MGR3Net: Multigranularity Region Relation Representation Network for Facial Expression Recognition in Affective Robots
IEEE TRANSACTIONS ON INDUSTRIAL INFORMATICS(2024)
摘要
Automatic facial expression recognition (FER) based on face images is essential for affective robots, which are designed for interactive companions and intelligent healthcare. Although existing DL-based FERs have made significant progress, an accurate FER model in robots is challenging due to the subtle differences in facial expressions across various scenarios. To address this issue, we propose a multigranularity region relation representation network (MGR(3)Net) to improve the robustness and generalization of FER via attention-guided global-local fusion. The MGR(3)Net is composed of three modules: multigranularity attention (MGA), holistic-regional feature extractor (HRFE), and hybrid feature fusion. In the MGA module, we first process each holistic cropped face image into three granularity of face regions from coarse to fine, which are four region-cropped faces, 2(2) face partitions, and 4(2) face partitions. Then, we propose the region attention relation cell to model the relationship between each region and the aggregated representation while preserving the spatial information of the local features. In the HRFE module, we align multigranularity features from the coarse space to the finer space and extract one holistic embedding and multiple region embeddings for each granularity. Finally, we use a hybrid-level fusion strategy to combine global-local features from the three granularities for final classification. Extensive experiments demonstrate that the MGR(3)Net outperforms the state-of-the-art methods evaluated on the in-the-lab datasets, in-the-wild datasets, and occlusion/pose-based sets.
更多查看译文
关键词
Affective robot,coarse-to-fine region partition,facial expression recognition (FER),hybrid feature fusion (HFF),multigranularity,region relation representation
AI 理解论文
溯源树
样例
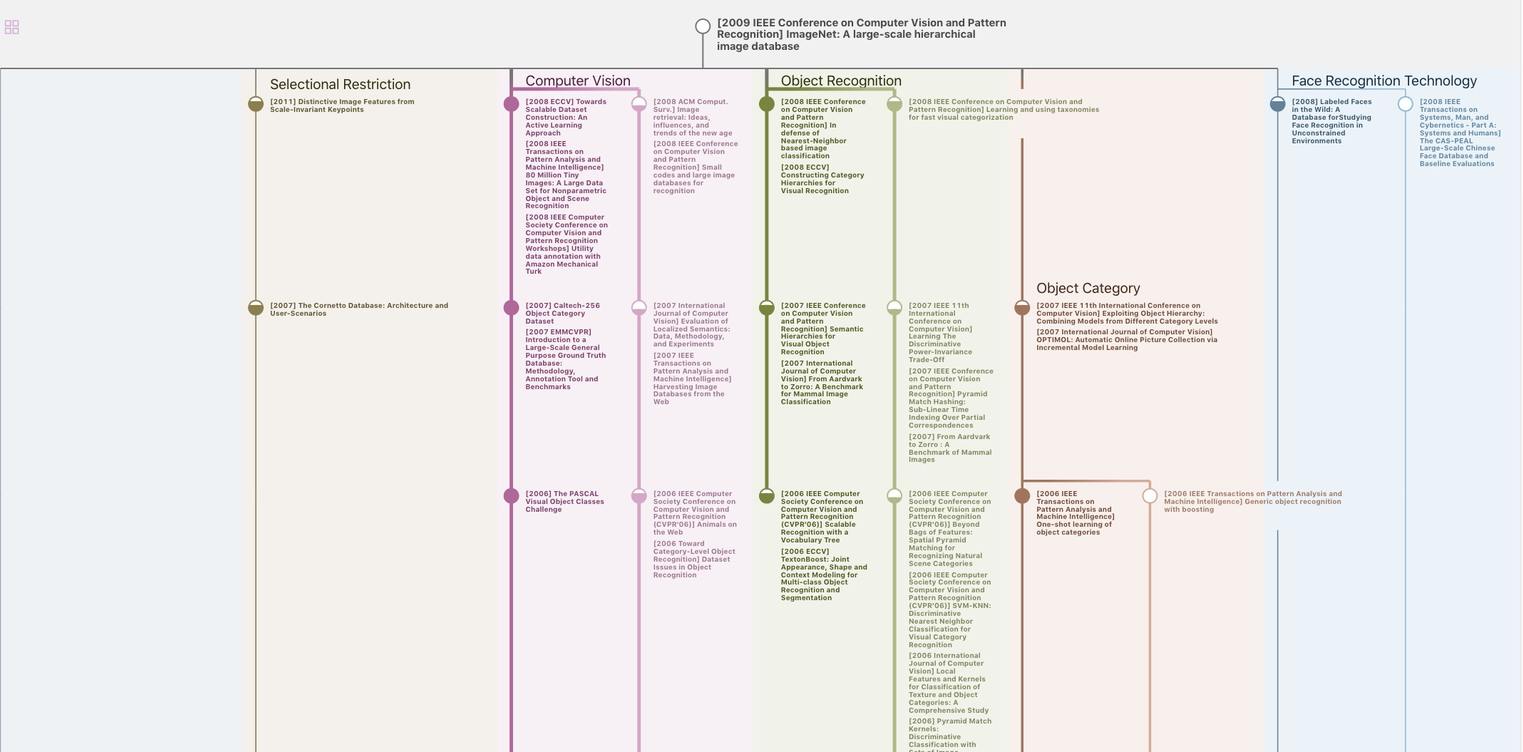
生成溯源树,研究论文发展脉络
Chat Paper
正在生成论文摘要