Patient-GAT: Sarcopenia Prediction using Multi-modal Data Fusion andWeighted Graph Attention Networks
38TH ANNUAL ACM SYMPOSIUM ON APPLIED COMPUTING, SAC 2023(2023)
摘要
Graph Attention Networks (GAT) have been extensively used to perform node-level classification on data that can be represented as a graph. However, few papers have investigated the effectiveness of using GAT on graph representations of patient similarity networks. This paper proposes Patient-GAT, a novel method to predict chronic health conditions by first integrating multi-modal data fusion to generate patient vector representations using imputed lab variables with other structured data. This data representation is then used to construct a patient network by measuring patient similarity, finally applying GAT to the patient network for disease prediction. We demonstrated our framework by predicting sarcopenia using real-world EHRs obtained from the Indiana Network for Patient Care. We evaluated the performance of our system by comparing it to other baseline models, showing that our model outperforms other methods. In addition, we studied the contribution of the temporal representation of the lab data and discussed the interpretability of this model by analyzing the attention coefficients of the trained Patient-GAT model. Our code can be found on Github(1)
更多查看译文
关键词
Electronic Health Records,Graph Neural Networks,Data Fusion,Prediction,Model Interpretation
AI 理解论文
溯源树
样例
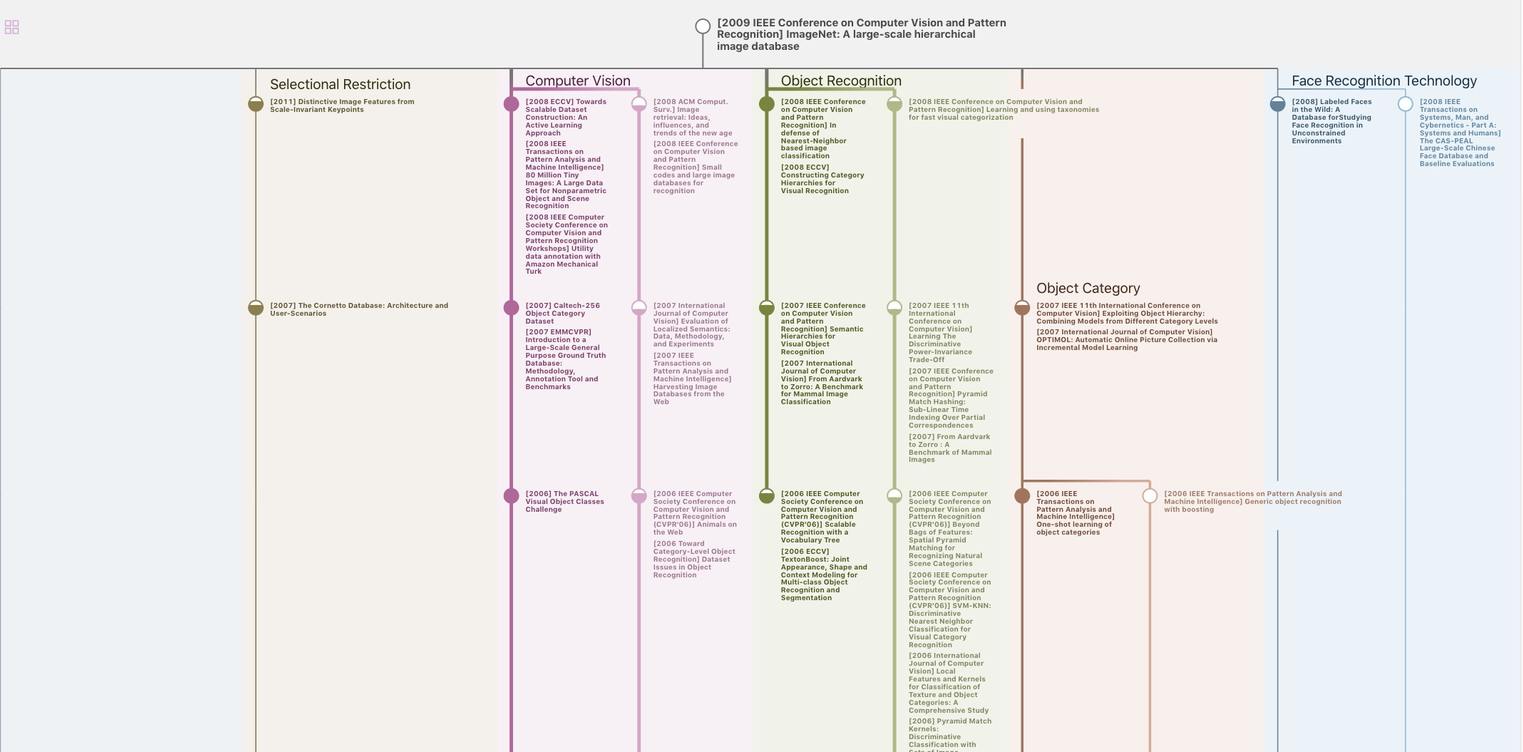
生成溯源树,研究论文发展脉络
Chat Paper
正在生成论文摘要