Multi-Identity Recognition of Darknet Vendors Based on Metric Learning
APPLIED SCIENCES-BASEL(2024)
摘要
Dark web vendor identification can be seen as an authorship aliasing problem, aiming to determine whether different accounts on different markets belong to the same real-world vendor, in order to locate cybercriminals involved in dark web market transactions. Existing open-source datasets for dark web marketplaces are outdated and cannot simulate real-world situations, while data labeling methods are difficult and suffer from issues such as inaccurate labeling and limited cross-market research. The problem of identifying vendors' multiple identities on the dark web involves a large number of categories and a limited number of samples, making it difficult to use traditional multiclass classification models. To address these issues, this paper proposes a metric learning-based method for dark web vendor identification, collecting product data from 21 currently active English dark web marketplaces and using a multi-dimensional feature extraction method based on product titles, descriptions, and images. Using pseudo-labeling technology combined with manual labeling improves data labeling accuracy compared to previous labeling methods. The proposed method uses a Siamese neural network with metric learning to learn the similarity between vendors and achieve the recognition of vendors' multiple identities. This method achieved better performance with an average F1-score of 0.889 and an accuracy rate of 97.535% on the constructed dataset. The contributions of this paper lie in the proposed method for collecting and labeling data for dark web marketplaces and overcoming the limitations of traditional multiclass classifiers to achieve effective recognition of vendors' multiple identities.
更多查看译文
关键词
darknet,data mining,multi-identity recognition,metric learning,Siamese neural networks
AI 理解论文
溯源树
样例
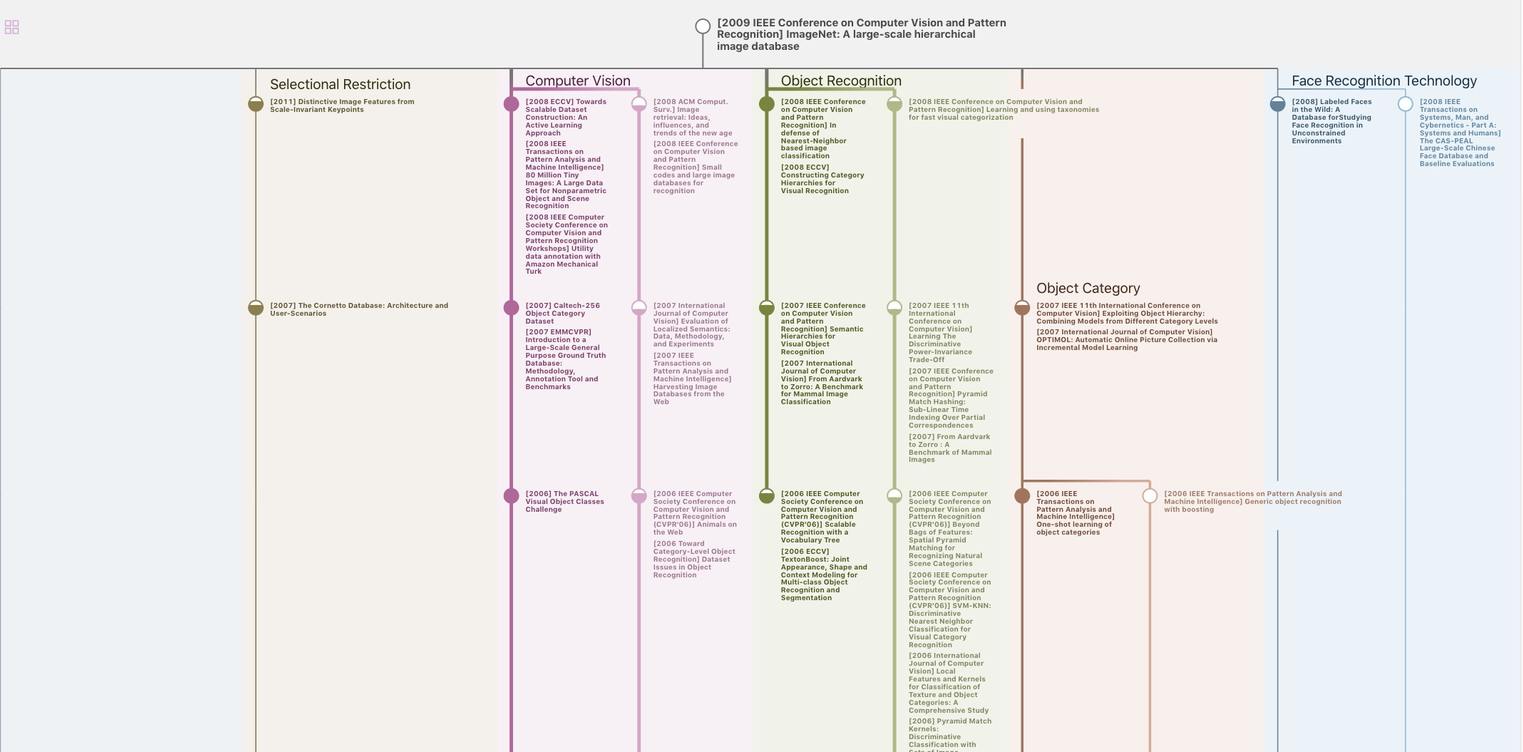
生成溯源树,研究论文发展脉络
Chat Paper
正在生成论文摘要