From Vision to Precision: The Dynamic Transformation of Object Detection in Autonomous Systems
2024 6th International Conference on Computing and Informatics (ICCI)(2024)
摘要
This paper explores the evolution of self-driving cars, from early radio-controlled prototypes to today's advanced autonomous vehicles, highlighting key milestones such as Houdina Radio Control's 1920s efforts and Carnegie Mellon University's Navlab project in the 1980s. Recent advancements driven by industry leaders like Google, Tesla, and Uber incorporate advanced technologies, including sensors, cameras, radar, and lidar, empowering self-driving cars for autonomous navigation. The literature review in Frontiers in Future Transportation focuses on performance metrics for automated driving systems, discussing methodologies for quantifying obstacle threats and enhancing human-like perception and response mechanisms, with a significant emphasis on deep learning methods such as Convolutional Neural Networks (CNNs) and Recurrent Neural Networks (RNNs). Object detection algorithms' dynamic evolution, influenced by CNNs, is explored, and the integration of hardware accelerators like GPUs, FPGAs, and specialized AI accelerators is highlighted for real-time responsiveness. Deep learning applications in self-driving cars, including obstacle detection, lane recognition, and traffic sign and light recognition, are outlined. Vision sensor-based obstacle detection using monocular and binocular approaches is discussed, emphasizing the importance of binocular vision technology. Lane recognition's significance for deviation warning, collision prevention, and path planning is highlighted, along with deep learning approaches such as John et al.'s lane detection algorithm. Traffic sign and light recognition are explored for safety and regulatory compliance, utilizing CNNs and Deep Neural Networks (DNNs). The discussion on sensors covers simultaneous localization and mapping (SLAM) using both passive (digital cameras) and active sensors (LIDAR, RADAR), emphasizing the integration of sensors and pre-existing maps to enhance the SLAM process and contribute to the overall perception of autonomous vehicles.
更多查看译文
AI 理解论文
溯源树
样例
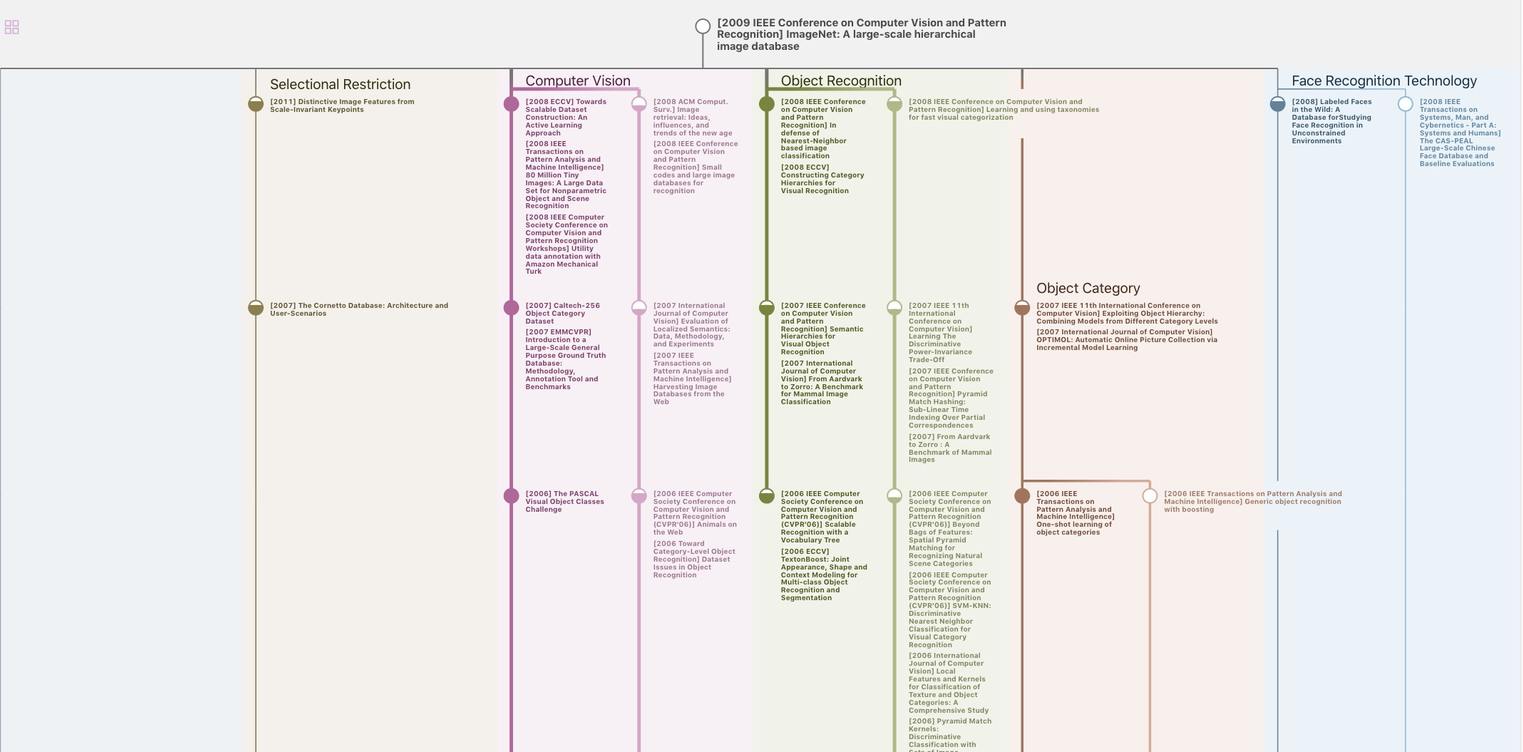
生成溯源树,研究论文发展脉络
Chat Paper
正在生成论文摘要