Hybrid Machine Learning Classification for Tipple Negative Breast Cancer
2023 11th International Japan-Africa Conference on Electronics, Communications, and Computations (JAC-ECC)(2023)
摘要
This research introduces an innovative approach to dissecting Triple-Negative Breast Cancer (TNBC) by integrating count-based RNA analysis and machine learning techniques. Through the strategic amalgamation of K-means clustering, Convolutional Neural Network (CNN), and Support Vector Machine (SVM), this study transcends conventional classification methods, revealing detailed molecular insights within the complex TNBC landscape. The methodology initiates with count-based RNA analysis for dimensionality reduction, refining molecular classifications through K-means clustering. The incorporation of CNN allows for nuanced feature extraction, capturing intricate genomic relationships, while SVM enhances subtype predictions with precise classification. The addition of Gene Ontology (GO) and pathway analyses enriches our understanding by unraveling the functional implications of identified gene clusters. This synergistic methodology not only advances our comprehension of TNBC subtypes but also establishes a groundwork for targeted therapeutic strategies. The fusion of count-based RNA analysis and machine learning enables a comprehensive and nuanced exploration of TNBC complexity, offering valuable insights for personalized oncology approaches in the era of precision medicine
更多查看译文
AI 理解论文
溯源树
样例
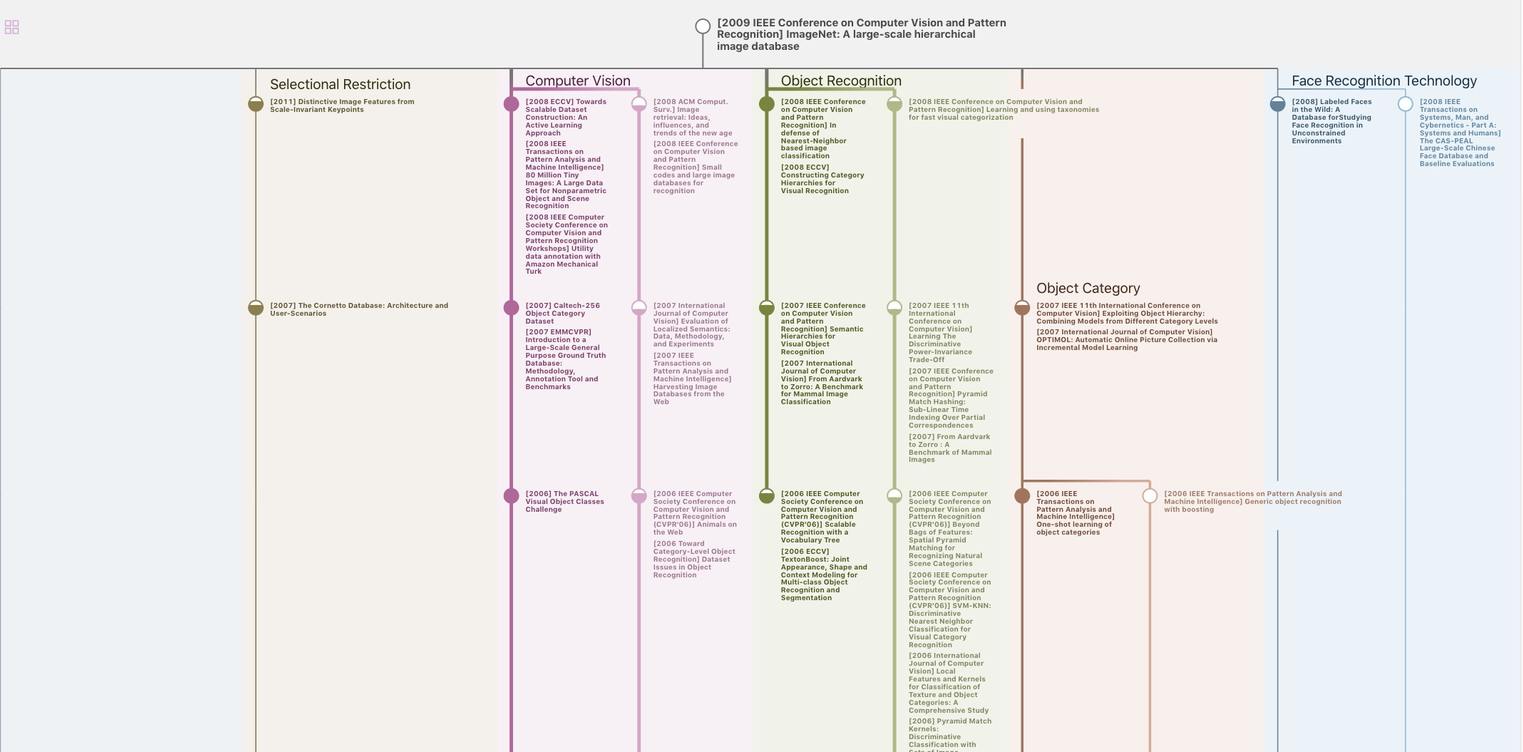
生成溯源树,研究论文发展脉络
Chat Paper
正在生成论文摘要