Improved Multi-Objective Data Stream Clustering with Time and Memory Optimization
PROCEEDINGS OF THE 2023 GENETIC AND EVOLUTIONARY COMPUTATION CONFERENCE COMPANION, GECCO 2023 COMPANION(2023)
摘要
Data stream clustering is essential in machine learning and big data analytics. However, clustering this type of data requires some restrictions in time and memory. This paper proposes a multi-objective data stream clustering algorithm (IMOC-Stream) that addresses these challenges by incorporating time and memory optimization into the clustering process. The goal of IMOC-Stream is to 1) reduce computation time by using idle times to apply genetic operations and enhance the solution. 2) reduce memory allocation by introducing a new tree synopsis. 3) find arbitrarily shaped clusters by using a multi-objective framework. We conducted an experimental study with high-dimensional stream datasets and compared them to well-known stream clustering techniques. The experiments show the ability of our method to partition the data stream into arbitrarily shaped, compact, and well-separated clusters while optimizing time and memory. Our approach also outperformed most of the other stream algorithms.
更多查看译文
关键词
Multi-objective clustering,AntTree algorithm,Data Stream,Evolutionary clustering
AI 理解论文
溯源树
样例
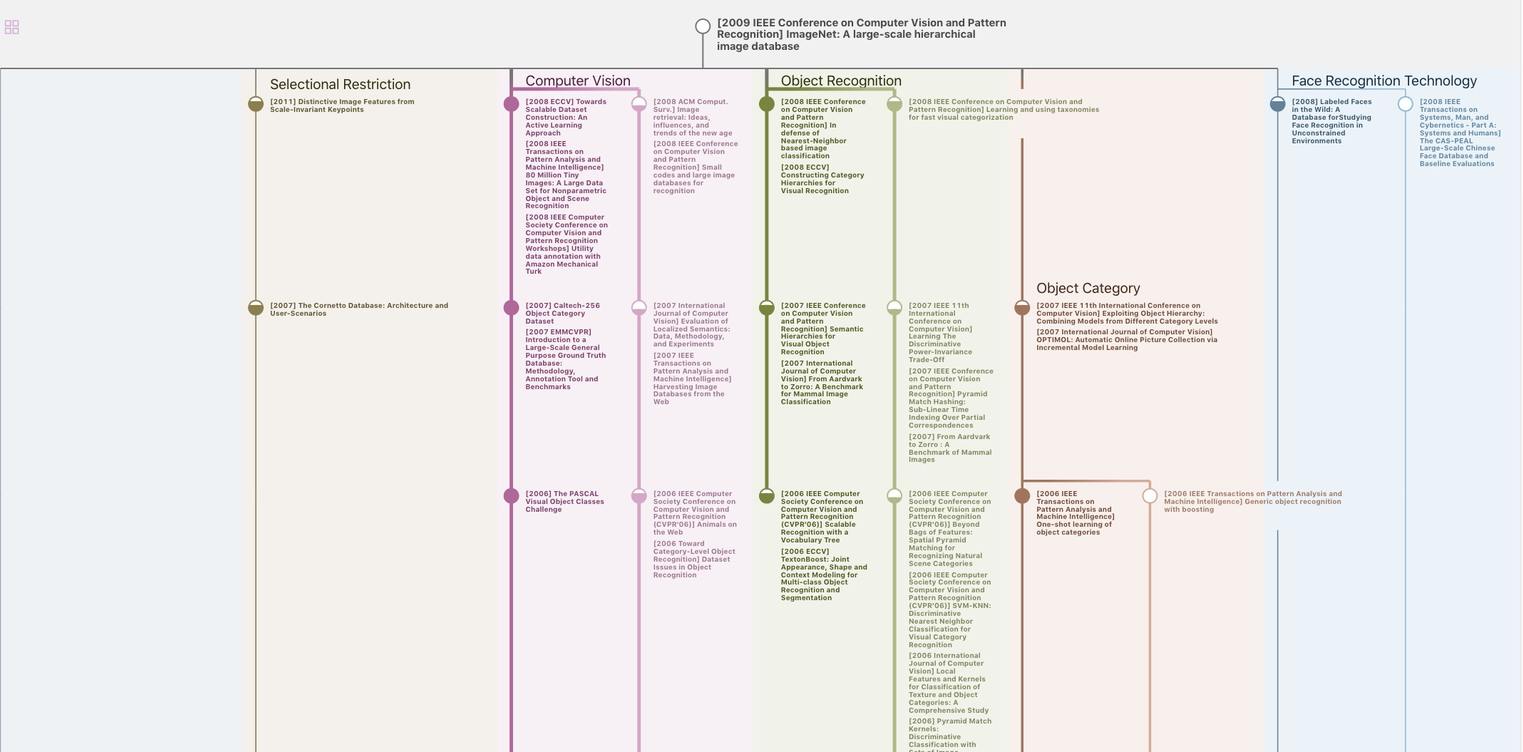
生成溯源树,研究论文发展脉络
Chat Paper
正在生成论文摘要