Class-SpecificWord Sense Aware Topic Modeling via Soft Orthogonalized Topics
PROCEEDINGS OF THE 32ND ACM INTERNATIONAL CONFERENCE ON INFORMATION AND KNOWLEDGE MANAGEMENT, CIKM 2023(2023)
摘要
We propose a word sense aware topic model for document classification based on soft orthogonalized topics. An essential problem for this task is to capture word senses related to classes, i.e., class-specific word senses. Traditional models mainly introduce semantic information of knowledge libraries for word sense discovery. However, this information may not align with the classification targets, because these targets are often subjective and task-related. We aim to model the class-specific word senses in topic space. The challenge is to optimize the class separability of the senses, i.e., obtaining sense vectors with (a) high intra-class and (b) low inter-class similarities. Most existing models predefine specific topics for each class to specify the class-specific sense vectors. We call them hard orthogonalization based methods. These methods can hardly achieve both (a) and (b) since they assume the conditional independence of topics to classes and inevitably lose topic information. To this problem, we propose soft orthogonalization for topics. Specifically, we reserve all the topics and introduce a group of class-specific weights for each word to handle the importance of topic dimensions to class separability. Besides, we detect and use highly class-specific words in each document to guide sense estimation. Our experiments on two standard datasets show that our proposal outperforms other state-of-the-art models in terms of accuracy of sense estimation, document classification, and topic modeling. In addition, our joint learning experiments with the pre-trained language model BERT showcased the best complementarity of our model in most cases compared to other topic models.
更多查看译文
关键词
document representation,topic model,graphical model,word sense disambiguation,document classification
AI 理解论文
溯源树
样例
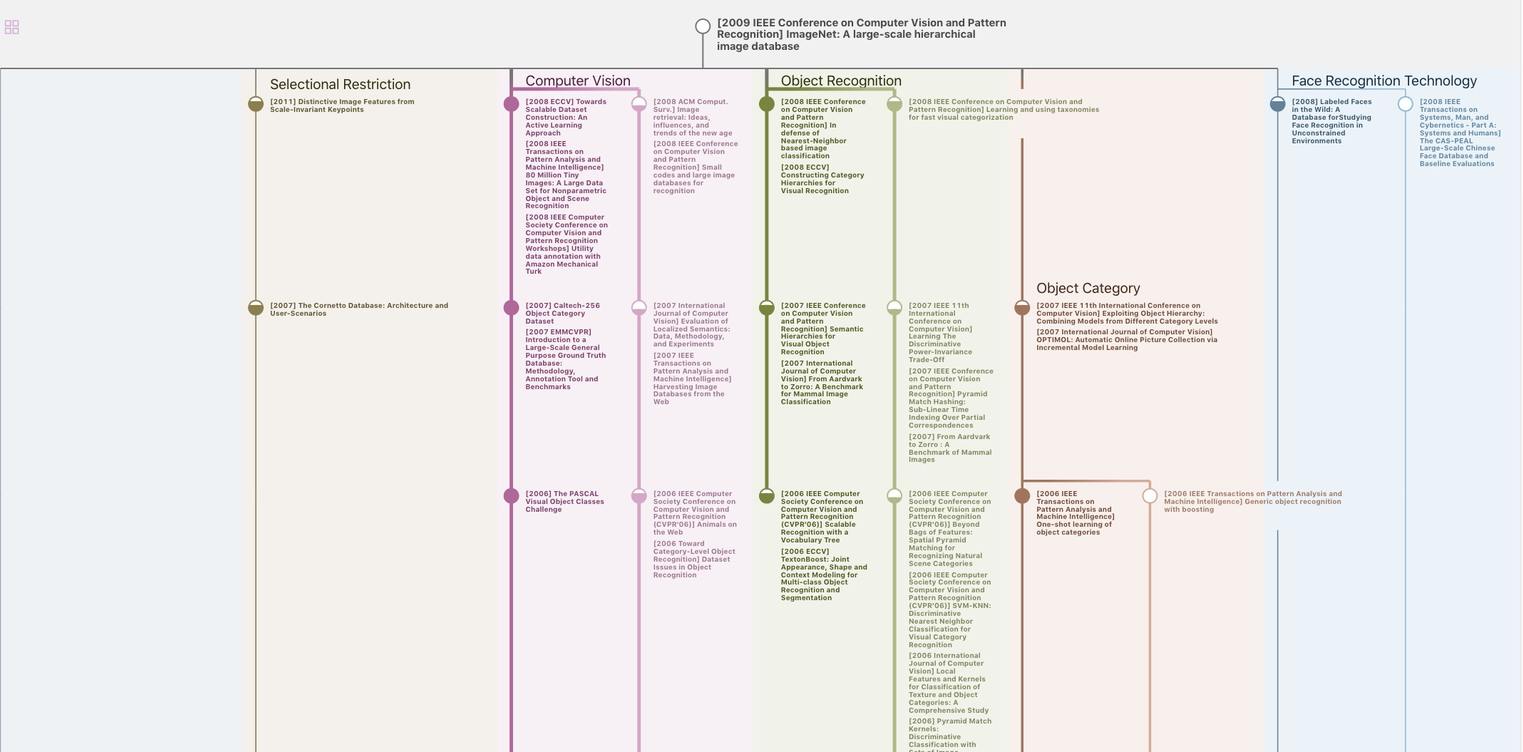
生成溯源树,研究论文发展脉络
Chat Paper
正在生成论文摘要