County-Level Evaluation of Large-Scale Gridded Data Sets of Irrigated Area Over China
JOURNAL OF GEOPHYSICAL RESEARCH-ATMOSPHERES(2024)
摘要
The reliability of irrigated area (IA) information dominates the performance of irrigation water use and crop modeling accuracy. IA is typically mapped using Food and Agriculture Organization (FAO) agricultural census and remote sensing indices. Recent advances in machine learning and sampling techniques further improve IA mapping. However, the relative performances of different IA mapping approaches and their capability in capturing long-term IA temporal variability remain unknown. Here, 1861 county-level IA information from Government Censored Data (GCD) during 2000-2021 are collected, cross-validated, and employed to evaluate commonly used gridded IA data sets. Results show that IA data sets based on the direct interpolation of FAO agricultural census can accurately capture the spatial distribution of IA. However, FAO statistics are only available in a particular year, which cannot capture inter-annual irrigation variations. In contrast, IA products solely based on vegetation indices are prone to positive biases over humid regions due to the lack of contrast in vegetation dynamics. Overall, the latest GCD-based machine learning IA data sets are relatively more accurate, but they are also problematic in estimating IA trends due to the use of temporally static training samples. Such biases are tightly related to agricultural suitability (AS calculated using precipitation and potential evapotranspiration). This suggests that AS should be employed as an endogenous variable in future machine learning based IA mapping algorithms. Understanding how much land is irrigated (Irrigated Area or IA) is crucial for accurate water use and crop modeling. Traditional IA maps are developed using data from the Food and Agriculture Organization (FAO) and remote sensing indices. Recent improvements, like machine learning, have enhanced IA mapping. However, the relative performances of these methods and their reliability in capturing long-term changes in irrigated areas remain unknown. This study collects government-censored county-level IA data from 2000 to 2021, which is subsequently used for evaluating commonly used IA data sets. Results show that data sets directly using FAO data can reasonability capture the spatial distribution of IA but may miss year-to-year changes. On the other hand, data sets relying only on vegetation data tend to overestimate irrigated areas in humid regions. The latest machine learning-based IA data sets are relatively more accurate but still contain significant uncertainties in IA trends. These biases are closely linked to agricultural suitability (calculated using precipitation and potential evapotranspiration), which may be useful for enhancing future IA mapping algorithms. Remote sensing vegetation index is insufficient for identifying irrigated area over humid regions Machine learning based irrigated area is sensitive to the quality of training sample All the irrigated area data sets are biased in representing the temporal dynamics of irrigated area
更多查看译文
关键词
irrigated area,agricultural suitability,irrigation modeling,machine learning,error evaluation
AI 理解论文
溯源树
样例
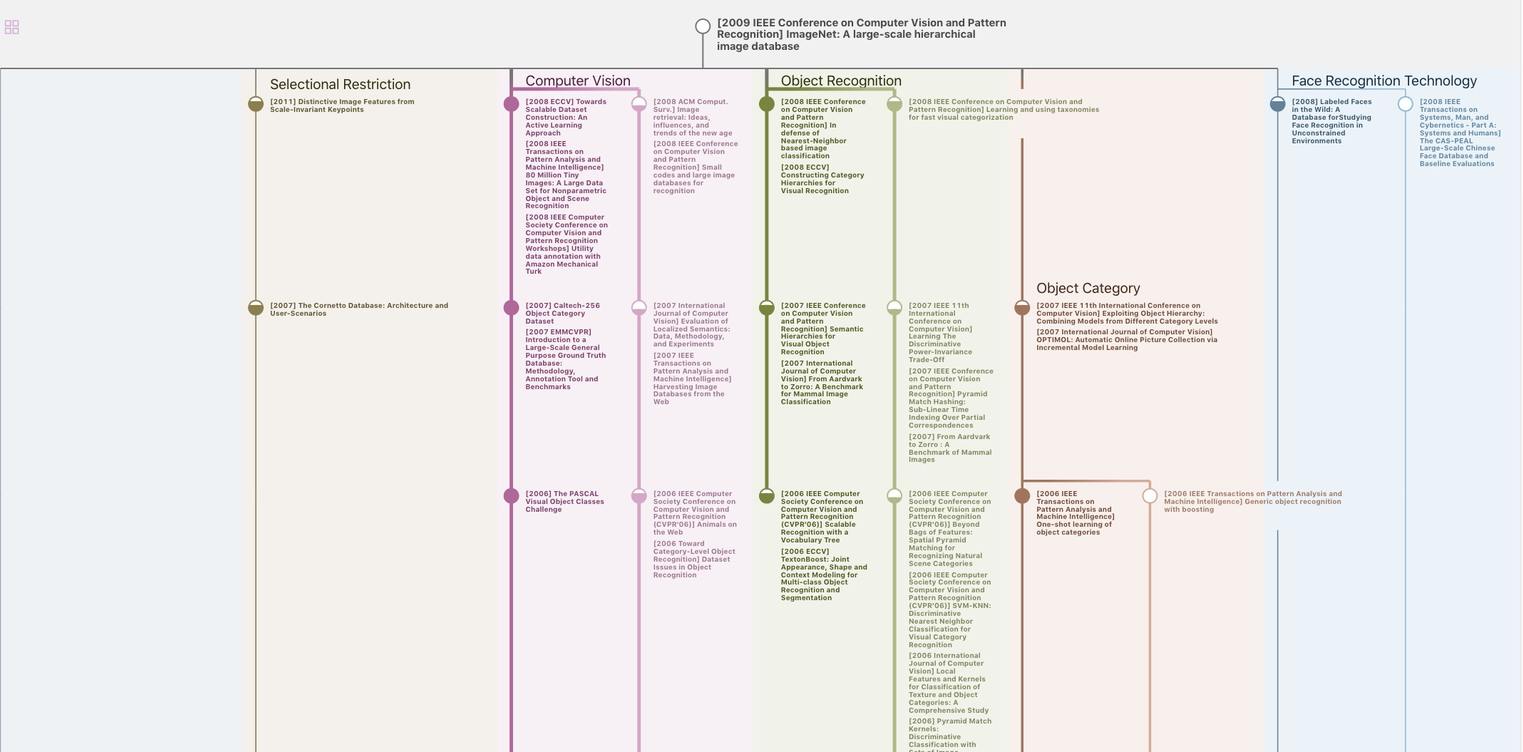
生成溯源树,研究论文发展脉络
Chat Paper
正在生成论文摘要