Large Language Models for Expansion of Spoken Language Understanding Systems to New Languages
arxiv(2024)
摘要
Spoken Language Understanding (SLU) models are a core component of voice
assistants (VA), such as Alexa, Bixby, and Google Assistant. In this paper, we
introduce a pipeline designed to extend SLU systems to new languages, utilizing
Large Language Models (LLMs) that we fine-tune for machine translation of
slot-annotated SLU training data. Our approach improved on the MultiATIS++
benchmark, a primary multi-language SLU dataset, in the cloud scenario using an
mBERT model. Specifically, we saw an improvement in the Overall Accuracy
metric: from 53
Fine and Coarse-grained Multi-Task Learning Framework (FC-MTLF). In the
on-device scenario (tiny and not pretrained SLU), our method improved the
Overall Accuracy from 5.31
Contrastive Learning Framework (GL-CLeF) method. Contrary to both FC-MTLF and
GL-CLeF, our LLM-based machine translation does not require changes in the
production architecture of SLU. Additionally, our pipeline is slot-type
independent: it does not require any slot definitions or examples.
更多查看译文
AI 理解论文
溯源树
样例
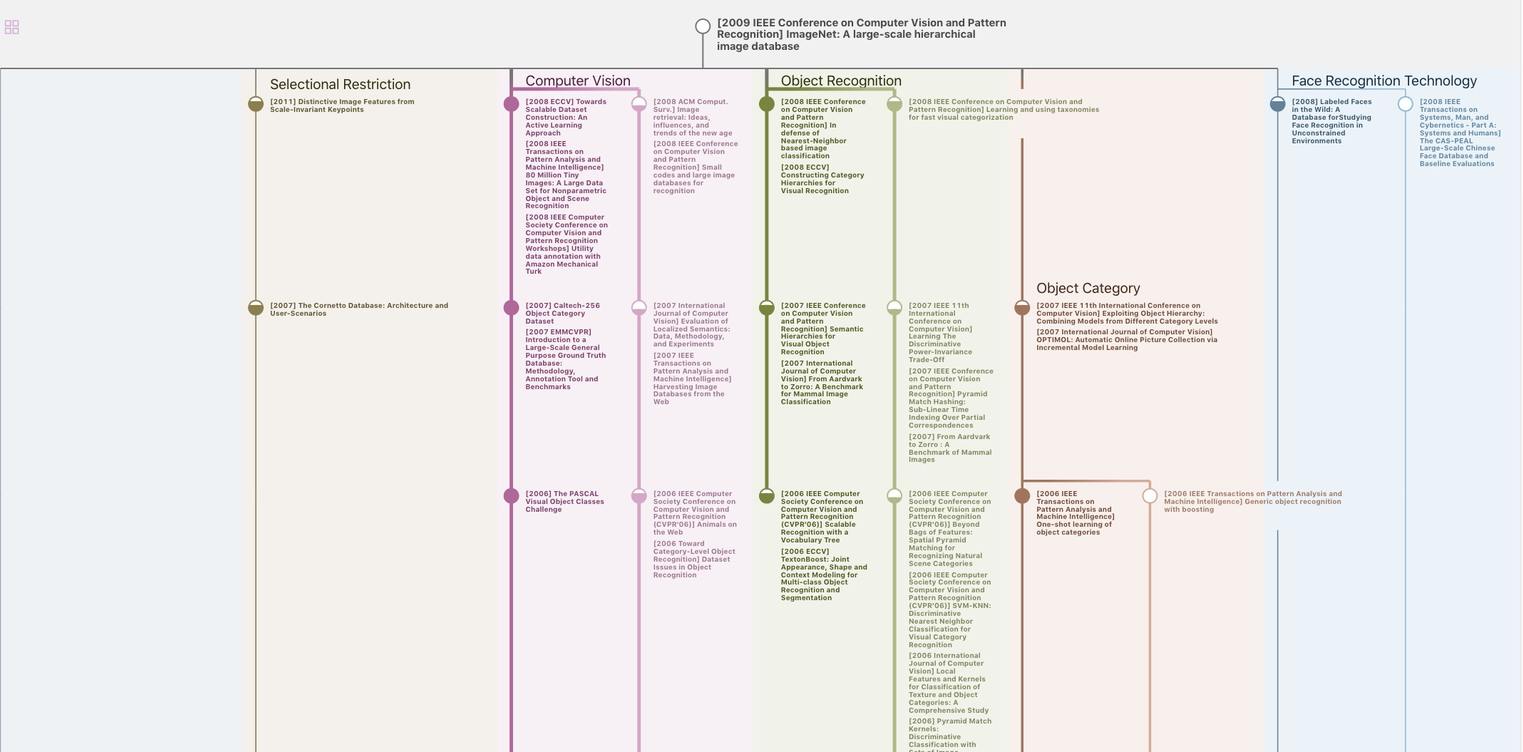
生成溯源树,研究论文发展脉络
Chat Paper
正在生成论文摘要