James ate 5 oranges = Steve bought 5 pencils: Structure-Aware Denoising for ParaphrasingWord Problems
PROCEEDINGS OF THE 32ND ACM INTERNATIONAL CONFERENCE ON INFORMATION AND KNOWLEDGE MANAGEMENT, CIKM 2023(2023)
摘要
We propose SCANING, an unsupervised framework for paraphrasing via controlled noise injection. We focus on the novel task of paraphrasing algebraic word problems having practical applications in online pedagogy as a means to reduce plagiarism as well as evoke reasoning capabilities on the part of the student instead of rote memorization. This task is more complex than paraphrasing general-domain corpora due to the difficulty in preserving critical information for solution consistency of the paraphrased word problem, managing the increased length of the text and ensuring diversity in the generated paraphrase. Existing approaches fail to demonstrate adequate performance on at least one, if not all, of these facets, necessitating the need for a more comprehensive solution. To this end, we model the noising search space as a composition of contextual and syntactic aspects to sample noising functions. This allows for learning a denoising function, that operates over both aspects and produces semantically equivalent and syntactically diverse outputs through grounded noise injection. The denoising function serves as a foundation for training a paraphrasing function, which operates solely in the input-paraphrase space without carrying any direct dependency on noise. We demonstrate that SCANING improves performance in terms of producing semantically equivalent and syntactically diverse paraphrases by 35% through extensive automated and human evaluation across 4 datasets.
更多查看译文
关键词
paraphrasing,self-supervised,denoising,neural networks,mwp,education
AI 理解论文
溯源树
样例
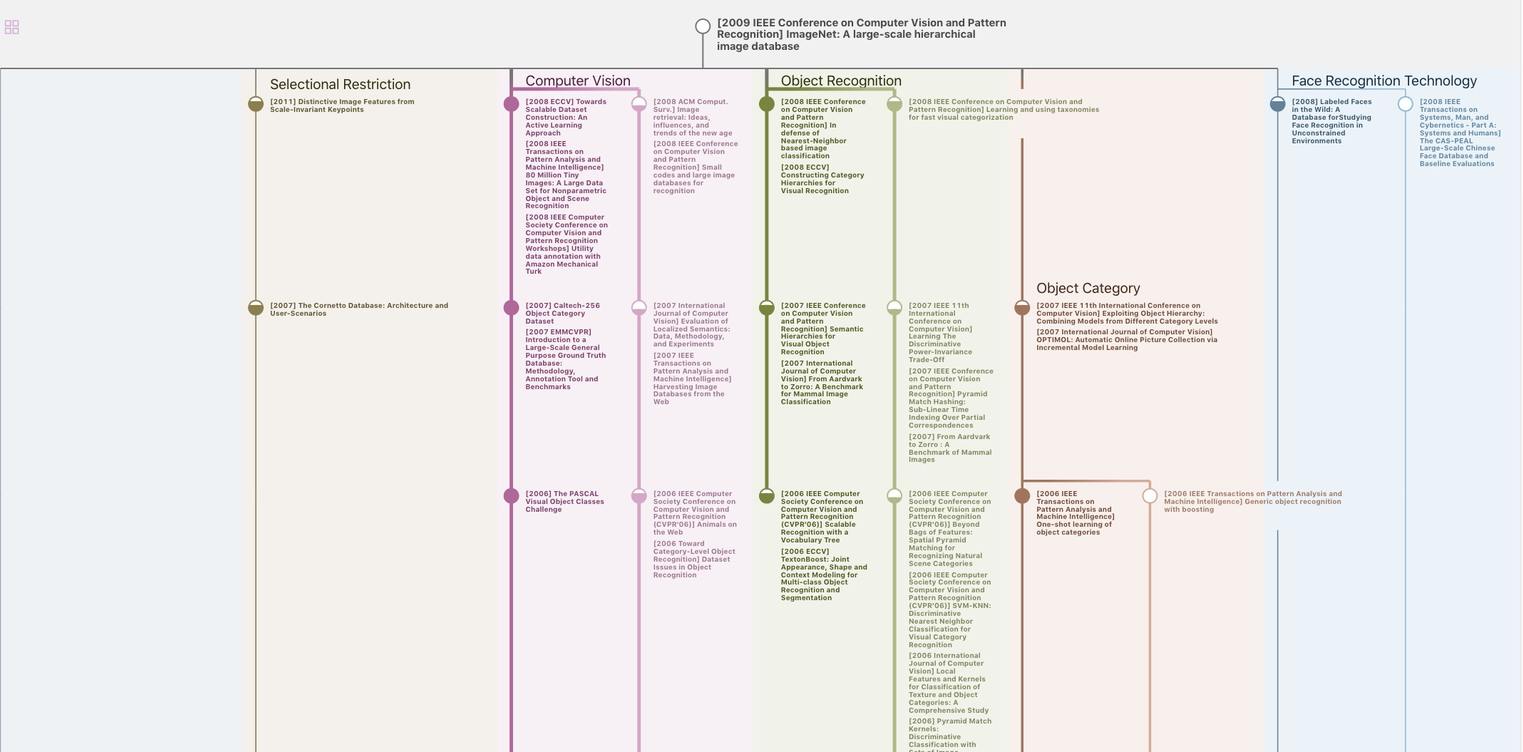
生成溯源树,研究论文发展脉络
Chat Paper
正在生成论文摘要