Machine learning-based vorticity evolution and superresolution of homogeneous isotropic turbulence using wavelet projection
arxiv(2024)
摘要
A wavelet-based machine learning method is proposed for predicting the time
evolution of homogeneous isotropic turbulence where vortex tubes are preserved.
Three-dimensional convolutional neural networks and long short-term memory are
trained with a time series of direct numerical simulation (DNS) data of
homogeneous isotropic turbulence at the Taylor microscale Reynolds number 92.
The predicted results are assessed by using flow visualization of vorticity and
statistics, e.g., probability density functions of vorticity and enstrophy
spectra. It is found that the predicted results are in good agreement with DNS
results. The small-scale flow topology considering the second and third
invariant of the velocity gradient tensor likewise shows an approximate match.
Furthermore, we apply the pre-trained neural networks to coarse-grained
vorticity data using superresolution. It is shown that the superresolved flow
field well agrees with the reference DNS field and thus small-scale information
and vortex tubes are well regenerated.
更多查看译文
AI 理解论文
溯源树
样例
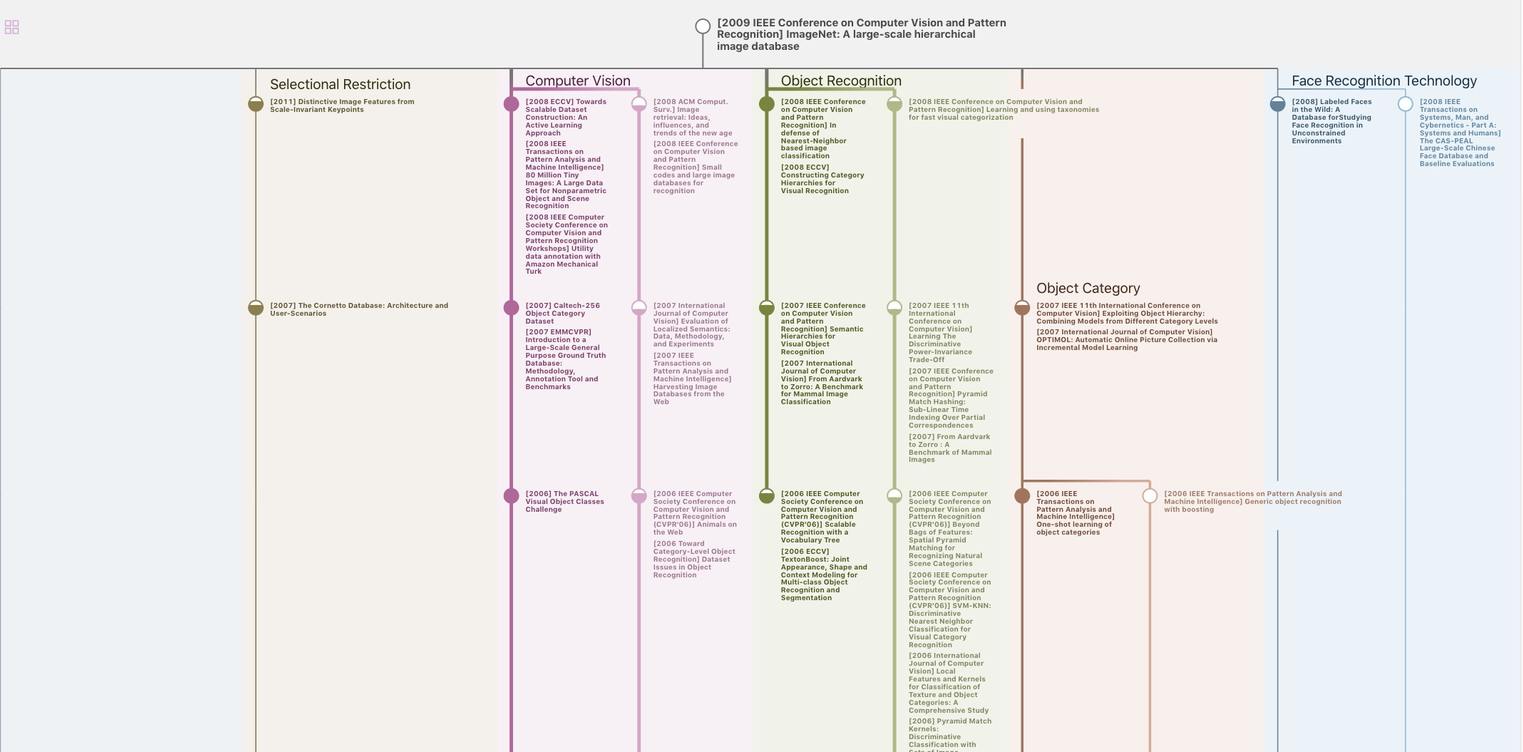
生成溯源树,研究论文发展脉络
Chat Paper
正在生成论文摘要