Effective MLP and CNN based ensemble learning for speech emotion recognition
Multimedia Tools and Applications(2024)
摘要
Speech emotion recognition (SER) is one of the most important and active areas of. research in speech processing. Numerous approaches have been proposed to address various limitations in this field, but the sheer diversity of speech emotions, as well as their complexity, continue to make SER a tough nut to crack. This paper attempts to conduct a thorough investigation into speech emotion recognition in order to determine the most appropriate feature set and model for SER. A multi-layer perceptron (MLP) and convolutional neural network (CNN) based ensemble model for SER is proposed, which is a simple yet very powerful model for SER that can greatly improve classification accuracy. The model’s performance is evaluated based on four benchmark datasets, namely RAVDESS (Ryerson Audio-Visual Database of Emotional Speech and Song), EmoDB (Emotional Dat0abase), SAVEE (Surrey Audio-Visual Expressed Emotion), and TESS (Toronto Emotional Speech Set). The proposed model dominates over several baseline methods (decision tree (DT), random forest (RF), support vector machine (SVM), k-nearest neighbour (KNN), and the base learners, i.e., MLP and CNN) in terms of various performance metrics for all the datasets. Furthermore, the proposed model outperforms all previous works for RAVDESS (Acc=73.1
更多查看译文
关键词
Speech emotion recognition,Deep learning,Classification,Convolutional neural network.
AI 理解论文
溯源树
样例
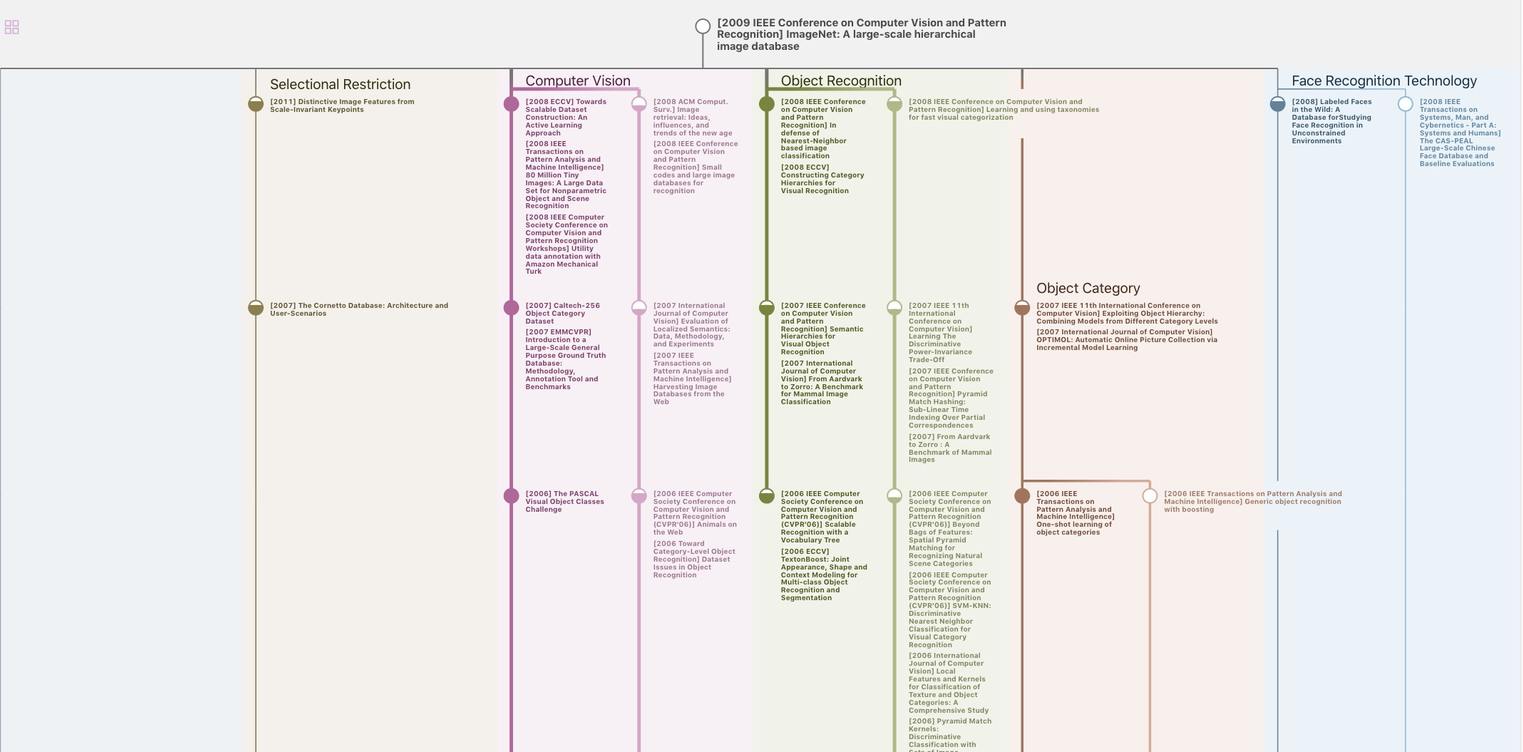
生成溯源树,研究论文发展脉络
Chat Paper
正在生成论文摘要