A feasibility study on the adoption of a generative denoising diffusion model for the synthesis of fundus photographs using a small dataset
Discover Applied Sciences(2024)
摘要
The generative diffusion model has been highlighted as a state-of-the-art artificial intelligence technique for image synthesis. Here, we show that a denoising diffusion probabilistic model (DDPM) can be used for a domain-specific task generating fundus photographs based on a limited training dataset in an unconditional manner. We trained the DDPM based on U-Net backbone architecture, which is the most popular form of the generative diffusion model. After training, serial multiple denoising U-Nets can generate FPs using random noise seeds. A thousand healthy retinal images were used to train the diffusion model. The input image size was set to a pixel resolution of 128 × 128. The trained DDPM successfully generated synthetic fundus photographs with a resolution of 128 × 128 pixels using our small dataset. We failed to train the DDPM for 256-by-256-pixel images due to the limited computation capacity using a personal cloud platform. In a comparative analysis, the progressive growing generative adversarial network (PGGAN) model synthesized more sharpened images than the DDPM in the retinal vessels and optic discs. The PGGAN (Frechet inception distance [FID] score: 41.761) achieved a better FID score than the DDPM (FID score: 65.605). We used a domain-specific generative diffusion model to synthesize fundus photographs based on a relatively small dataset. Because the DDPM has disadvantages with a small dataset, including difficulty in training and low image quality compared with generative adversarial networks such as PGGAN, further studies are needed to improve diffusion models for domain-specific medical tasks with small numbers of samples.
更多查看译文
关键词
Generative model,Diffusion model,Fundus photography,Denoising diffusion probabilistic model
AI 理解论文
溯源树
样例
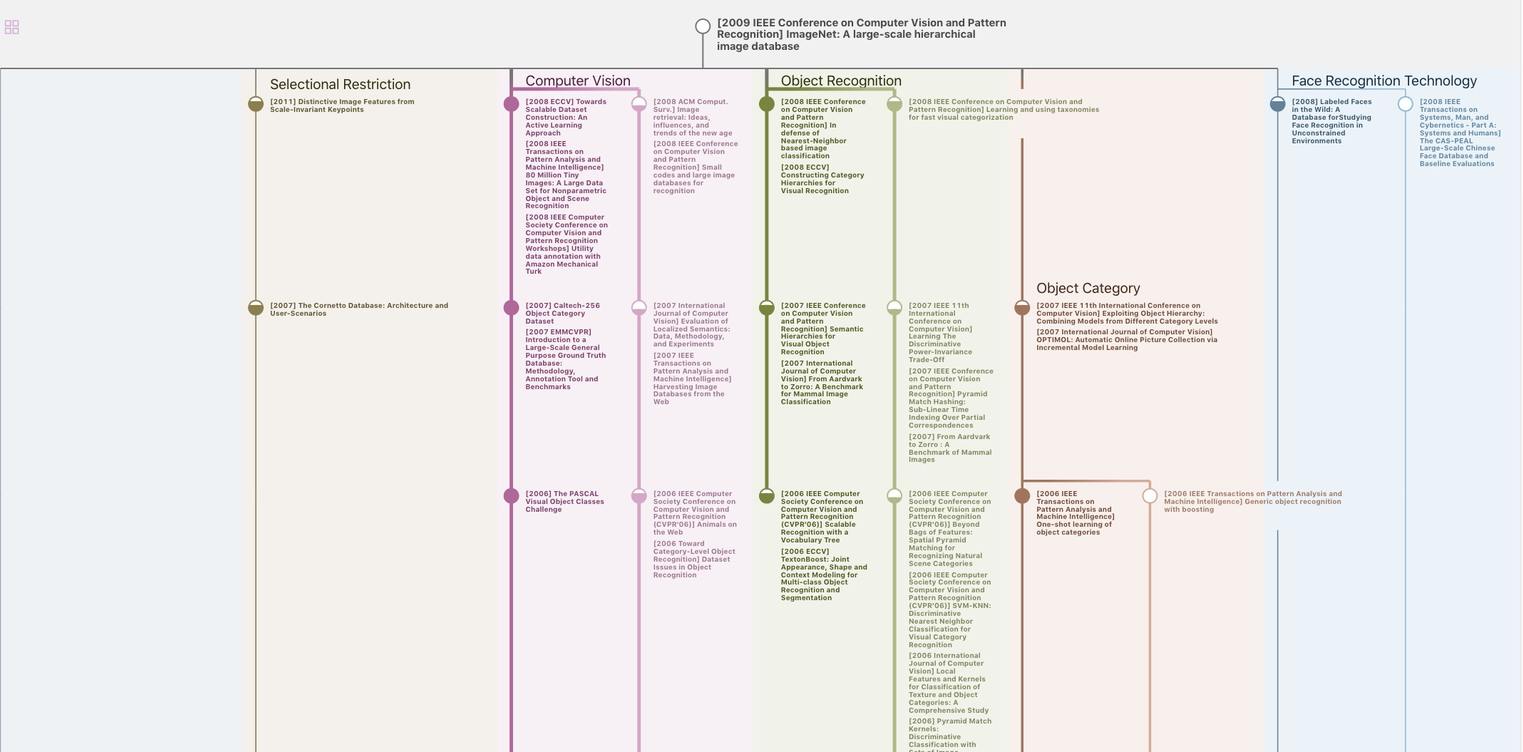
生成溯源树,研究论文发展脉络
Chat Paper
正在生成论文摘要