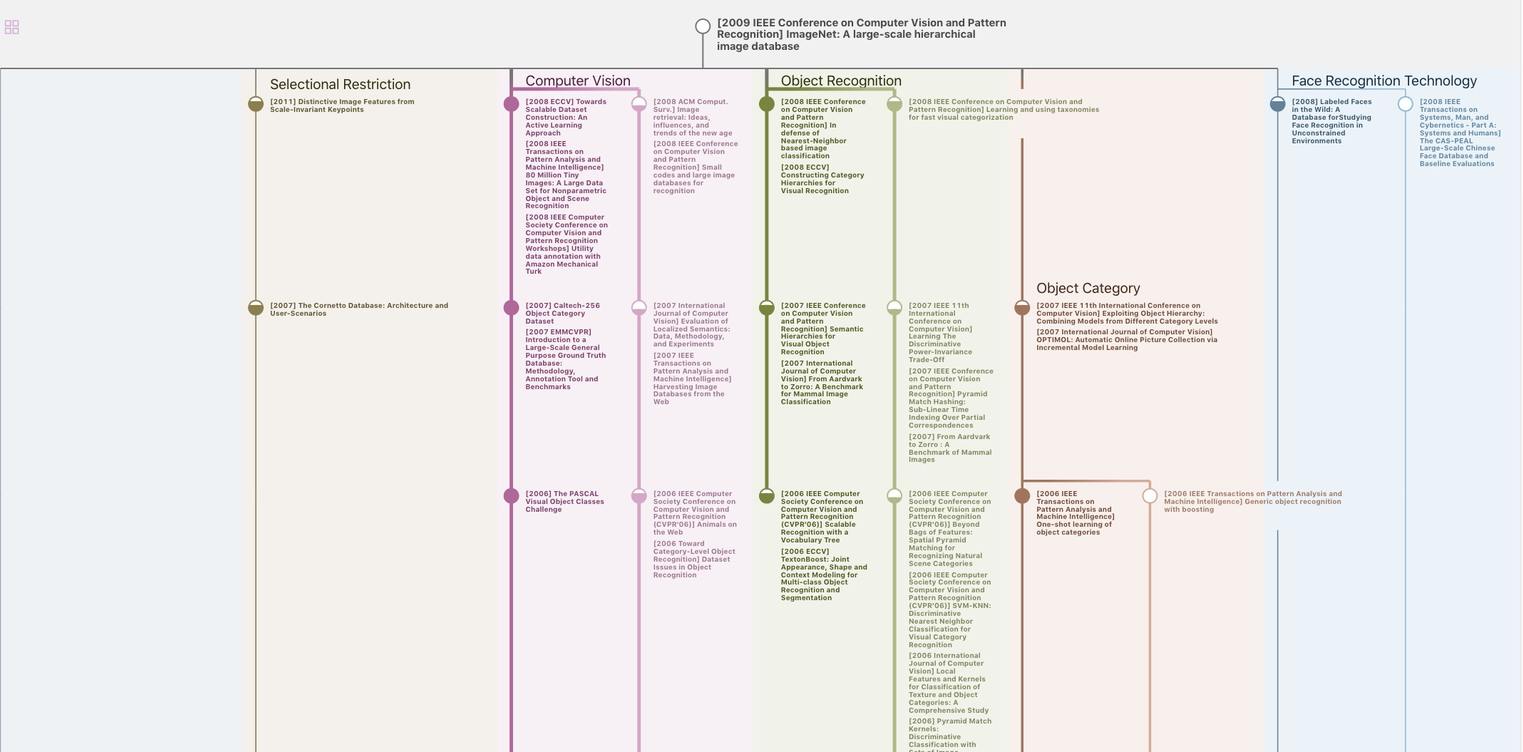
生成溯源树,研究论文发展脉络
Chat Paper
正在生成论文摘要