Quantitative Evaluation of Activation Maps for Weakly-Supervised Lung Nodule Segmentation
COMPUTER-AIDED DIAGNOSIS, MEDICAL IMAGING 2024(2024)
摘要
The manual assessment of chest radiographs by radiologists is a time-consuming and error-prone process that relies on the availability of trained professionals. Deep learning methods have the potential to alleviate the workload of radiologists in pathology detection and diagnosis. However, one major drawback of deep learning methods is their lack of explainable decision-making, which is crucial in computer-aided diagnosis. To address this issue, activation maps of the underlying convolutional neural networks (CNN) are frequently used to indicate the regions of focus for the network during predictions. However, often, an evaluation of these activation maps concerning the actual predicted pathology is missing. In this study, we quantitatively evaluate the usage of activation maps for segmenting pulmonary nodules in chest radiographs. We compare transformer-based, CNN-based, and hybrid architectures using different visualization methods. Our results show that although high performance can be achieved in the classification task across all models, the activation masks show little correlation with the actual position of the nodules.
更多查看译文
关键词
Nodule Detection,Chest Radiographs,Weakly Supervised Segmentation,Explainable AI,Deep Learning
AI 理解论文
溯源树
样例
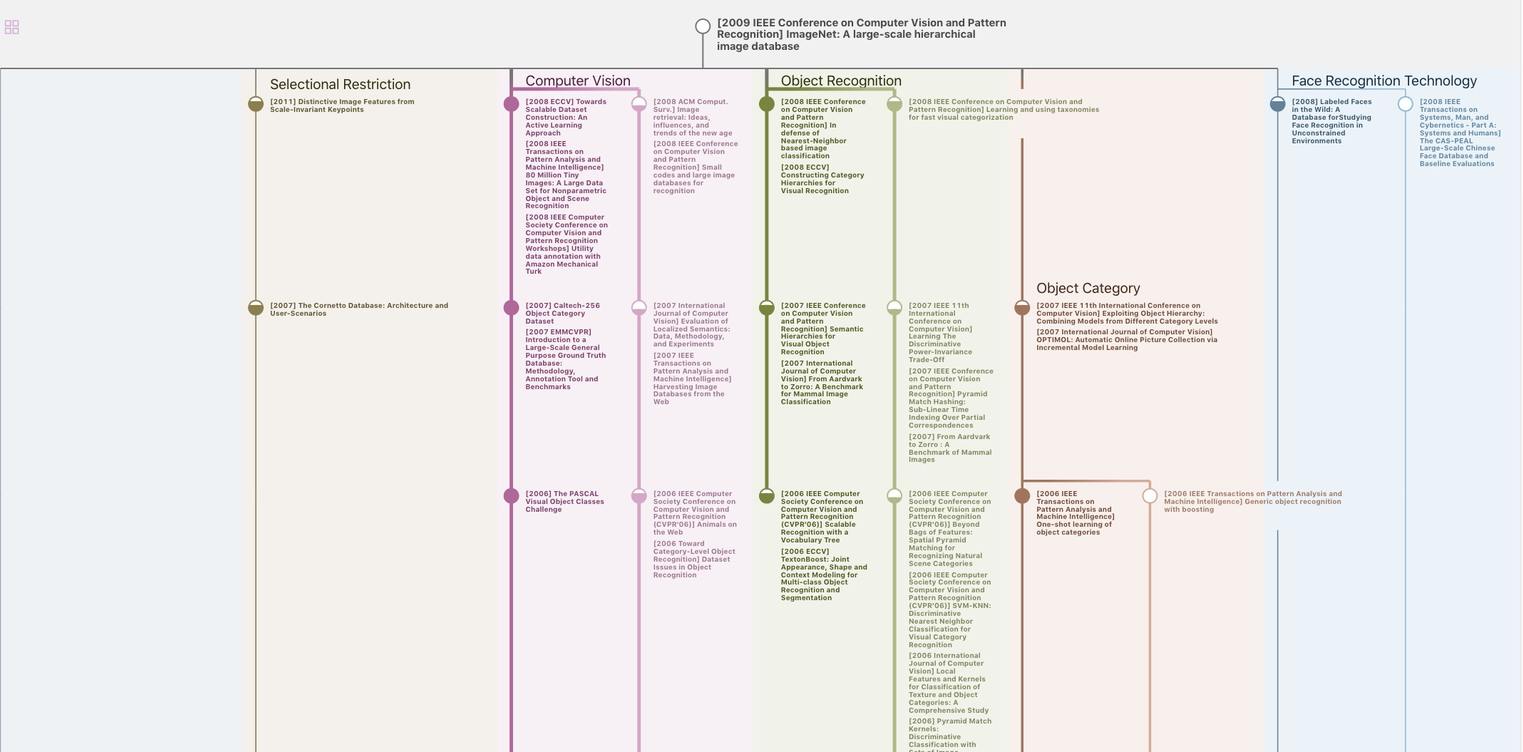
生成溯源树,研究论文发展脉络
Chat Paper
正在生成论文摘要