Cardiopulmonary Exercise Testing Parameters-Based Phenogroups of Patients with Heart Failure with Reduced Ejection Fraction Using an Unsupervised Machine Learning Algorithm
CIRCULATION(2023)
摘要
Introduction: Although cardiopulmonary exercise testing (CPET) is the standard test for functional capacity and risk stratification in patients with HFrEF, the integrative use of different CPET parameters for prognostication is warranted. Hypothesis: A differential prognostication of HFrEF phenogroups can be achieved by means of an unsupervised machine learning algorithm of CPET parameters. Methods: We reviewed medical records of 1,067 patients with HFrEF (EF ≤ 40%) undergoing CPET from 12/2012 to 9/2020. Patients with hypotensive or bradycardic response during exercise were excluded. Unsupervised machine learning using partition around medoids (PAM) algorithm was used to create clusters based on CPET parameters. Primary outcome was LVAD/heart transplant-free survival. Kaplan-Meier plots along with log-rank test and multivariable Cox regression analysis with adjustment for co-morbidities, age, smoking, and LVEF were used to compare prognosis across clusters. Results: Among 935 patients with HFrEF included in the algorithm (mean age 56±12 years, EF 24±8%, 73% men, and 73% with RER > 1.05), 329 (35 %) patients had adverse events (median follow up time 964 days). Using the PAM algorithm, we created 3 clusters using CPET parameters. Median (IQR) of age (53 [43-62], 58 [50-64] and 61 [54-68] years for cluster 1,2, and 3, respectively) and LVEF (30 [22-25], 25 [19-30] and 21 [16-26] % for cluster 1,2, and 3, respectively) were significantly different ( P <0.001). No differences in sex of BMI were observed. Co-morbidities burden was higher in cluster 2 and 3, compared to cluster 1 ( Figure 1A) . Compared to cluster 1, cluster 2 and 3 had worse LVAD/heart transplant-free survival (adjusted HR 2.15 [95% CI 1.46-3.17] and 5.68 [95% CI 3.89-8.30], respectively; Figure 1B ). Conclusions: Patients with HFrEF could be risk-stratified into low-, medium- and high-risk using PAM algorithm solely based on CPET parameters that tracked with increasing comorbidity burden.
更多查看译文
关键词
Heart Failure,Machine Learning,Cardiopulmonary exercise testing,Prognosis
AI 理解论文
溯源树
样例
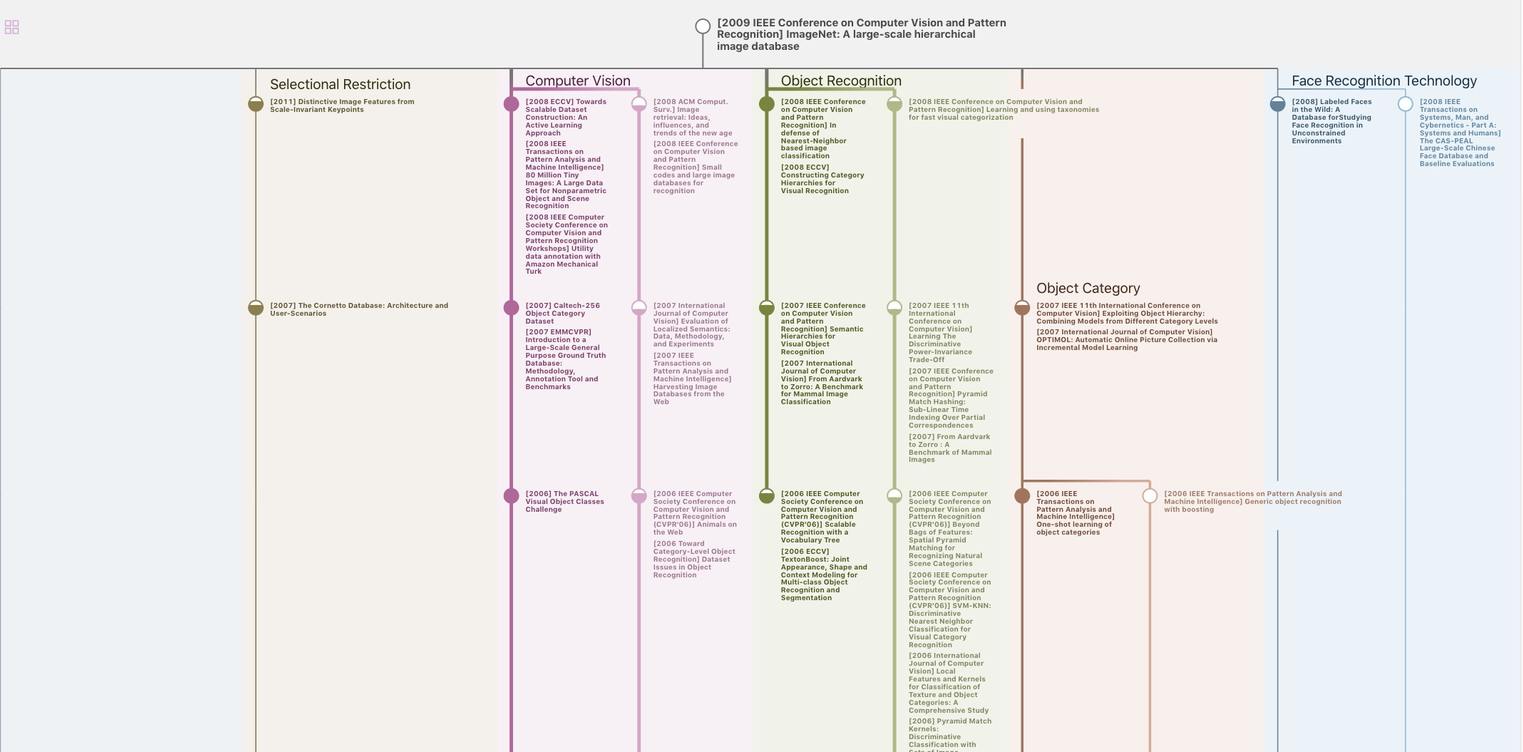
生成溯源树,研究论文发展脉络
Chat Paper
正在生成论文摘要