Quantifying the Relative Contributions of the Global Oceans to ENSO Predictability With Deep Learning
GEOPHYSICAL RESEARCH LETTERS(2024)
摘要
We propose a unified statistical method based on deep learning and analysis to quantify the relative contributions of the global oceans to El Nino-Southern Oscillation (ENSO) predictability. By incorporating subsurface signals in the Indian Ocean and Atlantic, the forecast lead can be skillfully extended by about one season. This skill enhancement mainly originates from the tropical Indian Ocean, presumably related to signals of the Indian Ocean Dipole passing to the tropical Pacific through the Indonesian Throughflow. The sea surface temperature anomaly (SSTA) in the Indian Ocean accounts for nearly 50% of surface contributions to both El Nino and La Nina predictions at a 15-month lead. The north tropical Atlantic SSTA has a moderate impact on La Nina at a 9-month lead. The Pacific Meridional Mode plays a significant role in both ENSO phases at a 12-month lead. Thus, our study suggests that trans-basin effects for ENSO are more vigorous than previously thought. El Nino-Southern Oscillation (ENSO) can excite various modes of climate variability in the Indian Ocean, Atlantic, and extratropical Pacific. However, less understood and quantified than the ENSO-driven influences is how these regions outside the tropical Pacific in turn affect ENSO. Here, we quantify the impacts of the Indian Ocean, Atlantic, and extratropical Pacific on the predictability of ENSO. The lead time can be skillfully extended by one season by incorporating subsurface signals from the Indian and Atlantic oceans. The extratropical Pacific SST can indeed provide some useful sources of information for ENSO predictability at long lead times. A heatmap analysis further indicates that the triggering precursors freely selected by the deep learning model link to our current understanding of physical pathways for ENSO prediction. The Indian Ocean Dipole, the north tropical Atlantic SSTA, and the Pacific Meridional Mode are the main trans-basin factors that vigorously influence ENSO at different lead times. Our findings emphasize that the impacts of the global oceans on ENSO are significant, and their influences should be properly considered in the coupled models. Heat content in the Indian Ocean can provide extra signals for long-term El Nino-Southern Oscillation (ENSO) forecasts and extend the lead time by about one season The Indian Ocean Dipole can significantly influence El Nino via both surface and subsurface physical pathways at a 15-month lead The north tropical Atlantic warming and the Pacific Meridional Mode also vigorously affect ENSO at lead times of both 12 and 9 months
更多查看译文
关键词
ENSO prediction,trans-basin effects for ENSO,deep learning
AI 理解论文
溯源树
样例
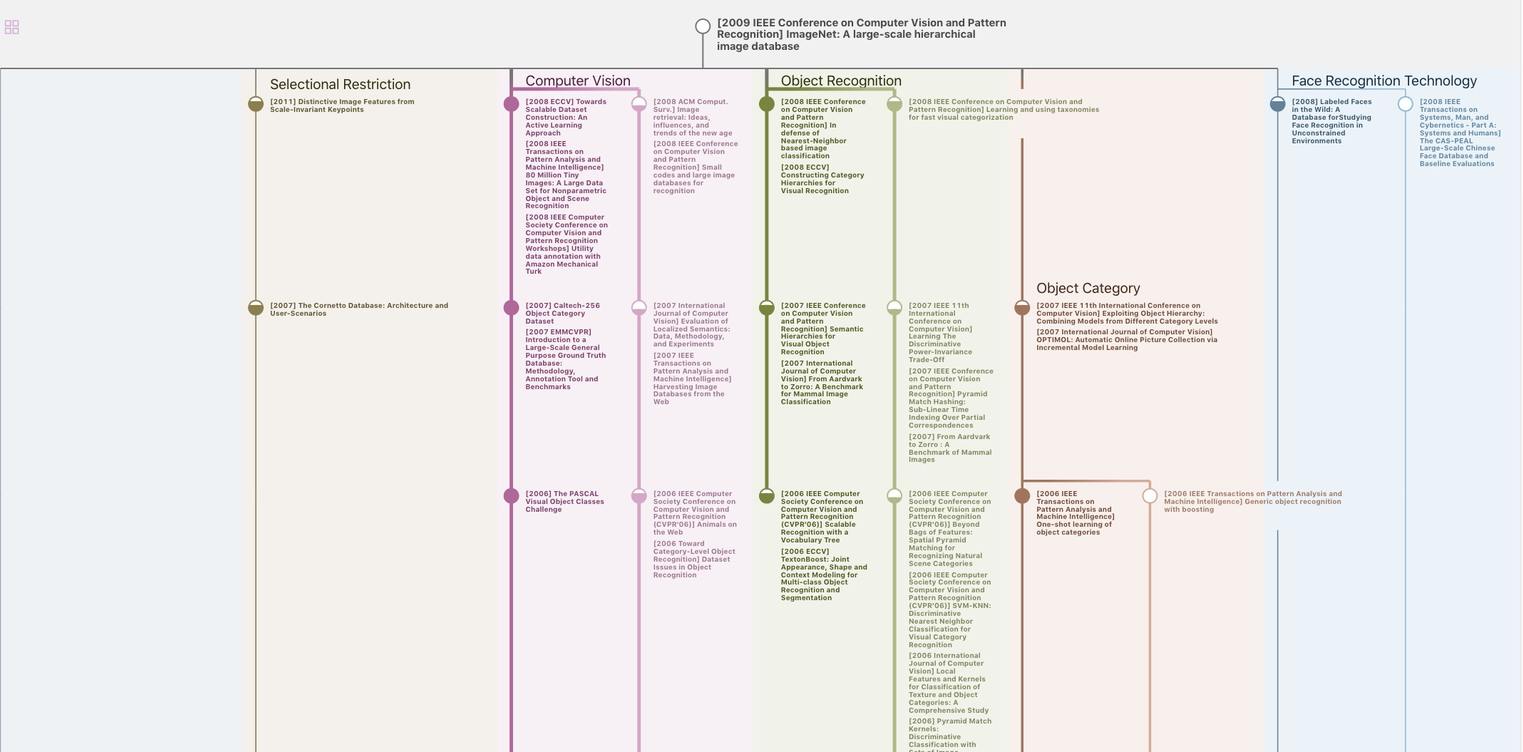
生成溯源树,研究论文发展脉络
Chat Paper
正在生成论文摘要