Investigating the Relation Between Problem Hardness and QUBO Properties
Lecture Notes in Computer Science Advances in Intelligent Data Analysis XXII(2024)
摘要
Combinatorial optimization problems, integral to various scientific and
industrial applications, often vary significantly in their complexity and
computational difficulty. Transforming such problems into Quadratic
Unconstrained Binary Optimization (QUBO) has regained considerable research
attention in recent decades due to the central role of QUBO in Quantum
Annealing. This work aims to shed some light on the relationship between the
problems' properties. In particular, we examine how the spectral gap of the
QUBO formulation correlates with the original problem, since it has an impact
on how efficiently it can be solved on quantum computers. We analyze two
well-known problems from Machine Learning, namely Clustering and Support Vector
Machine (SVM) training, regarding the spectral gaps of their respective QUBO
counterparts. An empirical evaluation provides interesting insights, showing
that the spectral gap of Clustering QUBO instances positively correlates with
data separability, while for SVM QUBO the opposite is true.
更多查看译文
AI 理解论文
溯源树
样例
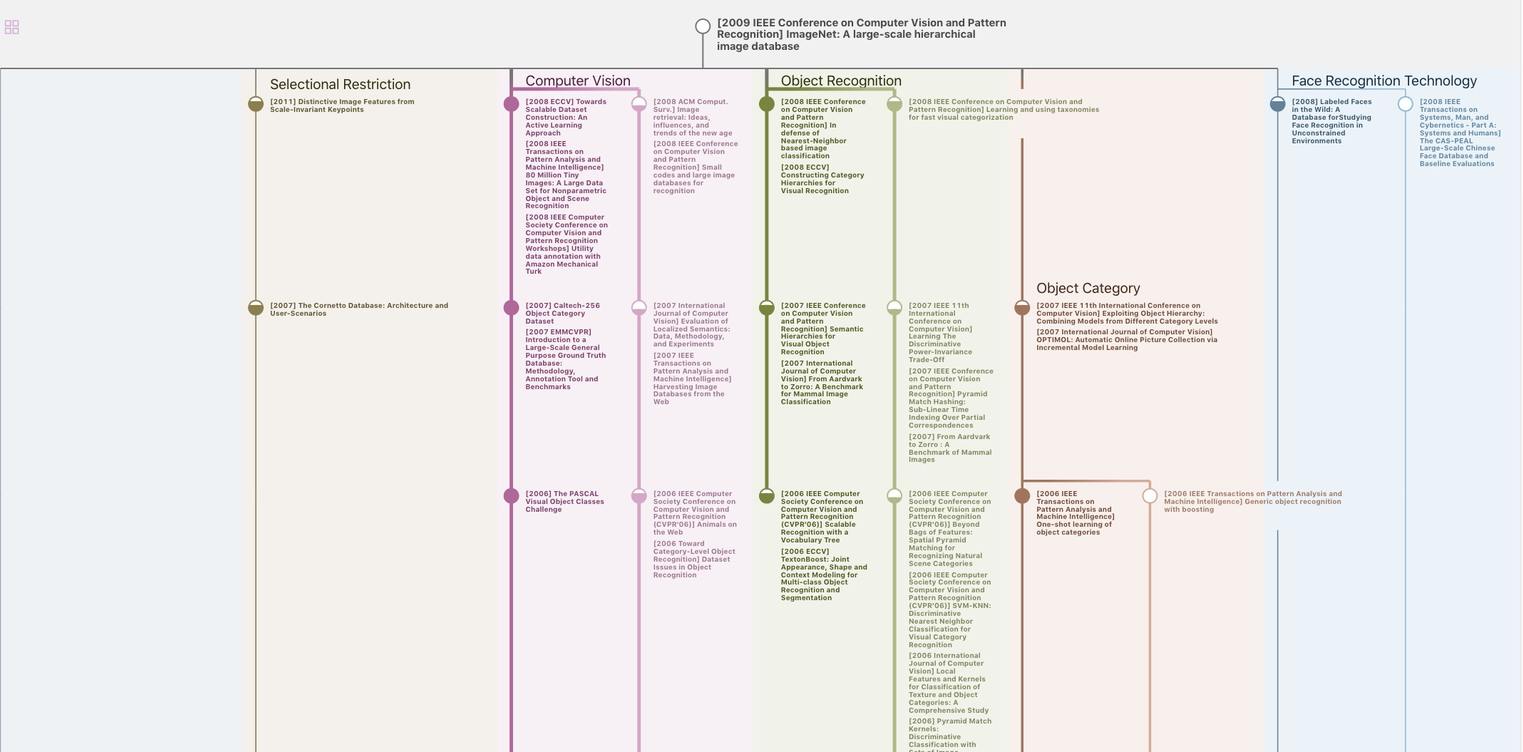
生成溯源树,研究论文发展脉络
Chat Paper
正在生成论文摘要