Interpretable machine learning-assisted design of Fe-based nanocrystalline alloys with high saturation magnetic induction and low coercivity
JOURNAL OF MATERIALS SCIENCE & TECHNOLOGY(2024)
摘要
Overcoming the trade-off between saturation magnetic induction (B-s) and coercivity (H-c) of Fe-based nanocrystalline alloys (FNAs) remains a great challenge due to the traditional design relying on trial-and-error methods, which are time-consuming and inefficient. Herein, we present an interpretable machine learning (ML) algorithm for the effective design of advanced FNAs with improved B-s and low H-c. Firstly, the FNAs datasets were established, consisting of 20 features including chemical composition, process parameters, and theoretically calculated parameters. Subsequently, a three-step feature selection was used to screen the key features that affect the B-s and H-c of FNAs. Among six different ML algorithms, extreme gradient boosting (XGBoost) performed the best in predicting B-s and H-c. We further revealed the association of key features with B-s and H-c through linear regression and SHAP analysis. The valence electron concentration without Fe, Ni, and Co elements (VEC1) and valence electron concentration (VEC) ranked as the most important features for predicting B-s and H-c, respectively. VEC1 had a positive impact on B-s when VEC1 < 0.78, while VEC had a negative effect on H-c when VEC < 7.12. Optimized designed FNAs were successfully prepared, and the prediction errors for B-s and H-c are lower than 2.3 % and 18 %, respectively, when comparing the predicted and experimental results. These results demonstrate that this ML approach is interpretable and feasible for the design of advanced FNAs with high B-s and low H-c. (c) 2024 Published by Elsevier Ltd on behalf of The editorial office of Journal of Materials Science & Technology.
更多查看译文
关键词
Nanocrystalline alloy,Machine learning,Feature selection,Saturation magnetic induction,Coercivity
AI 理解论文
溯源树
样例
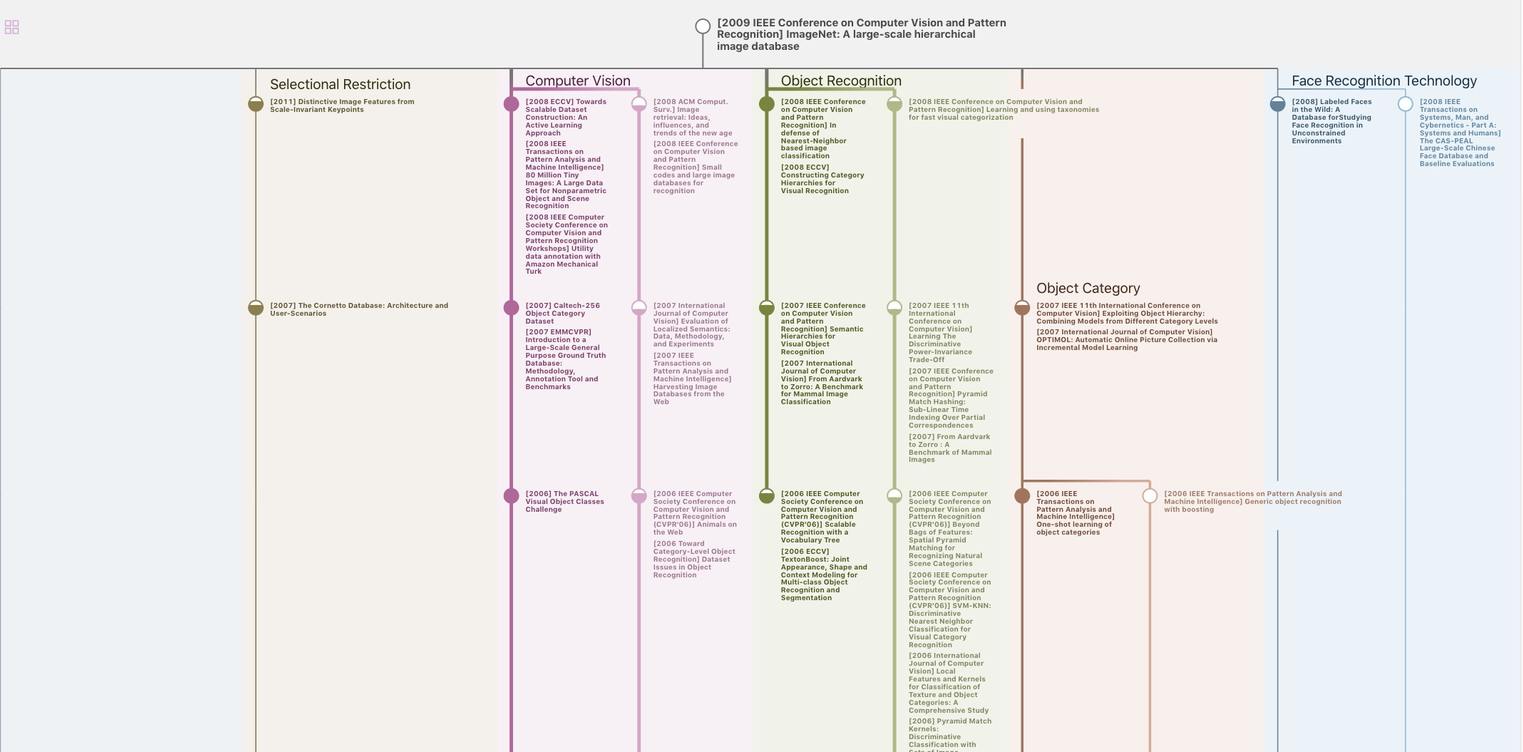
生成溯源树,研究论文发展脉络
Chat Paper
正在生成论文摘要