Copula-based pairwise estimator for quantile regression with hierarchical missing data
STATISTICAL MODELLING(2024)
摘要
Quantile regression can be a helpful technique for analysing clustered (such as longitudinal) data. It can characterize the change in response over time without making distributional assumptions and is robust to outliers in the response. A quantile regression model using a copula-based multivariate asymmetric Laplace distribution for addressing correlation due to clustering is introduced. Furthermore, we propose a pairwise estimator for the parameters of the model. Since it is based on pseudo-likelihood, it needs to be modified to avoid bias in presence of missingness. Therefore, we enhance the model with inverse probability weighting. In this way, our proposal is unbiased under the missing at random assumption. Based on simulations, the estimator is efficient and computationally fast. Finally, the methodology is illustrated using a study in ophthalmology.
更多查看译文
关键词
asymmetric Laplace distribution,copulas,inverse probability weighting,quantile regression,longitudinal data,missing data,pairwise estimator
AI 理解论文
溯源树
样例
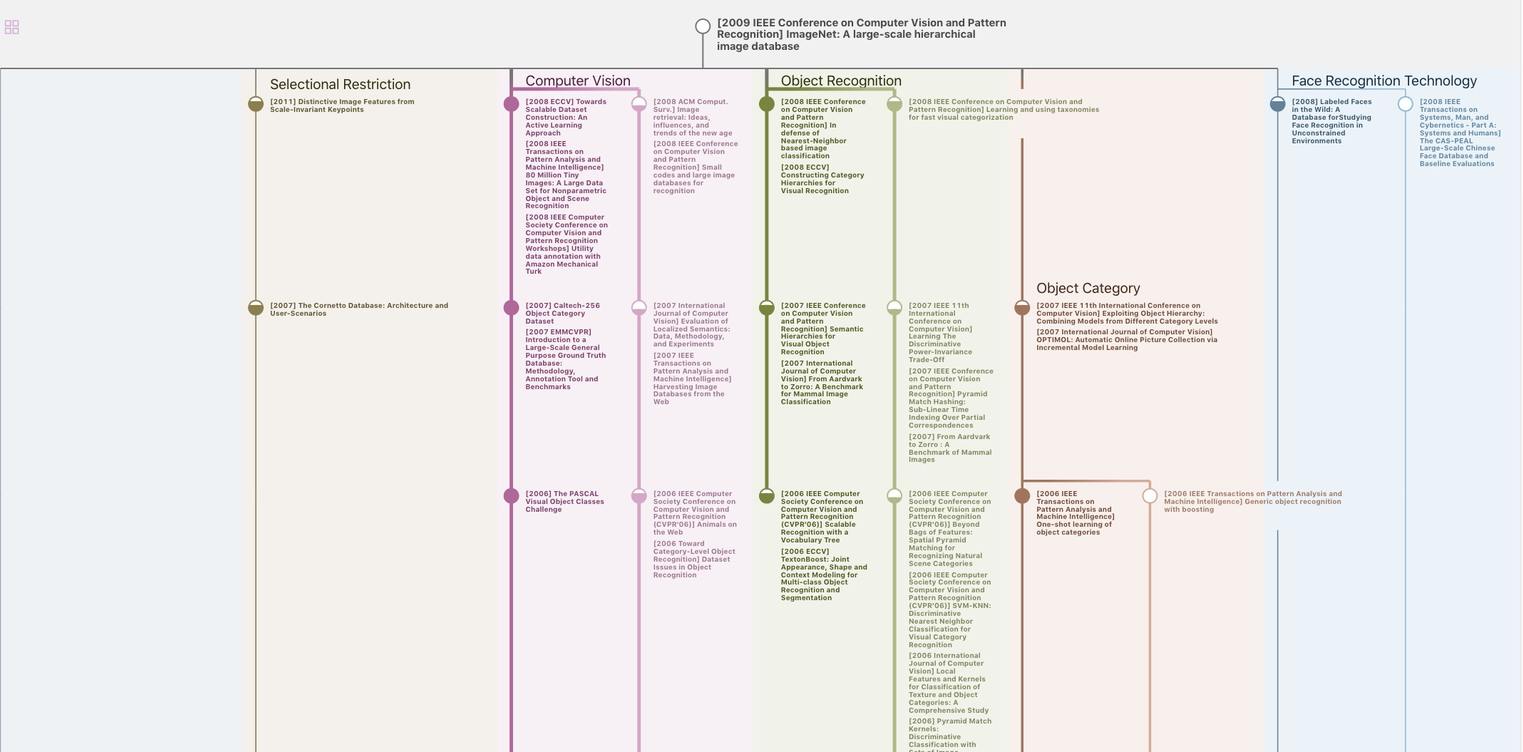
生成溯源树,研究论文发展脉络
Chat Paper
正在生成论文摘要