Fairness in Survival Outcome Prediction for Medical Treatments.
Annual Conference on Information Sciences and Systems(2024)
摘要
Cancer is a life-threatening disease and affects millions of people globally. Predicting the survival outcome of patients after receiving a cancer diagnosis is of great importance for personalized treatment planning. While predictive machine learning models have shown promising results in predicting survival outcomes, they may result in unfair treatments against certain groups, such as those based on age, gender, race and socioeconomic status. In this paper, we analyze and identify what kind of fairness criterion should be developed in cancer survival predictions. Considering the high stakes involved, we believe the basis for developing fairness in this field should be assisting to improve the model performance of diverse patients, rather than hurting the performance of certain patients in exchange for better performance of other patients. We suggest min-max fairness as an appropriate concept that seeks to minimize the group error of the worst-off group, instead of pursuing a strict equal error rate across various patient groups which would reduce the utility of the advantaged groups. We investigate the application of min-max fairness through active sampling in predicting the survival outcome of cancer patients using machine learning models, and evaluate the fairness-aware method on several publicly available cancer datasets. The experimental results demonstrate that the fairness-aware predictive method can help improve the model performance of the disadvantaged patient group without hurting the advantaged patient group, and thus increase the overall model performance for all patients.
更多查看译文
AI 理解论文
溯源树
样例
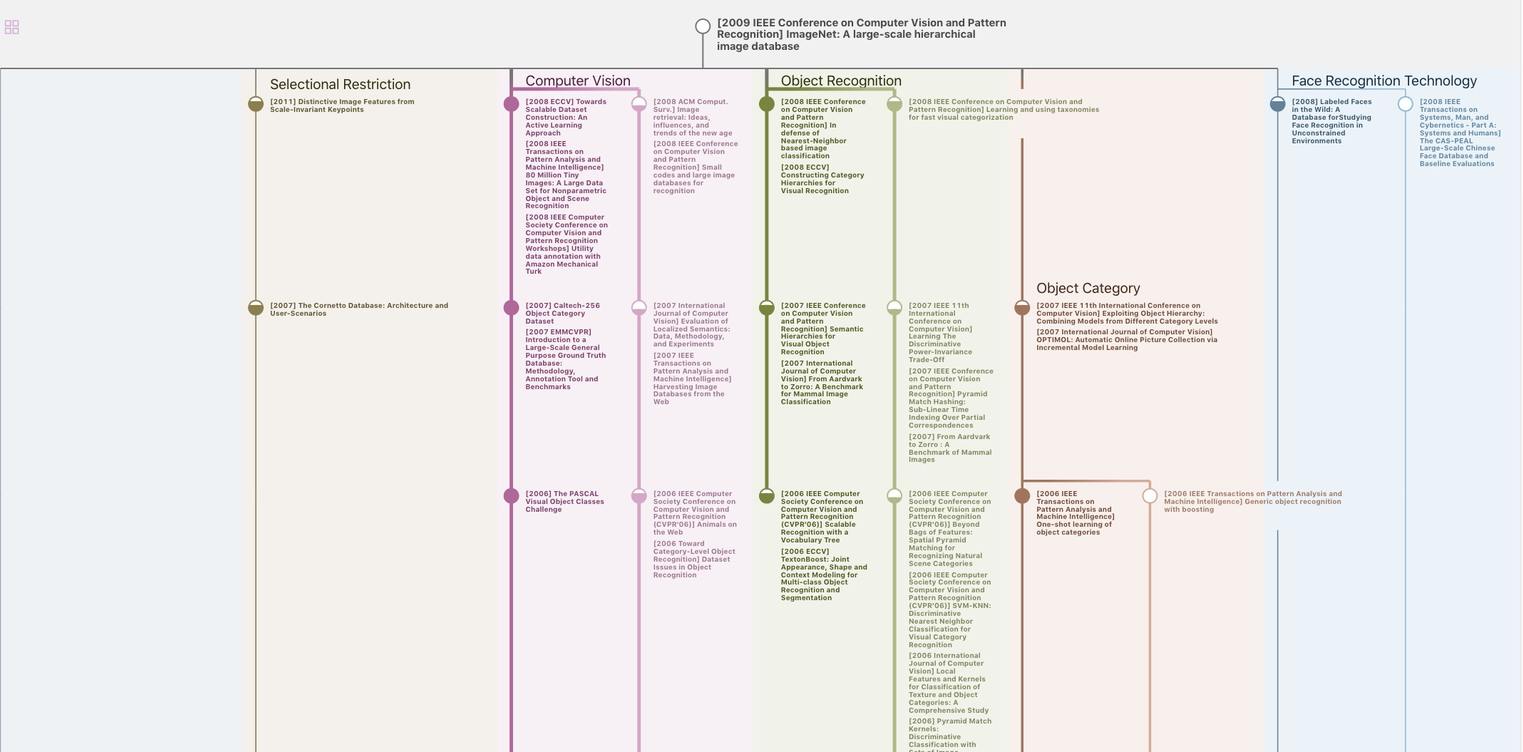
生成溯源树,研究论文发展脉络
Chat Paper
正在生成论文摘要