Neural-network-enhanced Line-of-sight Method for 3D Particle Cloud Reconstruction in Particle Tracking Velocimetry
Experiments in fluids(2024)
摘要
The algorithm of three-dimensional (3D) particle cloud reconstruction is a building block for 3D particle tracking velocimetry (3D-PTV). In the present study, a new 3D particle cloud reconstruction algorithm, i.e., neural-network-enhanced line of sight (NN-LOS), is proposed to update the traditional method based on Line of Sight (LOS) algorithm. The essence of NN-LOS is to use a matching neural network (Matching-NN) to evaluate whether or not one set of candidate matching being recorded by different cameras with various viewing perspectives is valid. Such a Matching-NN is in situ trained from virtual datasets that are synthetically generated by taking into account both the spatial calibration information and the actual seeding density in one particular experiment setup. This makes NN-LOS be self-adaptive to the measurement configuration, and thus avoids the problem of properly selecting a filtering threshold for the reprojection error in LOS. Both a series of synthetic tests and one surface morphology measurement are taken to prove that comparing to LOS, NN-LOS provides a significant improvement of the matching accuracy at high seeding density. A 3D-PTV measurement of a vortex ring in a synthetic jet is experimentally performed to demonstrate the advantage of NN-LOS. Comparing to tomographic particle image velocimetry, NNLOS-PTV enhances the spatial resolution of the velocity-field measurement and reduces the uncertainty of instantaneous velocity. The performance improvement is further empirically explained by a semiempirical test.
更多查看译文
AI 理解论文
溯源树
样例
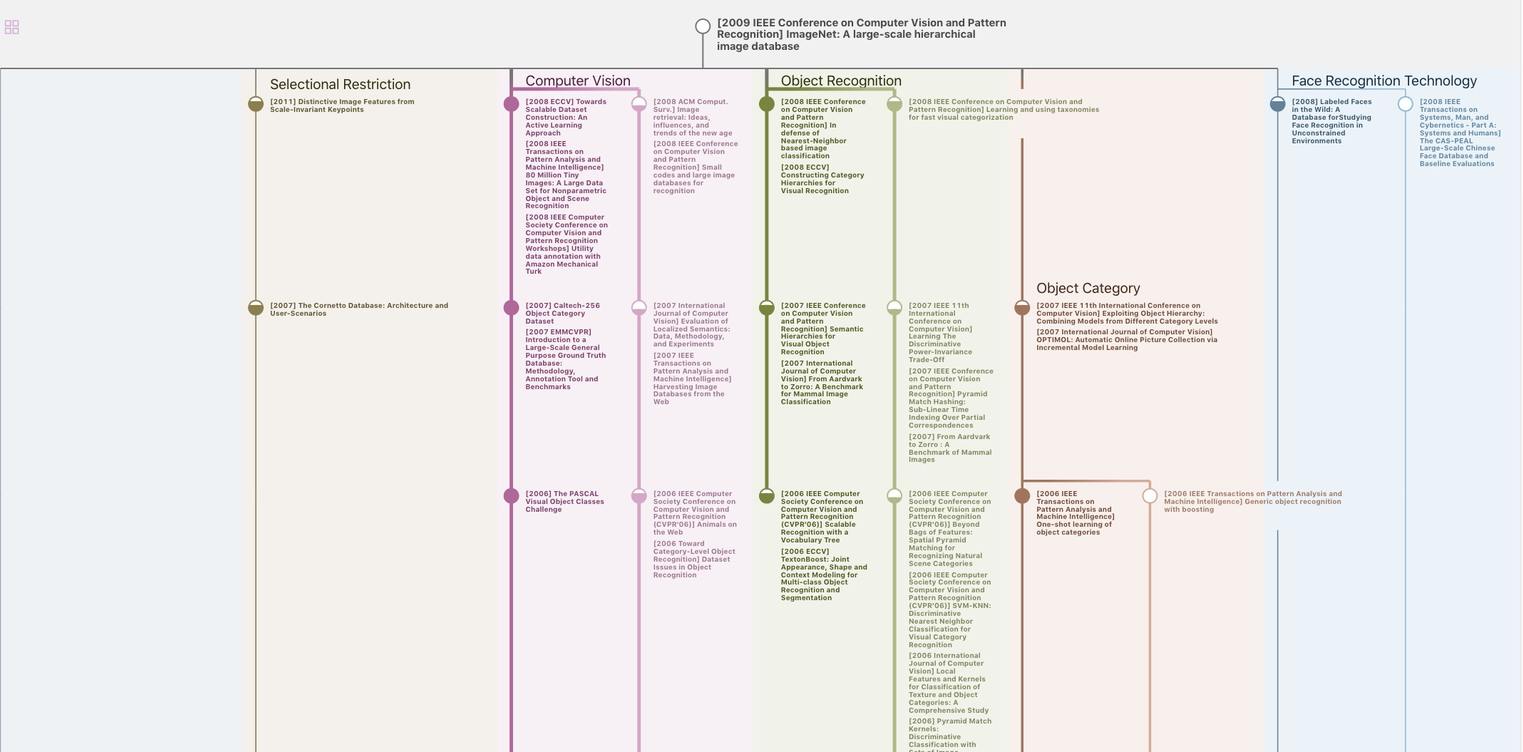
生成溯源树,研究论文发展脉络
Chat Paper
正在生成论文摘要