TLDBERT: Leveraging Further Pre-Trained Model for Issue Typed Links Detection.
Asia-Pacific Software Engineering Conference(2023)
摘要
Issue links are crucial for promoting software information flowing and development efficiency, but manually conducting typed links detection (TLD) is time-consuming and error-prone due to the numerous candidate issues. The general pre-trained NLP models, such as BERT, provide promising automated approaches for TLD task after fine-tuning. In addition, the further pre-training method, which is an intermediate pre-training process on the in-domain corpora, has been proven to further improve the performance of pre-trained models on many downstream tasks. In this paper, we apply the further pre-training method on TLD task and assess the improved performance. We further pre-train BERT by utilizing the in-domain corpora constructed by Jira issues dataset, and then finetune it on the links dataset to obtain the more applicable model for TLD task - TLDBERT. The experimental results indicate the statistically significant improvement in the performance of TLDBERT, with a range of 0.4%-6.6% for accuracy and 0.1%-9.7% for macro F1-score. Finally, based on correlation analysis, we conclude that the coverage and the ratio of issues to users are significantly correlated with the improvement, although the two directions are opposite.
更多查看译文
AI 理解论文
溯源树
样例
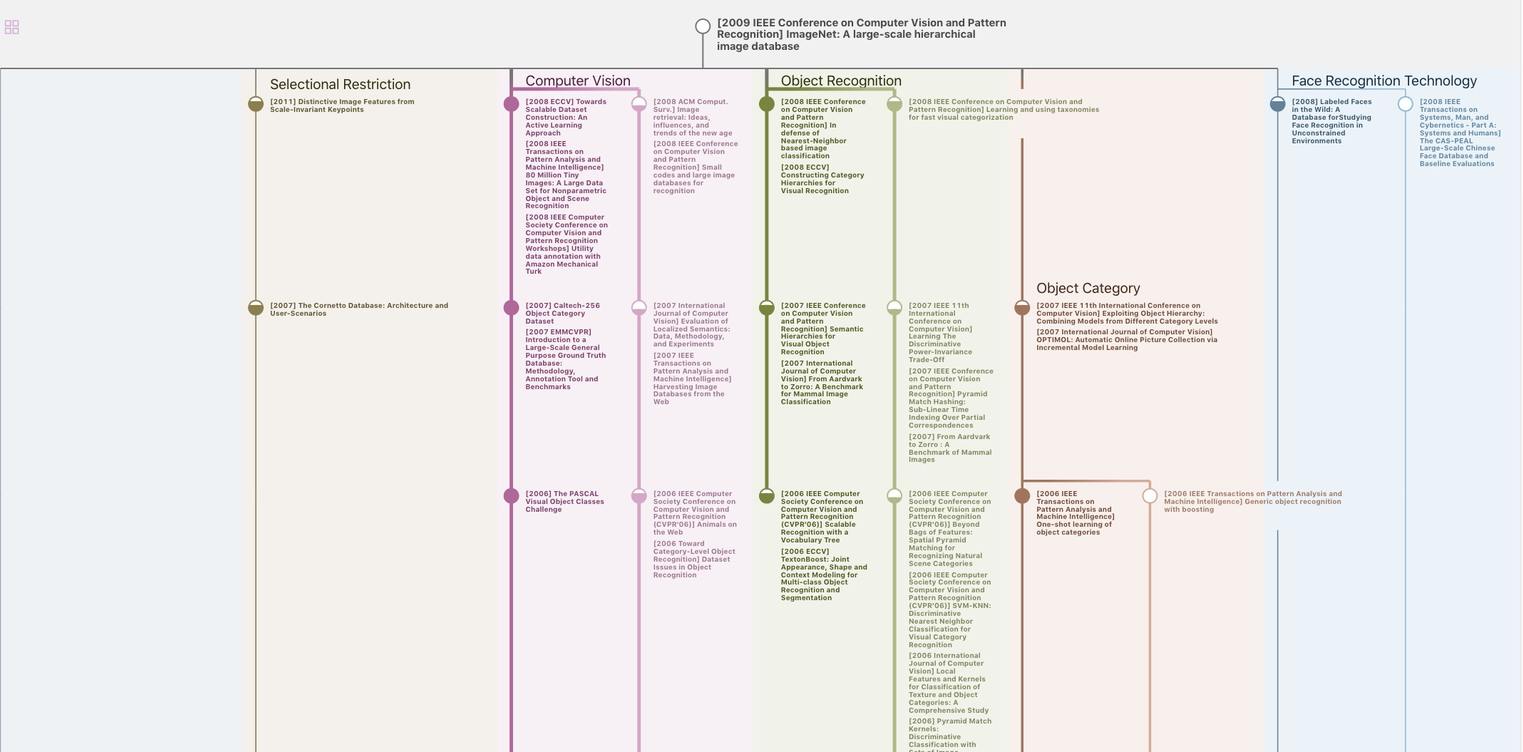
生成溯源树,研究论文发展脉络
Chat Paper
正在生成论文摘要