Comparative Analysis of Machine Learning Techniques for Hand Movement Prediction Using Electromyographic Signals
JITeCS (Journal of Information Technology and Computer Science)(2024)
摘要
The analysis of electromyography (EMG) signals plays a vital role in diverse applications such as medical diagnostics and prosthetic device control. This study focuses on evaluating machine learning methods for EMG signal analysis, specifically in predicting hand movements and controlling prosthetic hands. In contrast to many existing studies that solely employ a limited set of feature extraction methods, we employ a comprehensive comparison technique that encompasses nine machine learning techniques K-Nearest Neighbor (KNN) , State Vector Machine (SVM ) , Decision Tree, Random Forest, Linear Discriminant Analysis (LDA), XGBoost, Naïve Bayes, Gradient Boosting, and Quadratic Discriminant Analysis (QDA) and five combination of feature extraction methods (Mean Absolute Value (MAV), Root Mean Square (RMS), Waveform Length, Willison Amplitude, and Skewness). The experimental results demonstrate promising accuracy levels, with the best result method being KNN achieving 96.66% accuracy, SVM achieving 95.83% accuracy, and RF achieving 92.5% accuracy. These findings contribute to advancing the understanding of effective machine learning approaches for EMG signal analysis and provide valuable insights for guiding future research in this field. The study also compares the results with previous studies and showcases the effectiveness of the proposed approach.
更多查看译文
AI 理解论文
溯源树
样例
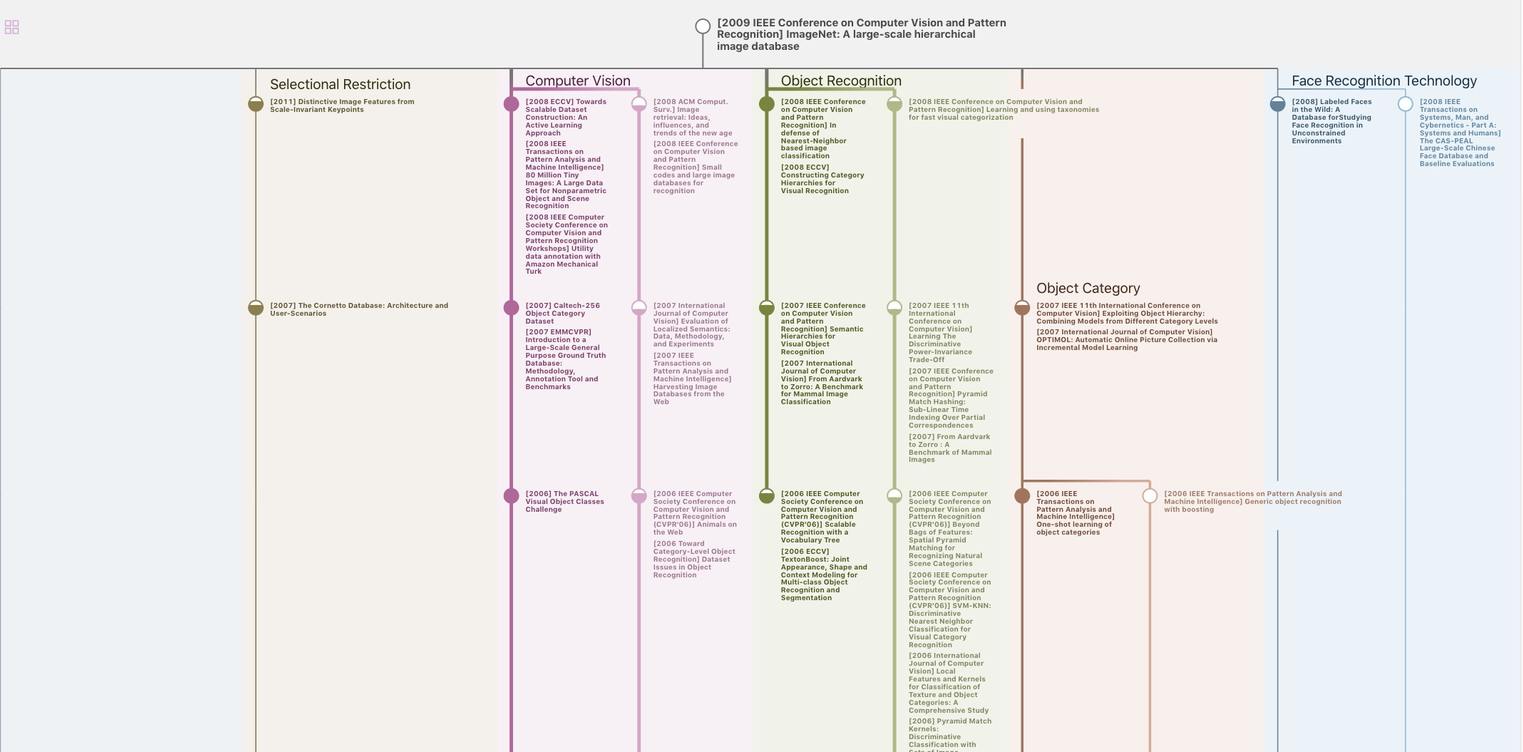
生成溯源树,研究论文发展脉络
Chat Paper
正在生成论文摘要