IMPROVE: a feature model to predict neoepitope immunogenicity through broad-scale validation of T-cell recognition
FRONTIERS IN IMMUNOLOGY(2024)
摘要
Background Mutation-derived neoantigens are critical targets for tumor rejection in cancer immunotherapy, and better tools for neoepitope identification and prediction are needed to improve neoepitope targeting strategies. Computational tools have enabled the identification of patient-specific neoantigen candidates from sequencing data, but limited data availability has hindered their capacity to predict which of the many neoepitopes will most likely give rise to T cell recognition.Method To address this, we make use of experimentally validated T cell recognition towards 17,500 neoepitope candidates, with 467 being T cell recognized, across 70 cancer patients undergoing immunotherapy.Results We evaluated 27 neoepitope characteristics, and created a random forest model, IMPROVE, to predict neoepitope immunogenicity. The presence of hydrophobic and aromatic residues in the peptide binding core were the most important features for predicting neoepitope immunogenicity.Conclusion Overall, IMPROVE was found to significantly advance the identification of neoepitopes compared to other current methods.
更多查看译文
关键词
neoantigen,neoepitope prediction,machine learning,immunotherapy,immunoinformatics
AI 理解论文
溯源树
样例
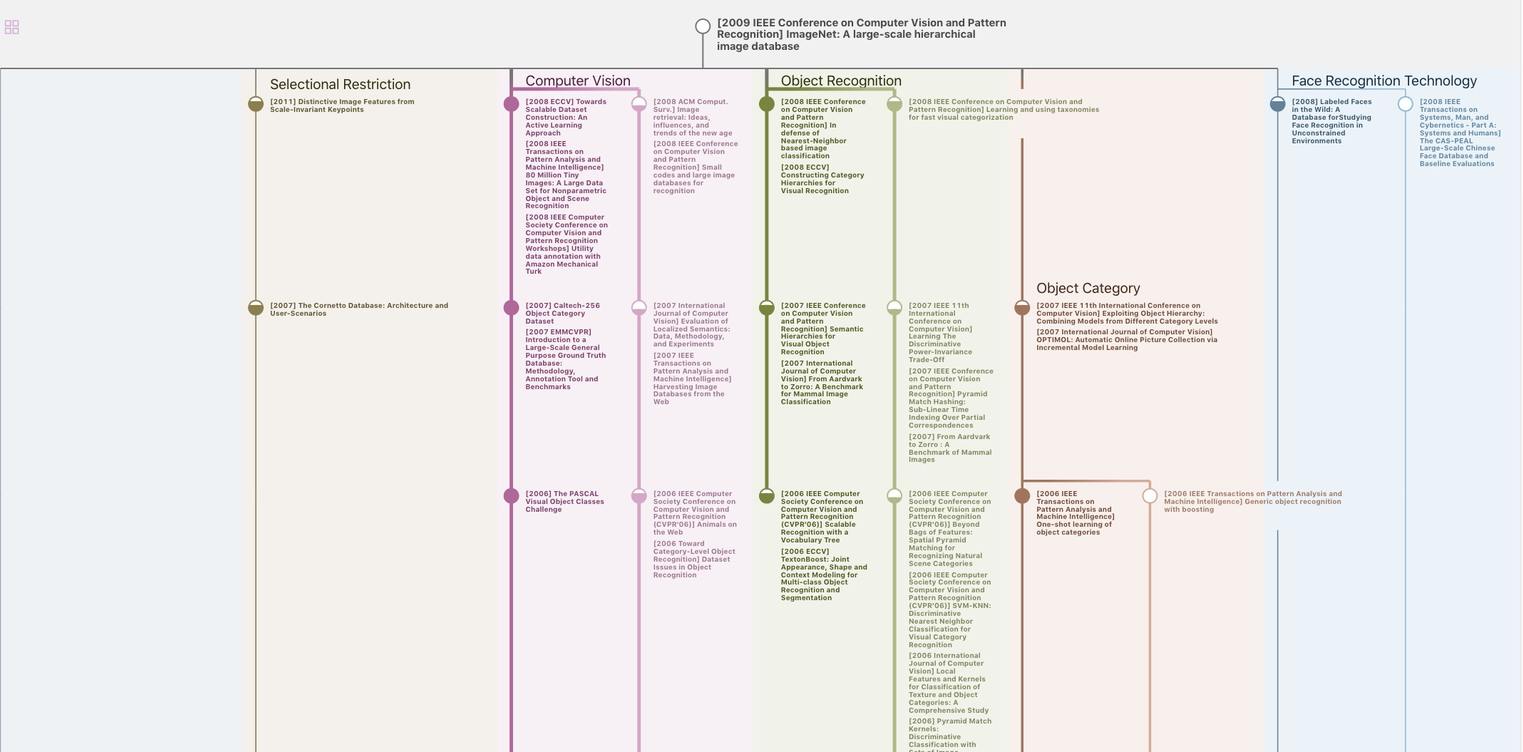
生成溯源树,研究论文发展脉络
Chat Paper
正在生成论文摘要