Regional landslide mapping model developed by a deep transfer learning framework using post-event optical imagery
GEORISK-ASSESSMENT AND MANAGEMENT OF RISK FOR ENGINEERED SYSTEMS AND GEOHAZARDS(2024)
摘要
Landslides are major natural disasters in mountainous areas, often caused by earthquakes and heavy rainfalls. Traditional manual delineation methods for identifying landslide features using optical imagery are inefficient, highlighting the need for automated detection techniques. Deep Convolutional Neural Networks (CNNs) have emerged as advanced solutions in computer vision for this purpose. Despite the reliance on pre-event and post-event imagery or various data sources like digital elevation models (DEMs), the success of deep learning models largely depends on the quality and availability of training data. This poses a challenge for their immediate application after a landslide. This study explores the transferability of a CNN model trained on data from the 2016 Kumamoto Earthquakes for detecting landslides in different events, specifically the 2018 Hokkaido earthquake and the 2017 Asakura Rainfall in Japan. These cases were chosen for their geographical similarities. The proposed deep transfer learning model, based on a DeepLabV3 + architecture built on a pre-trained ResNet50, automatically identifies landslide features without needing specific training data or model adjustments for each event. It achieved high accuracy in both cases, demonstrating CNNs' potential for broad application in landslide detection and enhancing disaster response efforts.
更多查看译文
关键词
Deep transfer learning,regional landslide mapping,cross-event transferability,semantic image segmentation,data augmentation
AI 理解论文
溯源树
样例
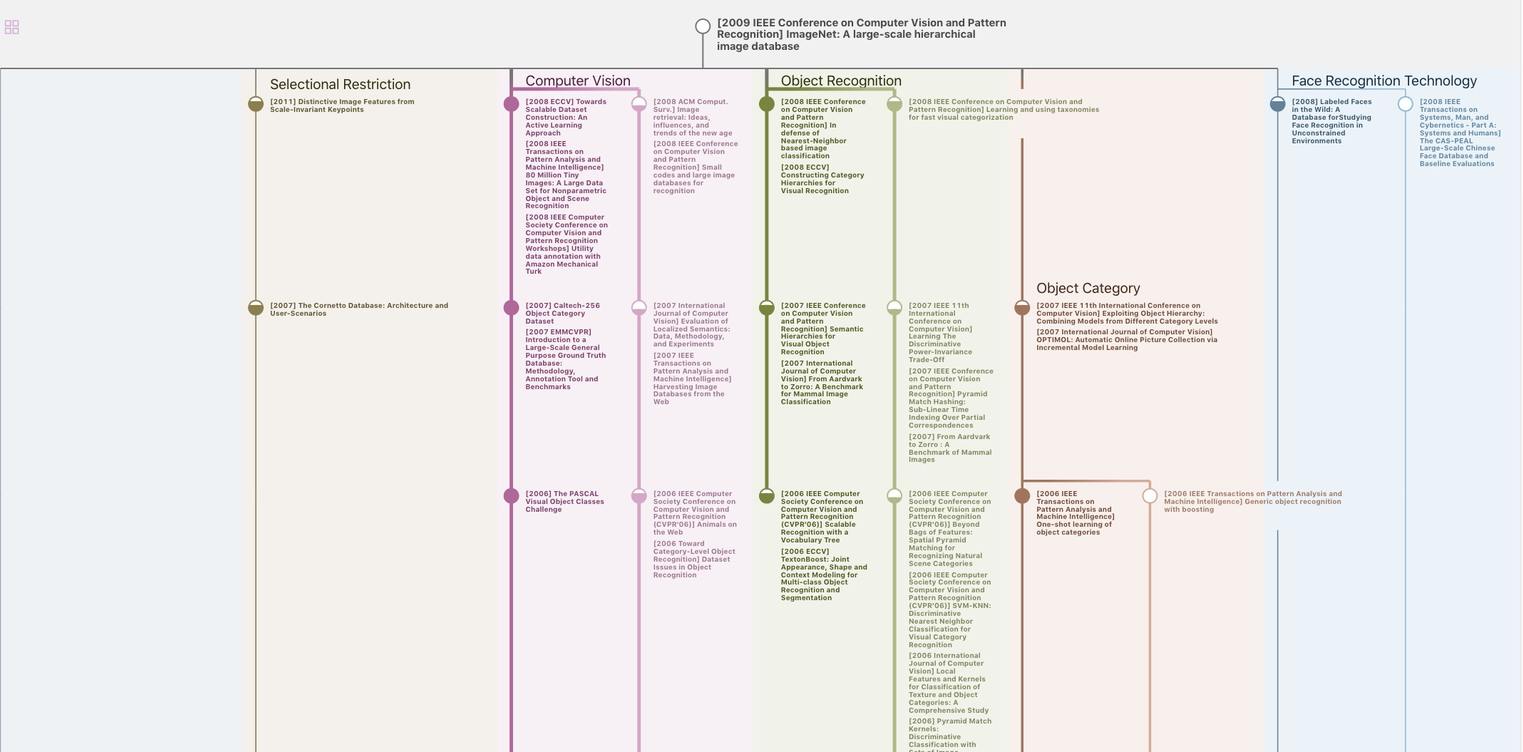
生成溯源树,研究论文发展脉络
Chat Paper
正在生成论文摘要