A data-driven framework for learning governing equations of Li-ion batteries and co-estimating voltage and state-of-charge
JOURNAL OF ENERGY STORAGE(2024)
摘要
This paper presents a reduced -order nonlinear model for Lithium -ion batteries (LiBs). Unlike mechanistic models, data -driven models offer accurate representations of system dynamics without relying on in -situ measurements and proprietary information. However, these models may perform poorly in unseen scenarios due to overfitting training data, which is typical. We propose a physics -inspired, data -driven approach to determine LiBs governing equations based on their electrochemistry rather than generic terms. We employ a sparse identification method achieved through sequentially thresholding ridge regression to construct a nonlinear model from electrical current (excitation input) and measured voltage. We formulate the problem to optimize the sparsification parameters as hyperparameters and minimize a cost function comprised of training and validation sets and the number of terms as a measure of complexity. We augment the model with a joint unscented Kalman filter to handle noisy experimental data, enabling a more accurate estimate of the state of charge (SOC) and voltage. Model performance in unseen scenarios is evaluated with urban dynamometer driving schedule (UDDS) data, where the identified model achieves a root mean square error of 1.26e-2 for SOC and voltage prediction.
更多查看译文
关键词
Energy storage,Sparse modeling,SINDyC,Joint unscented Kalman filter,SOC prediction,Data-driven modeling
AI 理解论文
溯源树
样例
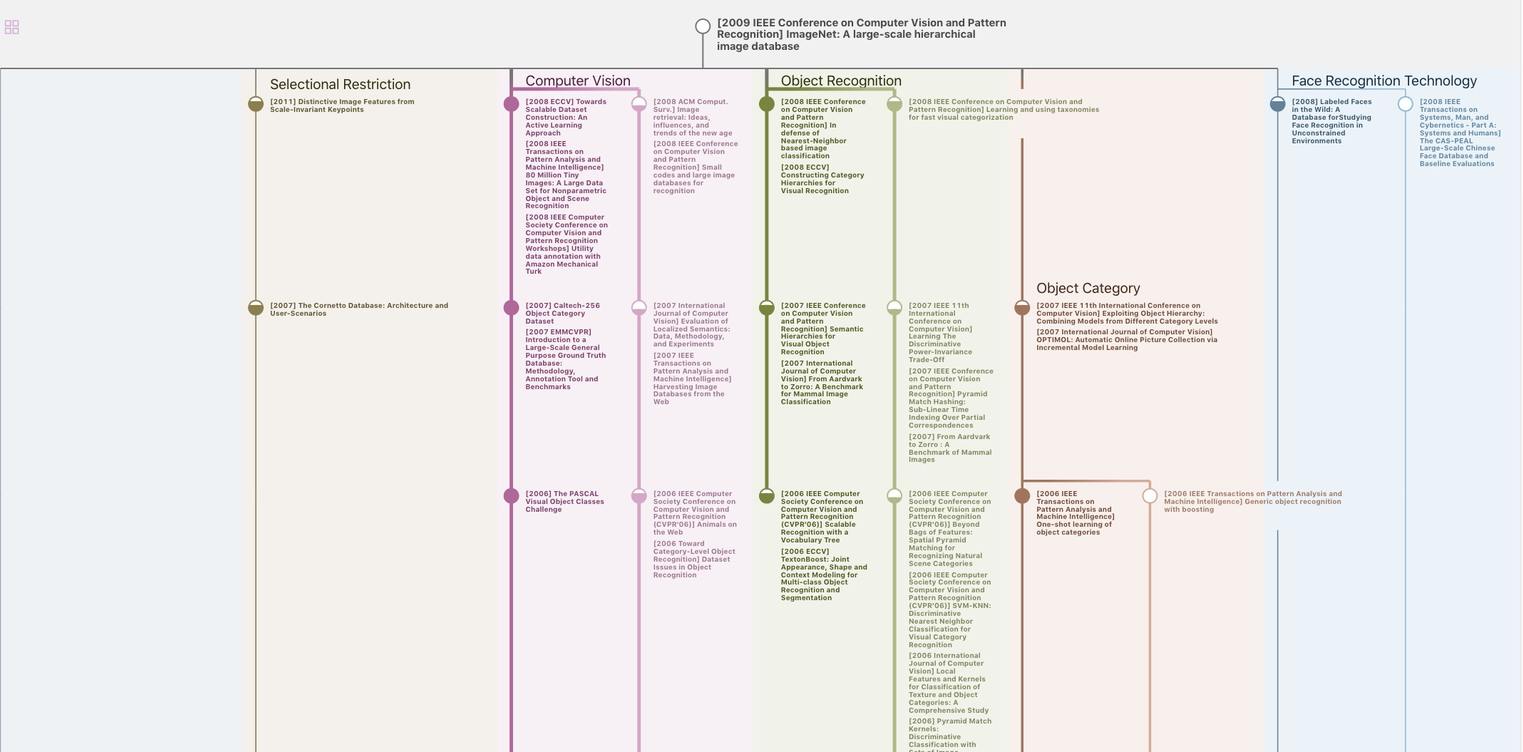
生成溯源树,研究论文发展脉络
Chat Paper
正在生成论文摘要